Feasting on High-Quality AI DataFeasting on High-Quality AI Data
AI hungers for quality data. Learn how you can feed it properly.
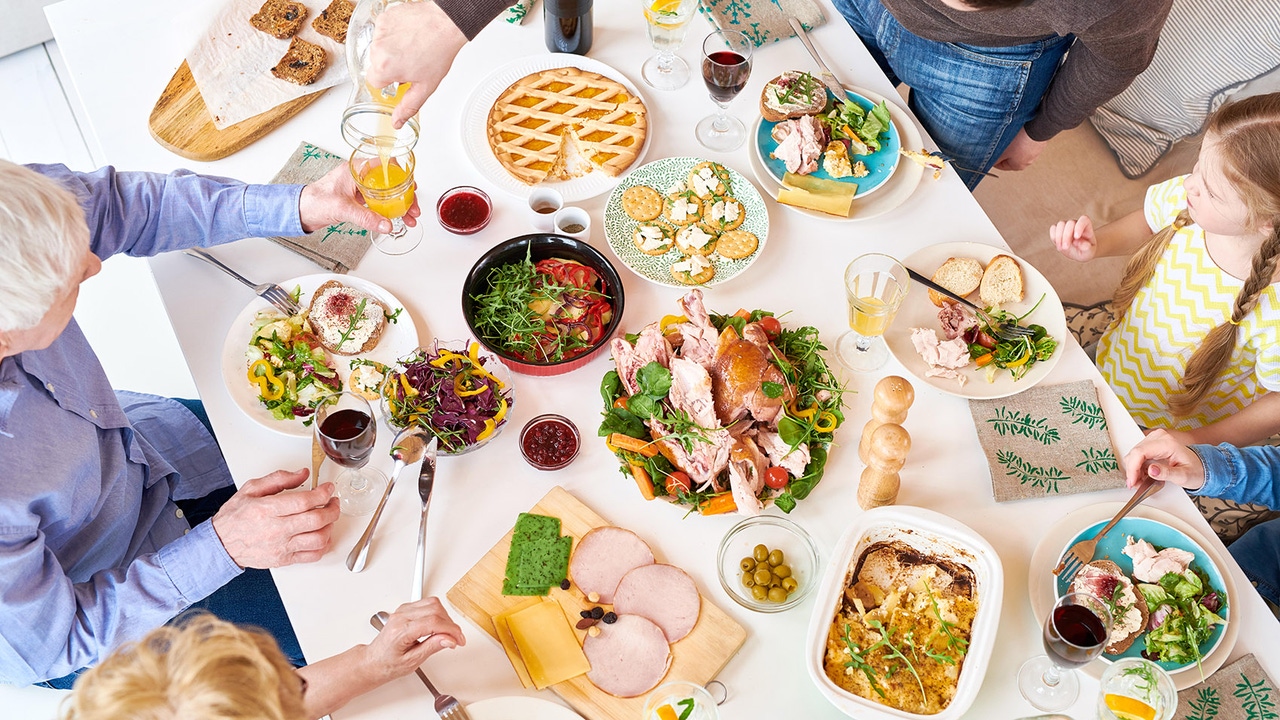
At a Glance
- Avoiding poor quality data can boost AI models.
- The quality of data impacts the integrity, quality, and reliability of AI systems.
- Rigorous data governance leads to higher quality AI data in the long term.
Garbage-in, garbage out is concept that dates back to the earliest days of software development. Yet flawed, poor quality data is also a threat that AI developers need to watch out for. If the data used to train an AI model is incomplete, inaccurate, inconsistent, or biased, its predictions and decisions will be flawed and most likely useless.
Maintaining data quality to feed AI systems is like nurturing a garden, says Noelle Russell, data and AI lead with Accenture Federal Services, in an email interview. “It requires diligence, intentional care, and a deep understanding of the ecosystem.”
Ensuring data quality requires a multi-faceted approach. “This includes establishing robust data governance frameworks, implementing comprehensive data validation and cleaning processes, and fostering a culture of data literacy within the organization,” Russell advises. By treating data as a valuable asset, organizations can ensure that their AI systems are fed with high-quality, relevant, and unbiased data.
It’s essential to approach data quality through an empathetic lens, Russell says. “This empowers data workers to see data in new ways and ask better questions about how the intended solution can serve more people.”
Successful AI, particularly generative AI initiatives, require clean, well-organized, and accessible data. “Building AI on messy data is like building a shiny new rocket ship that you intend take to Mars, but you don’t have the fuel to even get it off the launchpad,” says Wendy Collins, chief AI officer at technology service and consulting firm NTT Data via email.
Collins stresses the importance of paying the closest attention to the data that’s most important. “No organization is ever going achieve the pinnacle of perfection when it comes to data quality, so focus on what matters most and start there.”
Multiple Benefits
Feeding AI quality data leads to multiple benefits. “It enhances the accuracy and reliability of AI predictions and decisions,” says Ed Marshall, CTO at technology consulting firm Hedgehog Lab, via email. Quality data also ensures that AI models are trained on accurate, comprehensive, and relevant datasets, leading to more effective outcomes. Perhaps most important, quality data reduces the risk of AI biases, which can result from incomplete or skewed data. “High-quality data can [also] improve the efficiency of AI systems by reducing the need for constant retraining and adjustments.”
Data excellence significantly improves the accuracy and reliability of AI predictions and decisions, leading to better business outcomes. “It also helps in building trust in AI systems among users and stakeholders by ensuring transparency and fairness in AI operations,” Russell says. “Quality data also reduces the risk of AI biases, which is critical for ethical AI practices.”
Collins recommends focusing near-term resources on the most valuable data elements. “We don’t believe in having a big bang approach to AI where you build and build and then the magic happens three years later,” she says. “Our philosophy is to incrementally build in value creation opportunities along the way.”
Doing it Right
IT leaders often take the wrong approach to AI data quality. “One common mistake is underestimating the importance of diverse and representative datasets,” Russell says. “This can lead to biased AI models that don’t perform well across various scenarios or different groups of people.” Additionally, many leaders underestimate the resources needed to build models that can scale responsibly. Russell recommends investing in well-tested and robust data pipelines, and to focus on input data model standardization as well as comprehensive data validation to ensure that the data being fed into the generative model is high quality.
The best way to maintain long-term AI data quality is by establishing a rigorous data governance framework, Marshall advises. This requires creating strict protocols for data collection, processing, and management. “The effectiveness of this approach lies in its ability to ensure that the data feeding into AI systems is accurate, consistent, and representative,” he explains. “By maintaining high-quality data, you reduce the risk of biases, errors, and anomalies in AI outputs, which is crucial for the reliability of AI-driven decisions.”
IT leaders often underestimate the importance of ensuring ongoing data quality management. “There’s a common misconception that once an AI system is trained and deployed, the focus can shift away from data quality,” Marshall states. That can be a big mistake, since AI systems are dynamic and require continuous feeding with high-quality data to maintain accuracy and relevance. “Neglecting this fact can lead to degraded AI performance over time and a failure to adapt to new patterns or changes in the operational environment.”
A Final Thought
Given the rapid pace of AI advancement, Russell believes that today’s IT leaders must continue to learn, not only through books and courses, but also via hands-on experiences. “Now is the time to embrace intellectual curiosity and empower those in every part of your organization to do the same.”
About the Author
You May Also Like