Clean, Lean Data Is the Cornerstone of AI SustainabilityClean, Lean Data Is the Cornerstone of AI Sustainability
Messy data is making AI inefficient and hampering its sustainability. So why aren’t more organizations doing a better job of optimizing their data for AI?
October 14, 2024
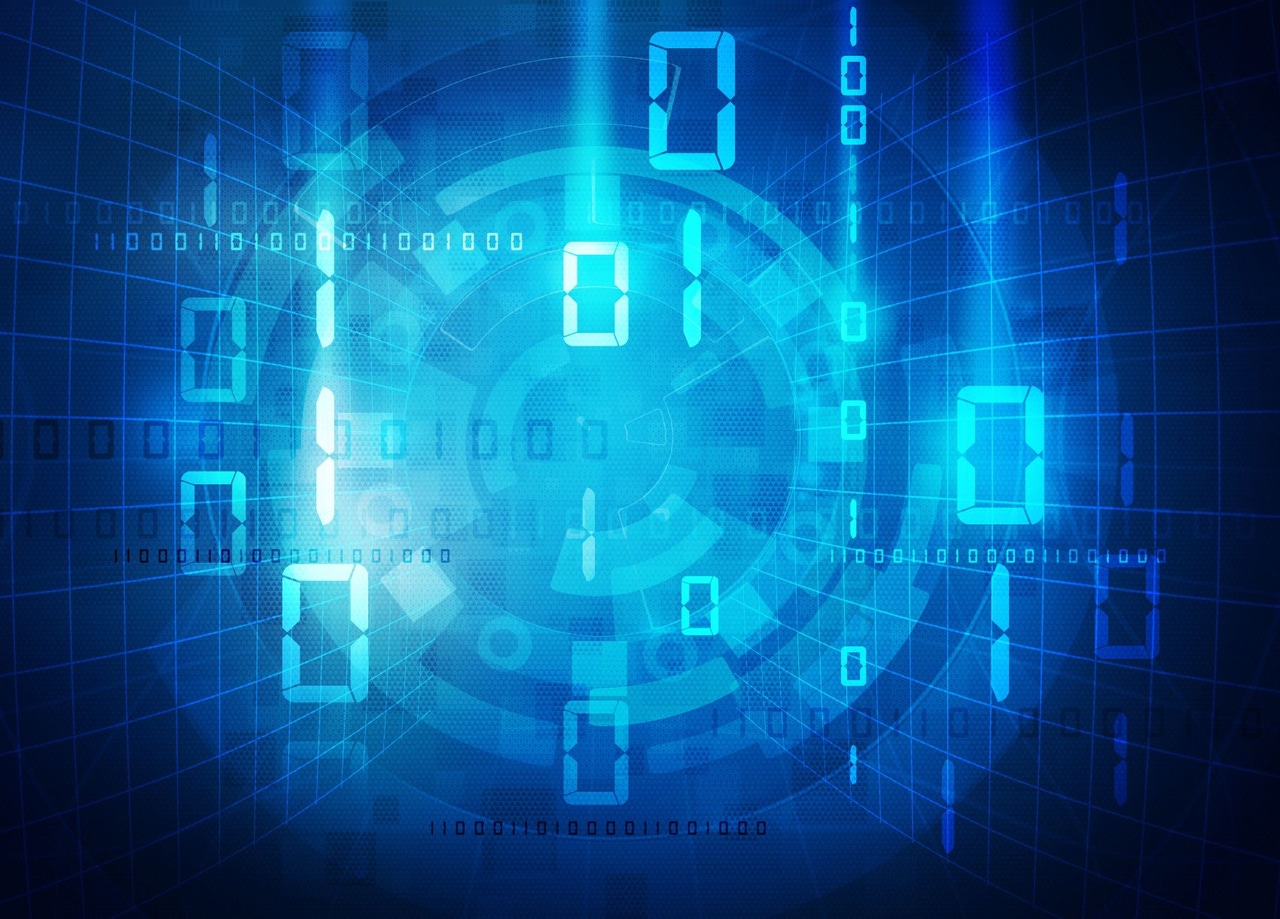
Worries about the environmental impact of AI workloads are no longer a discussion limited to researchers and technologists. Debates about the projected power crisis and climate impacts associated with running predicted AI demands have also started popping up at dinner table and water cooler conversations. Given the promising breakthroughs experts predict AI will make to global challenges in healthcare, climate science, and sustainability, it makes sense that people are interested. Yet, we must carefully prepare ourselves to manage AI’s potential downfalls while celebrating its promising potential.
At its core, the environmental impact of AI workloads is a concept that is fairly easy to grasp for even nonexperts: AI models run on extremely powerful, energy-hungry computers. And since most electricity production creates greenhouse gas emissions, AI could cause carbon emissions to skyrocket if left unchecked. The solution, however, is far from simple.
To help organizations avoid getting bogged down in the many variables of the AI sustainability conundrum, I recommend breaking down AI sustainability into five areas of efficiency, beginning with data efficiency. As a general rule, making IT more sustainable requires us to do more with less, maximizing system efficiency to get more output from fewer resources. The five pillars of sustainable IT that I walk my customers through are: equipment efficiency, energy efficiency, resource efficiency, software efficiency, and data efficiency.
Although addressing each efficiency area improves AI sustainability, given the data-intense nature of AI workloads, organizations need to start by optimizing the data sets they feed into their AI models.
How to Tackle Data Efficiency for AI Workloads
Map out your data strategy upfront: Start with knowing what data you need, where it will come from, how often you’ll collect it, the process you’ll use to gain insights from it (for example, which AI models you’ll use), how data will be moved between systems, where and how long you’ll store it. Can data be consolidated, disposed of, or stored using low-impact techniques, such as tape or other backup methods? Data that does not need to be retrieved immediately can often be offloaded to more low-energy media.
Clean up before you start: For traditional workloads, data efficiency used to mean we focused on only storing data that we were going to generate business value. But for AI workloads, data sets need to be adequately sized, adequately cleaned up and optimized BEFORE training a model -- because when you simply use off-the-shelf data sets or repositories without minimizing them before model tuning, you end up doing unnecessary work and making the AI solution work harder.
Get the training data set right: Getting the data set optimized in the first place, before you do the training, is a key part of AI sustainability, and then you can use your customer’s specific data as you tune that model as well. By starting first with the data efficiency -- and getting that data population as concise as it can be from the first early stages of the process -- then you’re driving efficiency all the way through.
Process data only once: Data used for training/tuning should be processed only once, with additional retraining/fine tuning happening on only the new data being collected
Avoid data debt: Managing and maintaining data becomes especially critical with AI workloads because they require such massive amounts of data, including unstructured data. One way to ease the pressure on data storage systems is to get rid of inaccurate, erroneous, out of date, or duplicated data. Data debt, like technical debt, becomes problematic in AI systems because AI results hinge on the data fed into the models.
Location matters: Data should be processed as close to the original location as possible to minimize the energy implications of movement and the timeliness of the information.
About the Author
You May Also Like