AI in the SOC: Is It the Savior or Just Another Gimmick?AI in the SOC: Is It the Savior or Just Another Gimmick?
AI can enhance security operations, especially by aiding less experienced analysts. However, successful AI integration requires attention to output quality.
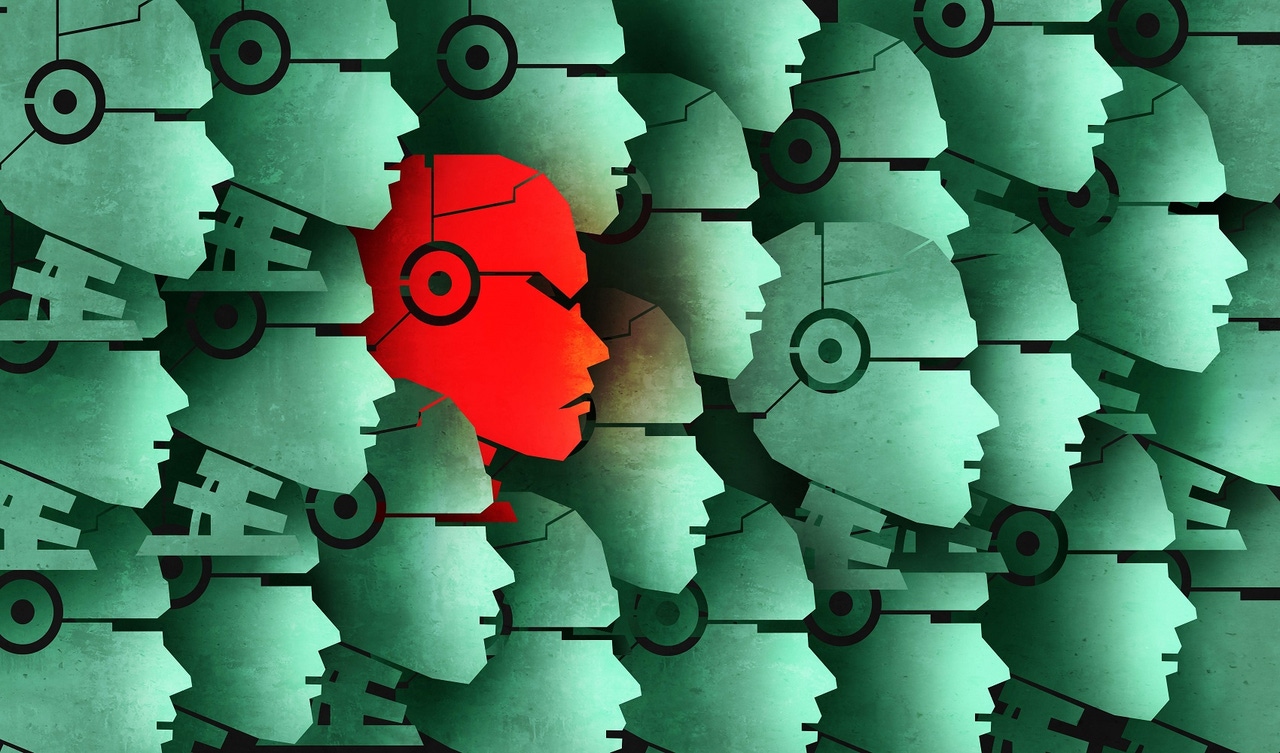
We’ve been talking about the multiple ways AI can support security operations activities. There are many new products and features being released based on LLM-based chatbots, with a strong focus on helping analysts do their jobs. Among the most typical use cases are requests for additional information and context during investigations and querying data using natural language as opposed to query languages. These features could theoretically help less skilled analysts do things that would normally require more experienced resources. AI’s capacity for processing natural language queries can streamline interactions with intricate security systems, thereby facilitating a more user-friendly experience for analysts.
The infusion of AI into security operations has the promise of transformative change. By automating routine tasks, AI can free up analysts to tackle more sophisticated challenges, effectively enhancing the overall productivity of the security operation center (SOC). For those analysts who possess less experience, AI can provide guidance, simplifying complex tasks that would typically demand a more seasoned hand.
AI's rapid response capabilities can prove invaluable in addressing emerging threats, offering a speed of detection and mitigation that often surpasses human capability. Additionally, the automation of certain security tasks by AI could lead to operational cost reductions, presenting an economic benefit to organizations by potentially diminishing the need for a large staff of highly specialized security professionals.
Despite these potential advantages, the introduction of AI into security operations is not without its challenges. An over-reliance on AI could lead to the atrophy of an analyst's own skills, creating a dependency that diminishes their ability to operate independently. The complexity of implementing AI systems can also introduce additional overhead, potentially leading to inefficiencies if not properly managed. The security of AI systems themselves is another critical consideration; if such systems are compromised, they could be manipulated to misdirect analysts or to automate the propagation of an attack within an organization.
Ultimately, how successful AI will be in the SOC will be defined by its output quality. That’s because when leaving a task for a less skilled analyst to perform, aided by an AI resource, they may not have the right skills to judge how well the AI is performing the task. What does that mean?
Let’s start by looking at how an analyst would perform an investigation via common queries on a search engine. They need to be proficient in that query language in order to do that. They will think about the data they need to see and then proceed to right the query to obtain it. Done.
Now, let’s imagine we have an analyst who does not have the same proficiency in the query language doing the same job and who also uses AI to obtain the query required to get the data. The analyst asks the AI what they want, and the AI will generate the query, which is executed to provide the expected data. In this scenario, how can we be sure the query generated by the AI will indeed provide the expected result? What if it's leaving out some condition in a way that will end up causing false negatives? This scenario is concerning because the analyst here does not have the knowledge required to review the generated query and confirm if it's indeed doing what it should. Moreover, if the AI’s decision-making processes are not transparent, this ‘black box’ effect can erode trust and make it difficult even for experienced analysts to follow the logic behind AI-driven actions.
That’s why a high standard for the AI output quality is crucial to enable the scenario above. Without it, we may have a tool that saves some time for the skilled analyst, but it’s not reliable enough to enable less skilled analysts to perform the same job.The same reasoning applies to creating detection logic, such as detection rules. Can we trust the AI enough so we can have it create detection content and put it in production without the review of a skilled analyst? What about response actions? How reliable must the AI be so we will let it run response actions without a highly skilled human in the loop?
AI certainly holds the potential to revolutionize security operations. However, its successful integration demands careful consideration, consistent oversight, and a balanced approach that leverages the strengths of both AI systems and human analysts. By emphasizing quality, organizations can utilize AI to boost the efficiency of security operations without jeopardizing security or efficacy.
About the Author
You May Also Like