A Plea to the Private Sector: Follow DOD on Data Strategy TenetsA Plea to the Private Sector: Follow DOD on Data Strategy Tenets
In 2020, the Department of Defense created a data management vision that any enterprise would do well to emulate.
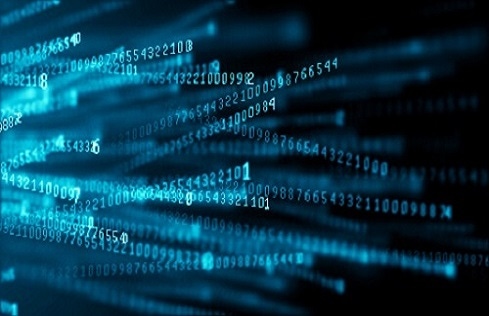
A wise person once said, “Do something today that your future self will thank you for.”
One could argue the U.S. Department of Defense applied that philosophy during autumn 2020, when Pentagon officials publicized its new data strategy.
A year and a half later, the business community also should be thanking DOD for its foresight. Defense officials outlined in plain English a master data management vision that any enterprise would do well to emulate.
VAULTIS
The Pentagon listed seven tenets under the acronym VAULTIS. It decreed that data should be made visible, accessible, understandable, linked, trustworthy, interoperable, and secure.
Implementing such an approach inherently increases an organization’s efficiency. Extracting value from data within this type of structure becomes an effortless exercise. Data becomes accessible, eliminating hurdles to make it actionable.
This is key to ensuring a company’s future viability and competitiveness. The Department of Defense has laid out a superlative high-level way of thinking how businesses must change their views on data to outperform rivals.
But it requires pivoting one’s mindset to that of a data-centric architecture -- one where data no longer is beholden to a singular application or exists in proprietary siloes. A company on this data-fabric path makes its data value core to achieving speed and scale.
Data as a Differentiator
I’ll illustrate using a personal example. I recently refinanced my home through Better.com. All the information gathering -- pulling the W2s, obtaining the house deed, collecting other forms -- and complex analysis was completely digital. If I had done it through Wells Fargo, where a person would have had to check 15 systems and figure everything out, the bank would have charged me half a percentage point more in interest because it has to cover all those expenses.
That’s an example of a company applying strategic data in real time.
It’s a phenomenon that’s just starting. Soon, every industry is going to be like this. Tesla already is disrupting the car industry as a data-centric organization: from direct-to-consumer sales instead of buying through dealerships to models automatically receiving software updates.
Weaving a Data Fabric
Getting there from here might not be easy or cheap, however. The best course of action is to put down the shovel. Stop digging yourselves into deeper data rabbit holes. Starting now and going forward, avoid creating single-app datasets, data silos and discrete datasets that can’t communicate with each other.
Problems plaguing many companies today include deciding whether to spend the money required to migrate data from an obtuse format to one that’s getable and usable. This is costly. Executives who need to bridge that gap will do so only in the most high-priority cases. One possible solution would be to deploy a data mesh, which makes possible accessing and querying data where it currently resides.
Some companies are employing semantic data catalogs to make information visible, but even then, accessibility still could be a challenge. A typical difficulty is establishing a language common to various datasets. Data integration today composes more than a third of the average IT department budget.
This is one of the obstacles solved by the W3C standards. That set of technical specifications and guidelines enables an open web platform with many features, including semantic interoperability -- a shared language, in other words, where data systems communicate through a universal vocabulary.
Such a framework allows for communication among machines when no human interaction occurs or the data stems from disparate sources. It also powers relationship discovery among datasets. Semantic interoperability makes it possible to draw inferences from the data. For example, if two individuals in different datasets possess identical names, homepages and email addresses, the inference is that these two are the same person.
Data Self-Defense
Ultimately, we want to be working in an environment where all data also is trustworthy and secure. That includes data individuals and companies must connect and communicate with, but don’t necessarily own or directly control.
This is the realm where data must defend itself across contexts, domains, users, and networks. Automated and scalable security logic software replaces individual source implementation. Data should possess provable provenance.
The consequences of trustworthy and secure data reach far beyond whether your computer warns you that a website you’re visiting is unsafe.
With self-driving cars likely to become a reality in the near future, data trustworthiness becomes literally a matter of life or death. The algorithms and artificial intelligence systems that will run autonomous vehicles also must be nimble and tactical. Data exchange among the car or truck, municipal-run traffic lights, the surrounding vehicles in these situations must be seamless and unbreachable with minimal latency.
What’s required further includes proof of data origination and modification, data-integrity detection capability that discerns the tampering of even a single pixel or letter and identity verification.
Math and cryptography already are being used in everyday use cases to provide individuals with verifiable credentials. Whether we’re talking about a digital school transcript or a digital vaccine card, applying those methods makes disinformation impossible. The data is independently verifiable. No need exists to hire a third-party auditor.
Replicating DOD’s approach to data at the very least ensures your company an edge. More importantly, however, private-sector adoption of VAULTIS guarantees a healthy free market.
About the Author
You May Also Like