Data Quality: The Strategic Imperative Driving AI and AutomationData Quality: The Strategic Imperative Driving AI and Automation
Here are some simple strategies for gathering and sharing data that can help drive success.
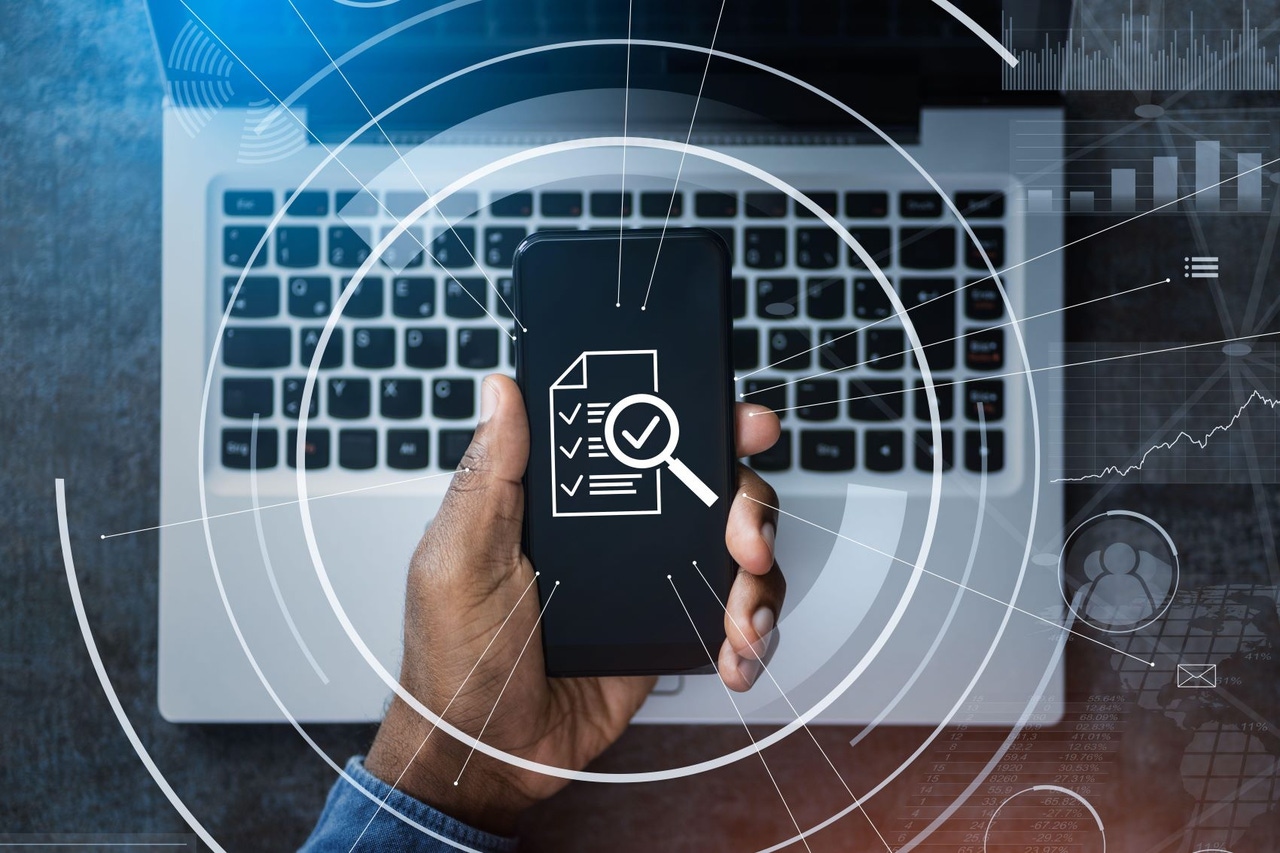
As enterprises race to implement AI and automation, one often overlooked factor can make or break their success: data quality. In fact, 72% of enterprises have adopted AI for at least one business function. The success of these AI and automation initiatives hinges on quality data. What separates effective automation from costly failures often boils down to the quality of the data feeding these systems. To achieve effective automation, enterprise leaders must rely on high-quality data. In this article, I’ll outline simple strategies for gathering and sharing data that drives success.
Data Quality: Impact Across Industries
The implications of poor data quality can vary across industries, but the underlying risks remain similar. For instance, in healthcare, poor data can lead to poor patient care, putting their safety at risk. Financial services are another sector where data accuracy is paramount -- poor data quality leads to flawed financial reports and increased operational risks, eroding trust, and potentially incurring regulatory penalties. Even retail isn’t immune, as inaccurate inventory data can lead to poor stock management decisions, resulting in costly stockouts or excess inventory.
Data Quality Checklist
High-quality data empowers automation and AI to provide outputs that are accurate, reliable, and context-rich, enabling users -- from data analysts to business leaders -- to make informed, confident decisions. This requires data to meet a checklist of criteria, which are as follows:
Timeliness: Is your data up to date? Timely data ensures relevant decision-making. For example, relying on outdated customer data in retail can lead to inaccurate personalization, missing opportunities for sales.
Accuracy: Does your data accurately represent real-world conditions? Eliminating biases or errors is critical. For instance, biased healthcare data can lead to improper diagnoses, directly impacting patient outcomes.
Completeness: Are your datasets comprehensive? Incomplete data can distort AI outcomes or even lead to “hallucinations,” where algorithms generate inaccurate or misleading results. For example, missing sales data could result in flawed revenue forecasts.
Consistency: Do your records align across datasets? Inconsistent data creates errors that can ripple across automation systems. Imagine a supply chain scenario where mismatched product IDs lead to shipping delays and increased costs.
Building a Foundation of Quality Data
Ensuring data quality is not just about data cleansing; it requires robust data governance and management practices. Implementing a framework that prioritizes data quality across the organization is essential to achieving reliable outcomes from AI and automation investments. Here are a few best practices:
Data stewardship: Designate individuals responsible for monitoring and maintaining data quality across its lifecycle. This ensures that the integrity of the data is preserved.
Automated data validation: Proactive detection and correction of errors in real-time is essential for organizations that rely on up-to-date data for fast-paced decision-making.
Data lineage tracking: By tracking data from its origin through its transformations, organizations can better understand its reliability and accuracy.
Hyperautomation as a Data Quality Use Case
Hyperautomation, as defined by Gartner, is reshaping business by automating end-to-end processes across the entire IT landscape. This process merges AI, machine learning, and robotic process automation (RPA) to streamline operations, cut costs, and elevate customer experiences. However, the effectiveness of hyperautomation depends on one crucial factor: data quality. This is because the intelligence behind hyperautomation -- AI and ML models --relies on data.
In hyperautomation, data-driven decisions are vital for optimizing processes. Poor data quality can lead to less effective choices, undermining efficiency gains. Analyzing historical data allows organizations to forecast trends and proactively automate, yet the accuracy of these predictions is only as good as the data they’re based on.
Hyperautomation also requires integrating data from multiple sources, and inconsistent formats or quality issues can impede seamless integration and scalability. High-quality data helps ensure the reliability and robustness of hyperautomation initiatives, minimizing errors and system risks.
For customer-facing hyperautomation projects, such as applications like AI-powered chatbots and virtual assistants, these depend on accurate, current data to respond effectively to inquiries. Organizations that focus on data integrity while deploying hyperautomation projects -- both internal and customer-facing -- can fully harness its potential, enhancing operational efficiency and gaining a competitive advantage.
Long-Term Impact of Quality Data on Business Strategy
At the strategic level, high-quality data doesn’t just make AI and automation systems work better -- it enhances business outcomes. With data that is complete, accurate, and timely, companies can leverage AI and automation to improve efficiency, reduce operational risks, and foster more data-driven decisions that strengthen competitive advantage.
Organizations that prioritize data quality today will be the ones to define industry benchmarks tomorrow. The question is: Is your data strategy ready to meet the demands of AI and automation?
About the Author
You May Also Like