4 Ways to Make Data Analytics More Efficient4 Ways to Make Data Analytics More Efficient
Finding a path forward that is profitable and sustainable in the face of rising cloud costs, economic uncertainty, and burgeoning environmental crises is a struggle. Here are some tips.
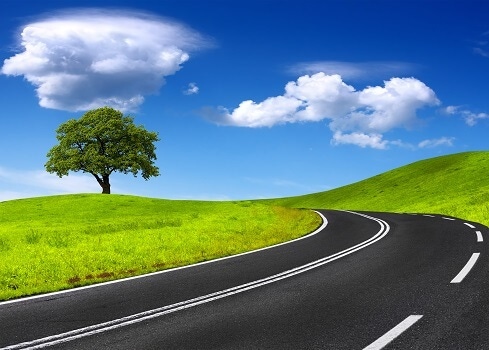
Widely available scalable data tools, cheap storage, and advanced analytics tend to make us think that seeing all data and knowing all outputs is the path to prosperity. But in truth the exercise leaves many organizations stuck on the informational highway somewhere between a boondoggle and a tar pit.
Finding a path forward that is profitable and sustainable in the face of rising cloud costs, economic uncertainty, and burgeoning environmental crises is still a struggle.
“It’s true, and easy to forget, that clear goals lead to more efficient analysis,” says Tim Panagos, chief technology officer and co-founder of Microshare, a smart building technology and data firm. “By establishing a clear business intent from the outset, analysts can focus their efforts on pertinent data, relevant tools, and suitable methodologies, thereby avoiding unnecessary detours and resource consumption.”
Fortunately, there are several ways to make analytics more efficient. Let’s take a look at a few:
1. Data sorting by the numbers
Do you know who in your organization is using what data? That information is key to figuring out how you can begin to make analytics more efficient. Start by analyzing your analytics use cases and workflows.
“The key to efficiency here is understanding the context around your data and not simply relying on automation to categorize and optimize it for you,” says Ian Smith, field CTO at Chronosphere, a platform that helps teams curb cloud costs and resolve incidents. “For example,” he says, “if 80% of the data in your data lake has never once been accessed, then dropping it from your analytics might be an easy call to make.”
“But if there’s another 15% that is only accessed by one person or team, understanding the context around that access might be very important. If that 5% of data is only being used by one person, but that one person is the chief data officer, who looks at it every month… then you may want to keep that data,” Smith adds.
2. Check to see if the data you have contains the answer you seek
Yes, you have lots of data. Everyone does. But is there an answer to your problem in it? If you want to make your analytics more efficient, first make sure that the required output is actually possible.
“Explore your data and look for strange patterns, outliers, or simple errors. Sometimes the data that is available simply doesn't allow an answer to the question that is being asked,” warns Michael Berthold, CEO of KNIME, a free and open-source data analytics, reporting, and integration platform.
So how do you discover that dismal fact early on?
“Start with explanatory data analysis of the dataset,” explains Katy Salamati, senior manager for AI and analytics at SAS. “This will help you understand the quality of the data and distinguish noise from outliers. Then build data models to uncover relationships within the data. With the emergence of powerful AI and machine learning technologies, you can now look at more and more of the data -- even all of it – not just a sample.”
3. Test AI models and pipelines
Inefficiencies can exist in the AI models you are using to do your analysis. Do not assume that a model lasts forever or that there is none better. Data drift and other issues can throw the best of algorithms in a tizzy.
“Tapping automated modeling facilitates quickly building and testing many models before identifying the best or champion model. And advanced modeling tools allow users to constantly tune and retrain models to ensure they continue to deliver analytical insights,” Salamati says.
Consider implementing decision intelligence methods to improve the efficiency of your outputs in terms of better business alignment. Last but not least, check your data and AI pipelines.
“Data intelligence needs to be step zero in any analytics process, whether the task is fancy data pipelining or simply making a spreadsheet for a shareholder meeting. DI is the layer that precedes AI and BI to ensure that only relevant, accurate, trustworthy data gets fed to algorithms and analytics,” says Aaron Kalb, co-founder and Chief Strategy Officer at Alation, a provider of data catalog, analytics, and data management.
“Most bad corporate decisions and much of knowledge workers’ wasted time can be tracked to bad data -- ungoverned, mismanaged, or otherwise low-quality data -- at the start,” Kalb adds.
4. Know when to quit
Somewhere along the way, enterprises became convinced that they should mine all their data, even dark data, and wring every last bit of profit from it. Never mind that isn’t always -- or even often -- a fruitful exercise.
“Anyone who has ever done data analysis has gone down the rabbit hole and ended up hours later thinking, 'what was I doing?' While segues and offshoots might help you form an even better set of analytics, they can just as often distract from the initial question or task,” says Kathy Rudy, chief data and analytics officer with global technology research and advisory firm, ISG.
Things keep getting worse if companies keep trying to find paydirt after they’ve hit nothing but data dirt.
“Analysis can often be an open-ended pursuit and doesn't always have a clear ending. Where people get bogged down is when they chase analysis without business leadership helping to drive a decision that needs to be made, with a clear understanding of the impact and timing of the decision,” says Bret Greenstein, partner, data, analytics, and AI, at PwC.
“Data-driven business leaders understand the difference between studying and deciding, and they make it clear to their teams what level of confidence and accuracy is needed for that decision. Without that, analysis never ends,” Greenstein adds.
What to Read Next:
About the Author
You May Also Like