Data Science: Why Humans Are Just as Important as MathData Science: Why Humans Are Just as Important as Math
It’s not easy to teach machines how to apply a critical lens to data. Businesses need human intuition and creativity for multi-faceted problems.
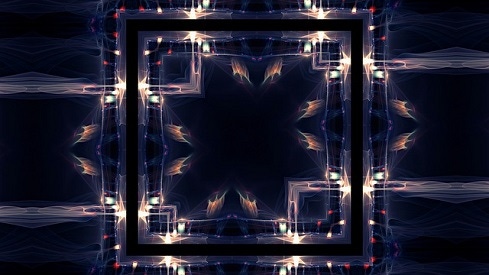
Data science rules everything around us. Recommendation algorithms that predict what we’ll want to watch, buy, and read are now ubiquitous, in part thanks to advances in computing power. But while today’s data science tools can sift through mounds of data to unearth patterns at levels of scale and speed that humans alone could never achieve, our models remain inadequate in fully understanding data and its applications, especially when the data becomes messy in reflecting fickle human behaviors.
Data science is a craft that relies on human intuition and creativity to understand multi-faceted problem spaces. Without human oversight, it operates on an incomplete picture, for which the implications have never been clearer in the present COVID-19 age as our algorithms struggle to grasp the reality that human behaviors don’t follow mathematics.
March 2020 marked the start of a series of behaviors that would have seemed unusual just weeks prior: As COVID-19 was declared a global pandemic, we started stockpiling toilet paper, Googling hand sanitizer, and searching for masks. As humans, we understand the cause and effect relationship at play here. These were our reactions as we learned more about the spread of the coronavirus. But for machine learning algorithms, these sudden behaviors represent data gone haywire, confusing our models and affecting the usability of resulting insights.
In many cases, machine learning (ML) depends on historical data to inform predictions. Therefore, when humans produce anomalous data, our models can struggle to make recommendations with the usual degree of confidence. From supply chains to financial forecasting to retail, every industry must think carefully about the data it’s collected over the past few months (do these aberrations represent our new normal, or are they one-time deviations?), and how it will be treated moving forward. By illustrating how our ML models aren’t always designed to withstand extreme data swings, the pandemic has demonstrated why we’ll always need human involvement to interpret and fine-tune the art of data science.
Data is volatile and ML models are reactive
No amount of stress-testing could have prepared even the most sophisticated machine learning models for the extreme data variation that we’ve witnessed in the past few months. Analysts and data scientists have had to step in to calibrate models. The ability to apply a critical lens to data and insights is not one we can readily teach machines. Overlooking this important step of the process leaves us susceptible to falling into the hubris of big data and making decisions that miss important elements of context.
For example, we saw an increase in demand for nonperishable foods across the supply chain, but once everyone has stockpiled their pantries, they’re unlikely to buy these items in similar quantities in the coming months. This will naturally lead to a drop in demand that we must prepare algorithms for, instead of automatically continuing to operate production lines as if such demand is the new normal.
Another example is a machine learning application in cybersecurity, in which an algorithm may monitor for threats against a retailer’s website. To the model, a sudden tenfold increase in website visits may seem like an attack; but, if you were to factor in that it coincided with the retailer launching mask sales, you have the context to understand and accept the uptick in traffic. Data has meaning beyond what can be gleaned from looking at algorithmic outputs, and it’s up to data scientists to understand it with the help of machine learning, not the other way around.
Adapting models to a changing normal
Data science can be thought of as a magical sword that knows certain forms and attacks and can even move on its own to some degree. But while the sword knows how to cut, it does not necessarily understand what, when, and why to cut. Similarly, our algorithms know how to make sense of the data we have at scale but are unable to fully comprehend the span of human behaviors and reactions. For example, based on recent trends, algorithms might advise supply chains to continue producing large quantities of yeast, whereas human reasoning may suggest that demand for yeast will soon drop as shelter-in-place restrictions lift and people get tired of baking bread.
The pandemic has confirmed that a “set and forget” approach to data science is not the end goal for our industry -- there is no wand to wave to automate the dynamic process of data science. We will always need humans to bring in the real-world context that our models operate in. Now, more than ever, real-time monitoring and adjustments are essential to yielding insights that matter. As data scientists take a long, hard look at the aberrant data and resulting insights from recent months, we must remember that even during “normal” times, we have a responsibility to actively assess our data and refine our models to avoid unintended consequences before they trickle through the decision-making process.
The world doesn’t operate with fixed boundaries, and neither can applied data science. As data scientists, our intuition helps bridge the gap between data science in the development environment versus reality. When uncertainty is the only constant, this current point in time is a proof point for the importance of human intuition in data science as we make sense of the changing situation and help our algorithms do the same. The fundamental law of data science is that your predictions are only as good as your data. I have an addendum: Your predictions are only as good as your data and the scientists that steer it.
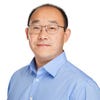
Peter Wang has been developing commercial scientific computing and visualization software for over 15 years. He has extensive experience in software design and development across a broad range of areas, including 3D graphics, geophysics, large data simulation/visualization, financial risk modeling, and medical imaging. Wang’s interests in vector computing and interactive visualization led him to co-found Anaconda. As a creator of the PyData community and conferences, he’s passionate about growing the Python data science community, and advocating and teaching Python at conferences. Wang holds a BA in Physics from Cornell University.
About the Author
You May Also Like