11 Irritating Data Quality Issues11 Irritating Data Quality Issues
Organizations have been trying to improve data quality for more than 30 years, but it’s still an issue. In fact, it’s more important than ever. Here’s a look at common data quality challenges that can drive you mad.
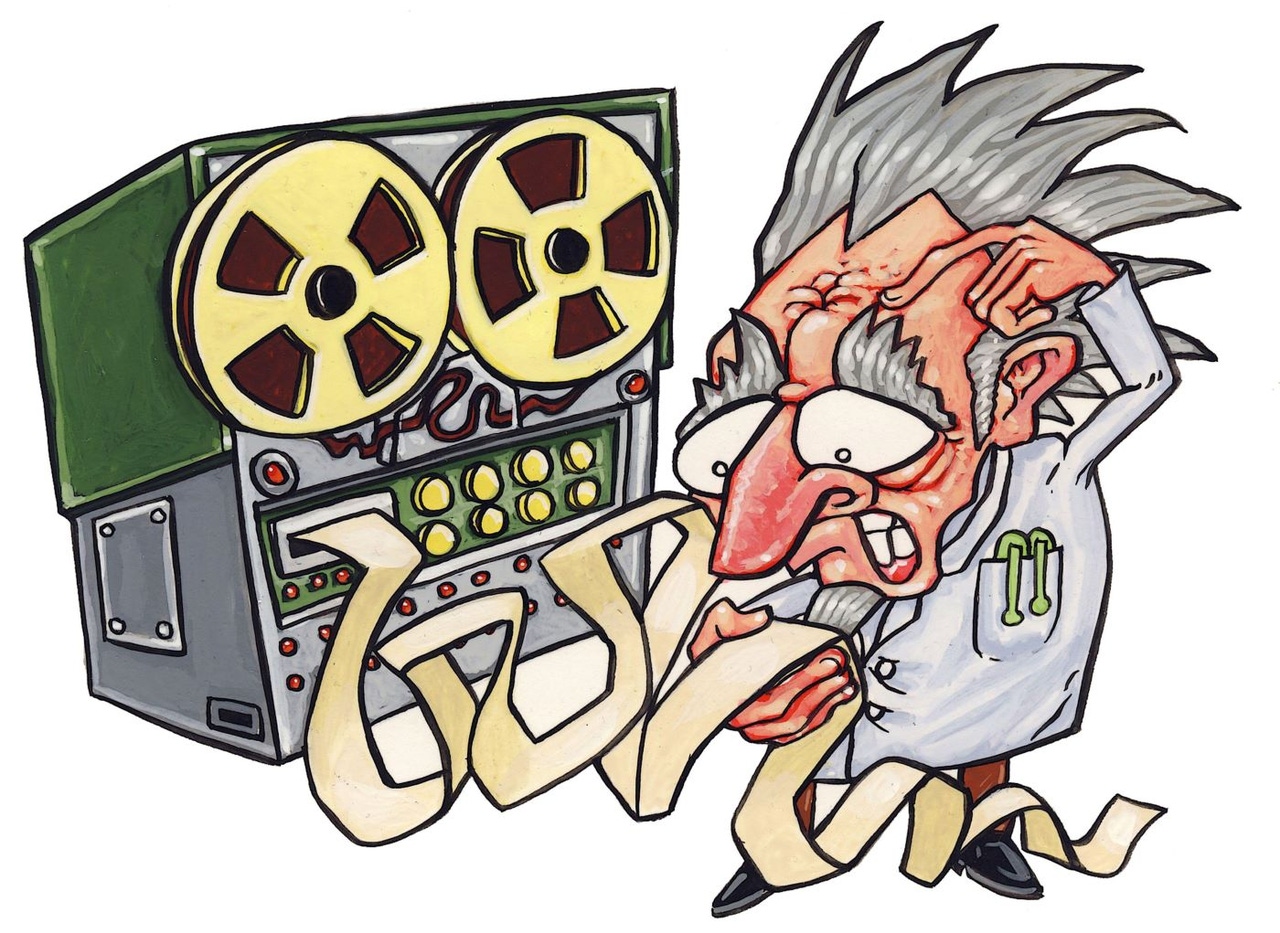
Data quality isn’t a new concept, though its importance has continued to grow with big data, analytics and AI. Without good data quality, analytics and AI are not reliable.
“The traditional issues around data quality are still the kind of issues we see today,” says Felix Van de Maele, CEO of data intelligence company Collibra. “If you think about completeness, accuracy, consistency, validity, uniqueness, and integrity, they’re very much the same data quality dimensions that companies struggle with to this day.”
So, why are companies still struggling with data quality? Because it’s still not getting the attention it deserves. For one thing, it’s not as sexy as analytics or AI.
“Data quality has never been more critical, particularly in the realm of artificial intelligence and analytics,” says Laura McElhinney, chief data officer at product-focused consultancy MadTech, in an email interview. “[T]he quality of the data directly impacts the accuracy and efficacy of AI outputs, whether we are discussing generative models or traditional machine learning systems. Data quality [also] forms the bedrock of effective analytics, reporting, and business decision-making. Without it, analytical insights are compromised, potentially leading to misguided strategies and decisions based on erroneous information. Therefore, ensuring high data quality is not just a technical requirement but a strategic imperative.”
The following are some of the most common data quality challenges that persist in enterprises.
1. Unstructured data
There’s a lot of talk about data quality as it relates to unstructured data, because there’s so much of it and organizations want to use it for AI. There are concerns about the quality of that data, its currency, redundancy, and the fact that people are cutting and pasting it from one system to another. Meanwhile, personally identifiable information (PII) and sensitive company data may be in places it shouldn’t be according to Jack Berkowitz, chief data officer at data intelligence platform provider Securiti AI. “[O]ne of the things about the data lakes was, well, we’ll just dump it in there. We’ll figure it out later,” says Berkowitz. “Here, you need to you need to have those business cases or those use cases decently defined that you’re going to try to do. Especially seek out and start incrementally getting your unstructured data organized. There's just too much of it to just say, well, we’re going to do everything. So, prioritizing some of these use cases, and just attacking it that way.”
2. Data entry
Humans are the root cause of data quality issues, and there are few better examples than in the healthcare industry in which information recorded on paper is manually entered into systems.
“Doctors’ offices, the physician, the nurses, the billing folks are taking your insurance care and typing things in [when] submitting bills,” says Ryan Leurck, co-founder, chief analytics officer at healthcare technology and data analytics company Kythera Labs. “The data quality in those electronic data systems is focused on the aspects that are the most important for ensuring that a payment happens, for example. They’re not going to mess up the dollars and cents, but there are 80 other fields. You might take for granted that a lot of the data on a claim is accurate, when it might be that no one ever looked at it.”
3. Readily available data
There is more data available today than ever, within organizations, on the internet, and elsewhere. Collecting that data is also easier than ever without proper labeling, cleaning, or adding context. Training AI models on such data could lead to erroneous results.
“Data without knowledge is like ingredients without cooking instruments or appliances. Sure, you could still mix all the ingredients together, but you’ll likely have a very unpleasant meal,” says Huda Nassar, senior computer scientist at AI coprocessor for cloud platforms and language models provider RelationalAI, in an email interview. “I believe the best way for organizations to produce good quality results is to introduce a knowledge layer that can help build the connections between their data. Additionally, knowledge that includes constraints on the data as part of a data cleaning process can be extra helpful.”
4. Failing to prioritize data quality
Organizations need to put data quality first and AI second. Without dignifying this sequence, leaders fall into fear of missing out (FOMO) in attempts to grasp AI-driven cures to either competitive or budget pressures, and they jump straight into AI adoption before conducting any sort of honest self-assessment as to the health and readiness of their data estate, according to Ricardo Madan, senior vice president at global technology, business and talent solutions provider TEKsystems.
“This phenomenon is not unlike the cloud migration craze of about seven years ago, when we saw many organizations jumping straight to cloud-native services, after hasty lifts-and-shifts, all prior to assessing or refactoring any of the target workloads. This sequential dysfunction results in poor downstream app performance since architectural flaws in the legacy on-prem state are repeated in the cloud,” says Madan in an email interview. “Fast forward to today, AI is a great ‘truth serum’ informing us of the quality, maturity, and stability of a given organization’s existing data estate -- but instead of facing unflattering truths, invest in holistic AI data readiness first, before AI tools."
5. Failing to label data
Many of the issues enterprises have with AI stem from a lack of organizing, sorting, and explaining of raw data that enable effective AI model training. This is also known as data labeling.
“Most often, a lack of effectively labeled data is the biggest challenge. Often, teams don’t know what they don’t know, which leads some founders to make alarming statements like AI could ‘most likely lead to the end of the world. If you don't know what the input is, you can’t be sure of what the output will be,” says Max Li, CEO of decentralized physical infrastructure networks startup Oort and an adjunct associate professor in the department of electrical engineering at Columbia University. “Organizations trying to tackle this internally don’t often fully grasp the magnitude of the work they are trying to accomplish and fail to realize how costly and labor-intensive this process is. It leads them to a place where transparency is lacking, and outcomes are puzzling.”
6. Having poor processes in place
According to recent research by HFS and Syniti, 85% of survey respondents realize that data is the cornerstone of business success, but only a third are satisfied with their enterprise data quality. In fact, they say 40% of their organizational data is unusable.
“Everyone involved needs to understand that all data problems are created by process problems and that those create further process problems,” says Kevin Campbell, CEO of enterprise data management company Syniti in an email interview. “It’s a Catch-22: You have a bad process, that means you get bad data. If you have bad data, that fuels bad process. You’ve got a process issue to find and fix. It’s important to have clarity as to who owns which data -- and hold people accountable for this data.”
Data is often viewed as an IT problem, but it’s really a business problem. That means leaders need to care more about this issue and understand that data is directly linked to business outcomes.
7. Poor metadata
Metadata is information that describes data, from the type of data to where and how it was captured. It is crucial from a governance and usage standpoint. In addition, poor metadata can often be a culprit for downstream problems.
“[O]rganizations should adopt comprehensive data governance policies, invest in automated data quality solutions, and foster a culture of data stewardship. Moreover, they must focus on continuous data quality monitoring and improvement to support accurate and reliable AI analytics, says Guru Sethupathy, co-founder and CEO of AI governance software company FairNow.
He also warns about inconsistencies in data calculation. “At the calculation stage, data quality issues frequently arise from poorly defined or inconsistently applied metrics. These inconsistencies can distort analytics outcomes and undermine trust in AI-driven insights,” says Sethupathy in an email interview.
8. Data silos
Yes. Data silos are still a problem. Despite that everyone is sick of hearing about them, customer data, for example, is typically fragmented across CRM, billing, customer service, interaction management, call recordings, and more.
“This fragmentation makes it incredibly difficult to serve a real-time and reliable customer view to the underlying LLMs powering customer facing GenAI apps,” says Yuval Perlov, CTO of operational data management software provider K2view in an email interview. “To overcome this challenge, you’d need a robust data infrastructure capable of real-time data integration and unification, master data management, data transformation, anonymization, and validation. The more fragmented the data, the steeper the climb towards achieving high-quality data for GenAI applications.”
9. Inaccurate data lineage
Inaccurate data lineage is caused by IT issues, lack of exception handling and moving data across various sources files and formats.
“The more data gets processed across multiple environments, applications, people, the greater the likelihood of something going wrong,” says Bryan Eckle, chief technology officer at data analytics and financial management solutions provider cBEYONData in an email interview. “Sloppy data processing can happen if a coder doesn’t handle a special character, systems crash, files get corrupted, or some new anomaly appears in the data over time.”
10. Software
Software captures data, and the capturing of that data will reflect errors, bugs, or misalignment of thoughts when the software was written based on how the data capture occurs. As long as that’s a reality, the data that comes in from a software process will always have some sort of issue, according to Avi Perez, co-founder and CTO of business and decision intelligence platform Pyramid Analytics. For example, if a particular piece of software doesn’t capture a customer’s phone number, it’s impossible to do any sort of analysis of sales by phone number.
“The second issue that happens a lot, especially in the enterprise, is that there are five different pieces of software that are all capturing one piece of the puzzle of the company. And at some point, you’re going to be asking questions where you need to glue all five of them together,” says Perez in an email interview. “And now you have a headache because the data that is captured in all five systems, while there are pieces of the data that are different. [A]t some point, there’s something analogous and common to all of them, hence the gluing of them. And if that is not done properly, then the glue will not work. Our data needs to be nearly perfect for it to be matchable. Otherwise, we wind up with what’s called, ‘fuzzy matching.’ It seldom works flawlessly.”
And the “glue” can be problematic: It can be difficult to glue together the systems collecting similar data and the outcome tends to have a lot of holes because of the incongruency of the data.
11. Messy data
Most organizations already have an enterprise data platform that collects and integrates data across source systems, but the data assets in these platforms are often disorganized and messy. As a result, data consumers are not aware of the full extent of data assets available, and even when they can locate an asset, they may spend a lot of time cleaning it before it can be used.
“Investment in data governance and management programs will help address general data quality issues. For most organizations, the sheer size and scope of data being collected make it impossible to fix all general data quality issues. Therefore, organizations should try to keep these programs focused on the data assets that are most frequently used by data consumers,” says Tyler Munger, VP analytics, global operations at enterprise software support, products, and services provider Rimini Street in an interview.
If organizations want better-quality data, Munger says they need to incentivize or reward the people who are performing the data capture. A key part of this process is the regular measurement of data quality and setting goals or targets. This can be done by regular data audits or directly measuring the improvement in the AI system's performance.
About the Author
You May Also Like