Data Strategy: Synthetic Data and Other Tech for AI's Next PhaseData Strategy: Synthetic Data and Other Tech for AI's Next Phase
Forrester's Data Strategy & Insights event will offer insights to help you take your machine learning and artificial intelligence initiatives to the next phase.
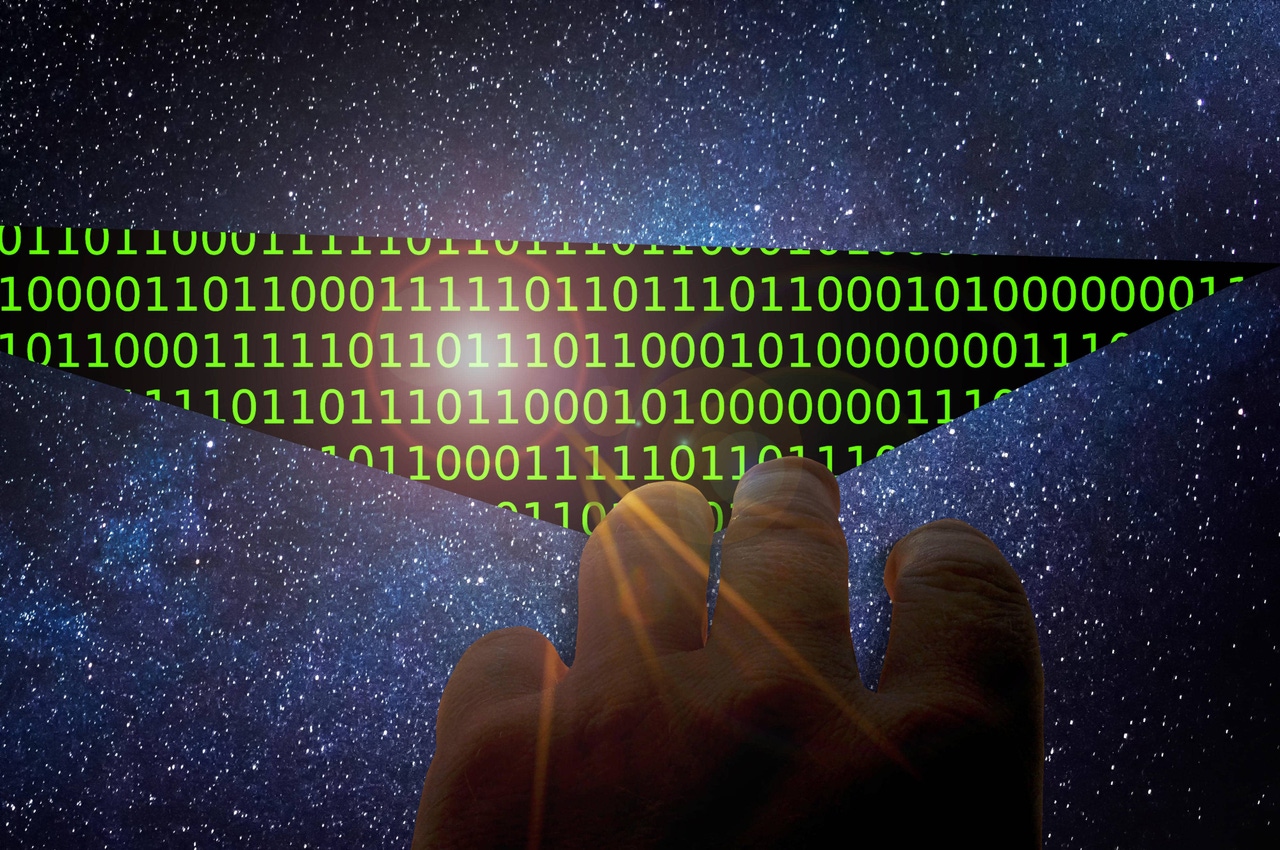
You’ve finally gotten your enterprise’s machine learning and artificial intelligence into production and your top executives are expecting results. Just one question: Do you have enough quality data to train those algorithms?
Now that enterprises are plowing ahead with these initiatives, sourcing data for the always-hungry algorithms will be a constant item on the to-do list. There can be obstacles to gaining access to needed data. There’s a limited amount of data that can be collected and cleaned by your own enterprise. New and existing privacy rules can limit data collection and storage. And there are some events that are so new that there’s not much if any data available to train an algorithm -- say for a pandemic that leads to a supply chain crisis.
One solution to these all these use case challenges is synthetic data. The topic will be among many covered by Forrester at their Data Strategy & Insights event, December 6 and 7, as organizations lean into the next era of machine learning and other artificial intelligence in the enterprise. Forrester analyst Rowan Curran will be among the presenters of a session on the synthetic data topic, “The Value of Tilting at Windmills: Synthetic Data in AI and Beyond at the event. Curran spoke with information about the upcoming session and the promise of synthetic data.
Synthetic Data: What is it?
According to Forrester, synthetic data is training data of any type (structured, transactional, image, audio, or other types) that duplicates, mimics, or extrapolates from the real world but maintains no direct link to the real world, particularly for scenarios where real-world data is unavailable, unusable, or strictly regulated.
“This is something that I think will become super interesting and a very important part of the AI landscape moving forward,” Curran says. He offers a couple of use cases to explain the potential of synthetic data.
For instance, one use case of synthetic data was designed to help auto makers collect computer vision data about what sleepy drivers look like. This was to comply with driver monitoring systems that may become a regulatory requirement in Europe and the US. Here are two options for how a company would collect that data. In Plan A, the company would hire actors from multiple demographic groups to feign fatigue, distractedness, and sleepiness, explains Curran. But this is an expensive and time-consuming process when organizations typically need lots of data quickly. Plan B called for partnering with a synthetic data company to simulate images of people looking tired, fatigued, sleepy, or distracted. This process yielded a much larger training set of quality images.
Curran explains that other applications of synthetic data could help, say, the human resources organization in a large multi-national company. For instance, an HR person is able to train an application with their voice and a video recording. Then the AI generated voice and video simulation of the HR person is fed with text scripts. The application then will produce one unique video for each unique script. This is useful for the HR organization that needs to make videos for employees in 100+ different countries, personalized for each country’s customs and language. Recording each video separately would take a huge amount of time. But training the app and then generating many videos using scripts can speed the process and reduce resources required.
Other AI Technologies You Should Know About
Synthetic data is one of several AI technologies identified by Forrester as less well known but having the power to unlock significant new capabilities. Others on the list are transformer networks, reinforcement learning, federated learning and causal inference.
Curran explains that transformer networks use deep learning to accurately summarize large corpuses of text.
“They allow for folks like myself to basically create a pretty concise slide based off of a piece of research I’ve written,” he says. “I already use AI-generated images in probably 90% of my presentations at this point in time.”
The same base technology of transformer networks and large language models can be used to generate code for enterprise applications, Curran says.
Reinforcement learning allows tests of many actions in simulated environments, enabling a large number of micro-experiments that can then be used for constructing models to optimize objectives or constraints, according to Forrester. For instance, Curran says, if you are a big manufacturer and you get an alert that a piece of equipment may fail and should be taken down for maintenance, but you are just at a critical rush time. Such a simulation would let you account for your big order, the cost of shutting down at peak season, and other factors in your decision of whether to take that piece of equipment down for maintenance.
Federated learning is a managed process for combining models trained separately on separate data sets that can be used for sharing intelligence between devices, systems, or firms to overcome privacy, bandwidth, or computational limits. Causal inference enables a deeper dive into cause-and-effect relationships in data that can be used for business insights and bias prevention when explainability may be as important as prediction accuracy, according to Forrester.
The upcoming Forrester event will cover aspects of these technologies to help organizations as they move into the next phase of AI implementations. Other sessions are The Seven Habits of Highly Trusted Artificial Intelligence, Get your Data Storytelling Starter Kit Today, and Future-Proof Your Data Architecture with Data Fabric 2.0
Those interested in attending Forrester’s Data Strategy & Insights Forum, taking place December 6–7, 2022, can register with voucher code FORRIW.
What to Read Next:
How Synthetic Data Accelerates Coronavirus Research
About the Author
You May Also Like