Can Analytics Outperform The Machine Whisperer?Can Analytics Outperform The Machine Whisperer?
The Internet of things promises to spot industrial failures before a human expert could. Will you trust that data?
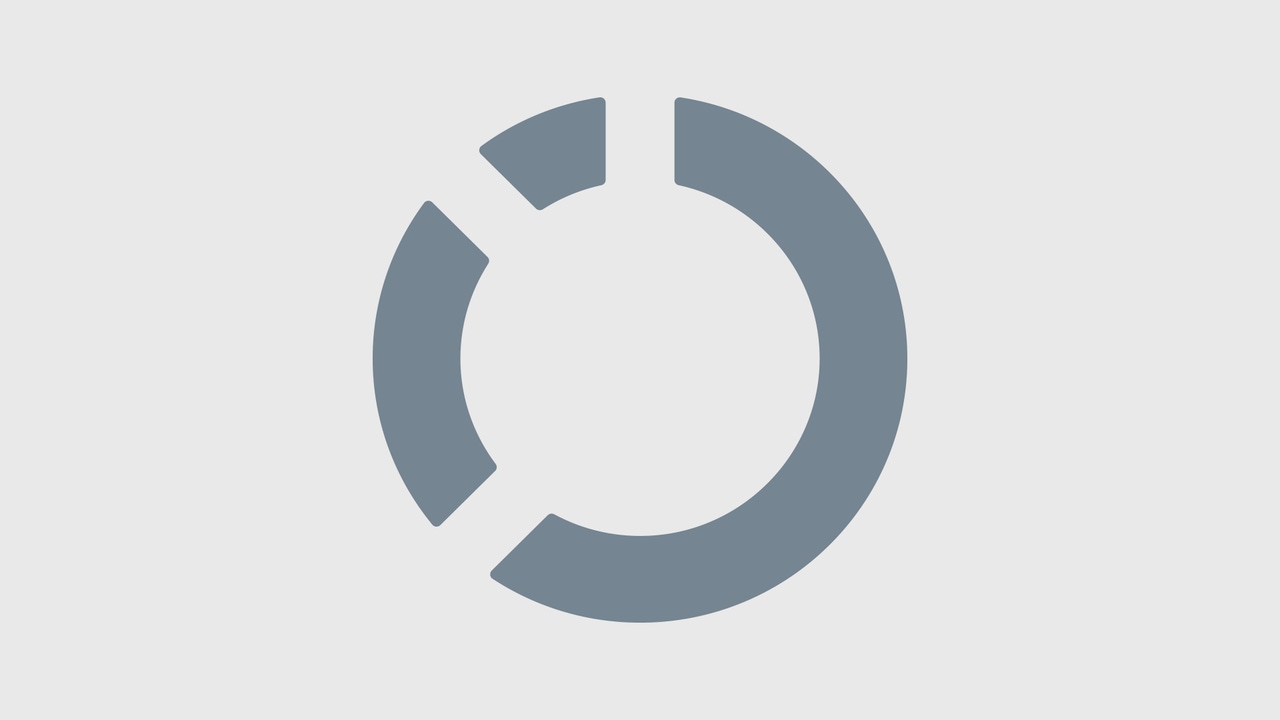
Thanks to analytics software and the Internet of Things, we're opening a new round in the never-ending bout of man vs. machine.
During a panel discussion this week at a General Electric Industrial Internet event (GE's name for the Internet of Things), the insight that got the most heads nodding came from Jay Neidermeyer, CIO of GE Aviation's supply chain and manufacturing operations.
Neidermeyer was describing GE Aviation's early efforts using GE Intelligent Platforms software for predictive analytics to anticipate when a CNC machine used to make airplane engine parts is about to break down, so that staff can do preventive maintenance and avoid delaying the delivery of a finished engine. On many factory floors, he noted, "there's the person who can predict the outage based on hearing the rattle." Neidermeyer said:
"It's hard to convince that person that this computer might actually have some insight that he or she doesn't. We're still kind of learning our way through that. I think our strategy is pretty simple -- let's find simple ways to demonstrate success. And by the way, maybe not even taking action on them yet. Just have the signals in place so you can say, 'I think something might be coming. Let's see if it's reality,' and let people observe an outcome together. The theory is that if some of these things work, it's going to start showing itself that the insight you gain from these methods is as good or frankly better than that person -- than the 'machine whisperer.'"
My sense is that GE Aviation is typical in being only in the earliest stages of using predictive analytics to understand when a machine or some other process might fail.
Global CIOs: A Site Just For YouVisit information's Global CIO -- our online community and information resource for CIOs operating in the global economy.
Rather than applying true predictive analytics based on actual performance metrics, what I see companies doing most often is relying on averages. To use a car example, we're told to change our fuel pump at 65,000 miles because company research shows that the pump generally fails sometime after that point. That scenario is a lot different from changing the fuel pump because the real-time data tells us that pump is running poorly and will fail and leave you stranded on the roadside at 45,000 miles.
Averages won't be good enough to achieve GE CEO Jeff Immelt's Industrial Internet vision of guaranteeing customers no unplanned downtime on their critical GE equipment. It's the exceptions -- the pump that fails 20,000 miles too soon -- that cause unplanned downtime.
Predictive analysis based on actual operating data is one of the big promises of the Internet of Things, as sensors gather data from devices and analytics software allows constant monitoring for problems. Some technical barriers remain, including the ability to gather the right data on which to base decisions.
But Neidermeyer's example points to another barrier: Do you really trust the data? His example resonates because it's human -- we can picture that wise veteran who has seen it all before and doubts this newfangled data. But we're all machine whisperers, relying on our experiences to make decisions about the future, so any Internet of things initiative must prove its data is strong enough to overcome our past.
About the Author
You May Also Like