Divining ActDivining Act
Each forecasting method has its own strengths and weaknesses. Our expert provides an overview, with particular focus on univariate versus multivariate methods.
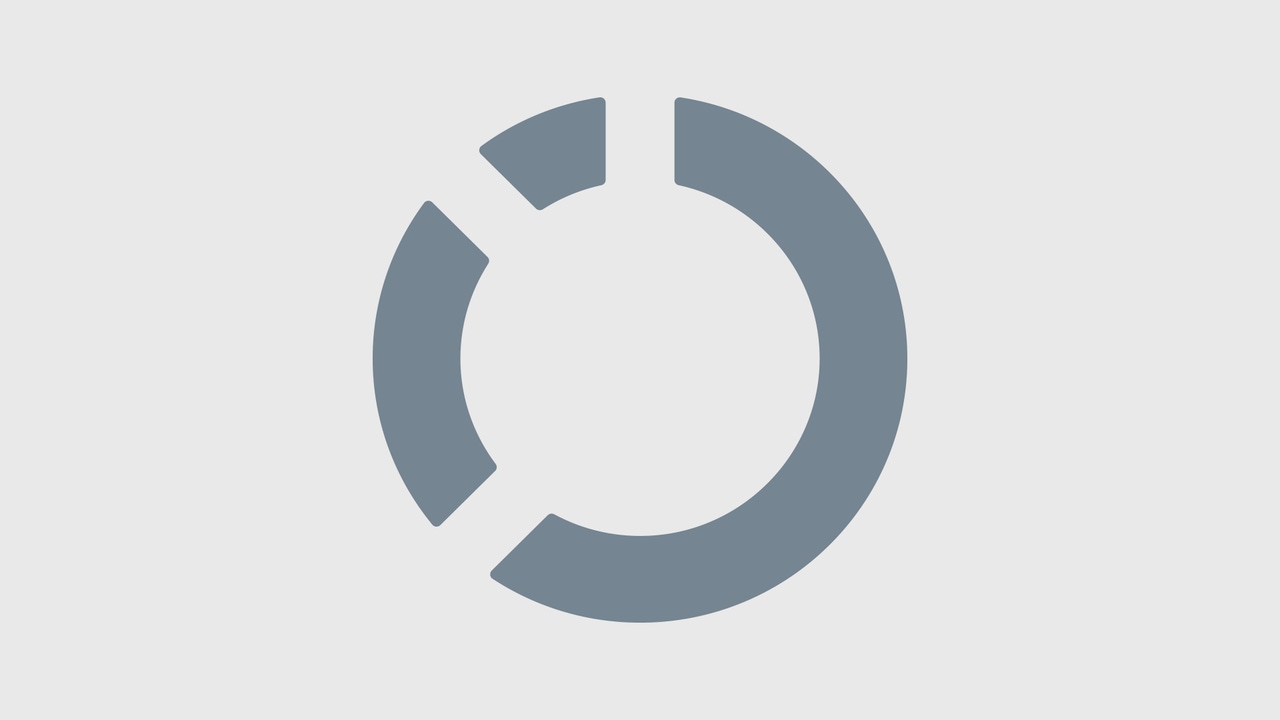
Multivariate Forecasting
Multivariate methods, which are better long-term predictors than univariate forecasts, can be quite complex and are sometimes inconsistent. They also can be costly.
For example, there has been and continues to be a great tussle between fairly different VARMAX, state space, neural network and econometric approaches to multivariate forecasting (see Glossary, below). A related problem is that for the added cost and effort involved in multivariate forecasts, there may not be a great ROI. Multivariate methods and practitioners, like univariate forecasters, have a good and sometimes spectacular record for predictions, but the complexity and costs are high and so is the inconsistency. It's as if the forecasters have to tune their models for each major disturbance, resulting in periods with decidedly mixed forecasting accuracy. For examples of this phenomenon, just consider forecasts of the economy and the stock markets.
The Early Warning & Long-Term Forecasting Conundrum
Forecasters are left with a conundrum. It's often best to use short-sighted, but reasonably accurate univariate methods, though they break down in the face of some systemic changes. It becomes a challenge to know when to change methods and what forecast methods to use when profound outliers and discrepancies start to appear.
It's important to remember, however, that univariate models tend to mask or minimize discrepancies. By themselves, they form relatively poor early-warning or long-term forecasting systems. Whole tomes have been written about long-term forecasting, but there are practical rules of thumb to follow when setting up an early warning system (EWS). The following are all necessary, but by themselves do not guarantee a good EWS:
Have a simply stated reason for the success of a product or product line;
Test that with scorecarding and simple forecasts on a monthly or quarterly basis;
Track unexpected, large increases and decreases or variances over time in performance;
Try to incorporate major events into your success model;
Be prepared to look at good news as well as bad news;
Keep the analysis simple until the implications and risks demand something better than simple analysis.
Many automated systems make it possible to analyze a much smaller set of variables for early warning screening. Other methods provide statistical tests. However, early warning methods are state-of-the-art, and there are a number of techniques available for analyzing data.
The field of predictive analytics and early warning systems has certainly garnered attention as techniques developed in diverse areas such as anti-terrorism, criminology, earthquake prediction, econometrics, stock market analysis and weather forecasting start to converge on practical methods for discovering time series anomalies and changes of state. However, nothing beats a solid causal model, which means understanding the behavior of a system. Short of that, a whole range of techniques from neural networks through state space systems to econometric modeling is being used to develop proxy models of behavior of systems.
By following progress in the arena of early warning systems, one can see the difficulties involved in predictions and forecasting in general. Analysts lacking a deterministic or causal model often use proxies and approximations to analyze and predict the behavior of complex systems or their departures from the expected. The author Santayana warned that ignoring history means we are condemned to repeat it. Likewise, by predicting the future with ever greater certitude, we inevitably change it. But the rewards of doing even that can be very compelling.
Jacques Surveyer is a consultant and trainer; see some of his tips and tutorials at theOpenSourcery.com.
Glossary:
Cluster analysis: a collection of statistical techniques for partitioning data into homogeneous groups or cases.
Combined analysis: methodology used by SAS for forecasting which combines several methods to arrive at single forecast.
Econometrics: the application of mathematics and statistics to the study of economic and financial data.
Heteroscedasticity: the condition of unequal variance in the data.
Least Error Predictor: the forecasting method which, when using the available actual data, generates a series of forecasts with the lowest cumulative error often measured as MAD, or Mean Absolute Deviation.
Markov series: a simple stochastic process in which the distribution of future states depends only on the present state and not on how it arrived in the present state.
Neural network: analysis in which inputs are connected in a manner suggestive of connections between neurons; but then strength of the connections are revised by trial and error analysis of the data.
Nonparametric statistics: the branch of statistics dealing with variables without making assumptions about the form or the parameters of their distribution.
VARMAX: one of a range of methods that extend Auto Regressive Moving Average methods to include exogenous variables. VARMAX has spawned other multivariable forecasting methods such as GARCH, or Generalized Autoregressive Conditional Heteroscedasticity, which are sometimes packaged together because of similar use and solution methods.
State Space: a modeling method derived from mathematical system dynamics.
Stochastic: being or having a random or heretofore unpredictable component.
Further Reference:
Practical Business Forecasting, by Michael Evans - A look at forecasting from a top-down point of view.
Forecasting Methods and Applications, 3rd edition, looks into forecasting methods carefully.
AutoBox - a free tutorial website that gives the rationale behind forecasting in general, their methods in details.
JustEnough Software - Automated demand forecasting
SAS Institute - Large-scale, automated batch-forecasting system
Autobox - Leading univariate and EWS software supplier
Forecast Pro - Well-rated range of forecasting technologies
About the Author
You May Also Like