Four Basic Steps to Prevent Your Data Lake from Becoming a SwampFour Basic Steps to Prevent Your Data Lake from Becoming a Swamp
Despite their great promise, data lakes have received a lot of negative buzz in recent years due to their lack of governability and general success.
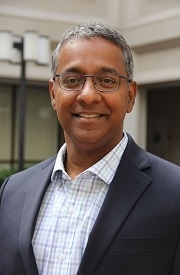
Business and technology leaders have been expecting game-changing insights from data lakes, only to be let down. But with the availability of cloud, it's easy to store much more data as you would in creating a data lake. Now, the fundamental challenge remains: How can a data lake be used to drive more analytics use cases that drive business decisions?
As technical complexity becomes less of a barrier, organizations still need to clean up some common mistakes that are not technical in nature. Here are four steps your subject matter experts and line of business folks can take to make sure your data lakes remain healthy:
1. Start with data you know you're going to use for a specific project
Although data lakes can hold an unfathomable amount of data, they’ve historically failed because of a lack of pre-planning. Instead of building their data lakes in accordance with specific needs, organizations were haphazardly dumping data into them. And while the point of a data lake is to eventually have all or almost all of your company’s data in it to enable a wide variety of analytics, you have to balance that with your need to prove the value of the data lake to your business.
2. Load data once and only once
There are two challenges you have to deal with when loading data into a data lake. The first is managing big data file systems requires loading an entire file at a time. For small tables this isn’t a big deal, but this gets more cumbersome when working with large tables and files. You can minimize the time it takes to load large source data sets by first loading the entire data set once and then subsequently loading only the incremental changes. This requires identifying just the source data rows that have changed and subsequently merging and synching those changes with existing tables in the data lake.
Organizations are running into another related challenge. When two different people load the same data source into different parts of the data lake, the DBAs responsible for the upstream data sources getting loaded into the lake will complain that the data lake is consuming too much of their capacity to load data. As a result, the data lake gets a bad reputation for interrupting operational databases that are used to run the business. You will need strong governance processes to ensure this doesn't happen (see step #4 below).
3. Catalog your data on ingest so it is searchable and findable
This next point is somewhat related in that when you do bring data into the lake, you need to make it easy for your analysts to find it. This same capability can be used to eliminate the accidental loading of the same data source more than once.
Thinking that you will load your data into the lake and some day in the future you will come back and catalog it all is a big mistake. While this is possible, why dig a hole for yourself right out of the gate? By simply implementing good data governance processes up front you can make it much easier to use your data lake and demonstrate value to your business sponsors, while also eliminating the multi-loading problem mentioned above.
4. Document your data lineage and implement good governance processes
Once people start using data in your data lake, they might clean it or integrate it with other data sets. Quite often it turns out that someone else has implemented a project that will have already cleansed the data that you are interested in. But if you only know about the raw data in your data lake, and not how others are using it, you are likely to redo work that has already been done. Avoid this problem by documenting data lineage thoroughly and implementing solid governance processes that illuminate the actions people took to ingest and transform data as it enters and moves through your data lake.
There are many other considerations that go into constructing a properly operationalized and governed data lake that aren’t covered here. However, these points provide a start if you want to have a data lake that works and provides value for your organization -- vs. a data lake that becomes a swamp.
Ramesh Menon is vice president of products at Infoworks. Menon has over 20 years of experience building enterprise analytics and data management products.
About the Author
You May Also Like