Have a Failing Big Data Project? Try a Dose of AIHave a Failing Big Data Project? Try a Dose of AI
A growing number of AI experts are turning their attention to big data. Will that be enough to save doomed projects?
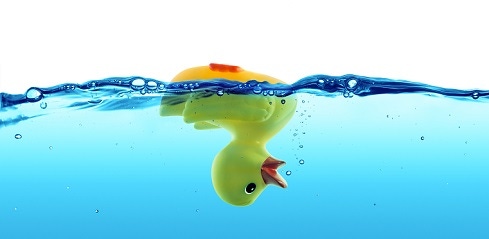
Back in late 2017, Gartner analyst Nick Heudecker estimated that the failure rate of big data projects was 85%. Move the calendar forward two years and there's no solid evidence proving that the failure rate has improved in any meaningful way.
But help may be on the way. A growing number of artificial intelligence experts are arriving at the conclusion that the technology has the potential to turn big data failures into resounding success stories. The trick lies in knowing how to use AI correctly.
Keys to success
Chris Heineken, CEO and co-founder of AI consulting firm Atrium, is optimistic that AI and machine language (ML) will emerge as the keys to big data project success. "Big data is all about making sense of massive amounts of structured/unstructured data and generally lays the foundation for [successful] predictive analytics," he explained.
When used properly in a big data project's early stages, ML algorithms can also help identify the viability of answering key questions, such as how to improve lead conversion. "ML can also be deployed to identify whether the existing data architecture can support big data program objectives and, if not, help identify gaps in the data that need to be addressed." Heineken added.
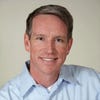
Chris Heineken
AI should be applied to a big data project whenever standard predictive analytics incorporates too many variables, making models cumbersome to optimize and slow to run, observed Ken Elefant, a managing director at venture capital firm Sorenson Ventures. Additionally, when an enterprise is in the process of transforming its business model, such as when moving from a brick and mortar environment to a hybrid setting, opening the gates to a flood of new data, constant remodeling is required. "An AI approach to data analytics provides much more flexibility," Elefant noted.
Complicating many big data projects is the common need to combine structured data with unstructured data. "Instead of using a structured, rules-based approach, artificial intelligence as part of a big data project treats data much like a human would, by predicting how new data is affecting past trends" Elefant explained.
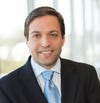
Ken Elefant
Bill Schmarzo, CTO for IoT and analytics, at data storage systems provider Hitachi Vantara warned that AI, while useful, can't by itself prevent big data projects from failing. To ensure that a project won't founder, organizations need to use cross-organizational collaboration to identify, validate, value, and prioritize big data-supportable use cases, he said. "Gaining business stakeholder buy-in on the targeted use cases is the key to driving out the passive-aggressive behaviors that dooms most IT-centric projects."
Any organization that wants to rely on AI to prevent future project failures needs to carefully consider why their past big data project failed. "This includes excellent hygiene around project post mortems and retrospective analysis on failed projects," observed Andrea Gallego, a CTO and principal at management consulting firm Boston Consulting Group. Equally important is understanding why big data projects succeed, so that AI algorithms can be designed to understand both sides of the coin, she noted. "This makes it easier to identify when a project does not have these qualifying factors."
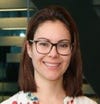
Andrea Gallego
Getting started
AI is a broad category that can include supervised and unsupervised machine learning, neural networks and reinforcement learning. "The key to knowing which of these tools to use is predicated on a detailed understanding of the problem you are trying to solve and the types of data -- structured, semi-structured, unstructured -- with which one has to work," Schmarzo explained. A good data scientist, he noted, is like a skilled carpenter in that both will use the best combinations of tools to solve the problem at hand.
AI may not be new, but AI at scale within complex organizations is still in its early stages. "We still do not yet understand every consequence of integrating AI into larger systems," Gallego said. "Organizations should be ready to take on this risk and should be mature enough to understand the consequences and tradeoffs."
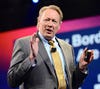
Bill Schmarzo
Heineken noted that all big data projects, regardless of the approach used, have three basic failure points: understanding the question that needs to be answered, the data architecture and its availability, and having the ability to land insights into a business workflow at scale. Effectively addressing these issues "are all critical success factors," he advised.
Takeaway
AI is mainstream to the point that it's hard to separate it from a big data project, Heineken said. "AI should be fused into all the phases of a big data project and should be used as a set of techniques/technologies that inform the design and provide the delivery mechanism for the value to be realized through big data."
For more on artificial intelligence and big data, check out these recent articles.
Beat the Odds: How to Conquer Common AI Challenges
Outlook is Good for Health Care, Thanks to Big Data
AI Ethics Guidelines Every CIO Should Read
Enterprise Guide to Digital Transformation
About the Author
You May Also Like