How to Create a Successful AI ProgramHow to Create a Successful AI Program
Artificial intelligence can be promising and valuable, but you need to focus on a few best practices to get your program moving in the right direction.
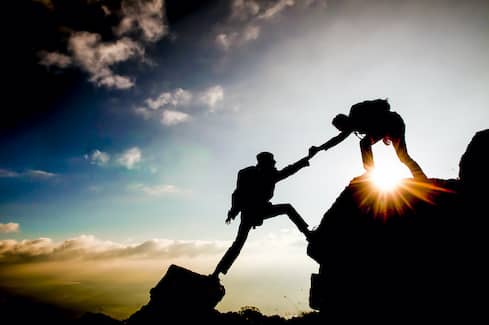
Lots of people are interested in artificial intelligence, and there are plenty of stories about the amazing strides that are being enabled by AI, in business, in medicine, and in any number of other areas. But the stories of wild successes hide a very different part of the AI process -- all the hard work that comes before that success.
Justin Nguyen, director of data science and AI and Sense Corp., likens those AI successes to snapshot photos he has taken at the summits of his favorite hikes in some of the world's most beautiful places. One photo shows an amazing view capturing a blue sky with puffy white clouds, miles of ocean coastline and then an entire forest, and hills of rocky outcroppings. It's spectacular.
"You can see that it was beautiful at the top," he said. "But there was a lot of work that went into this." Nguyen shared these thoughts during his Interop Digital session: Reaching the AI Summit: The Definitive Playbook for Setting Up Your AI Organization. AI is similar to that spectacular summit snapshot, he said. There's a lot of effort required, and many obstacles to overcome, but you don't hear so much about those.
For more on AI today, read these:
Analytics Salaries Steady Amid COVID Crisis
Data Science: How the Pandemic Has Affected 10 Popular Jobs
The State of Chatbots: Pandemic Edition
ITSM Platforms Gain Artificial Intelligence
"Instead you hear about all the wild success in how AI has been deployed and implemented in all these different industries," he said. "It's all the hype, all about the excitement. Everyone wants to do it. Everyone is talking about it. But you don't hear about everything it took to get there. You only see that snapshot of success."
But in fact, Nguyen pointed out, most data science projects fail. Almost 9 out of 10 never make it into production. There are three major reasons why projects fail.
First, there's a data problem. Data is the foundation of any successful AI program, and not every organization has put a priority on data quality, master data management and data governance throughout the organization. As a result, the data may be inaccurate, siloed, and slow.
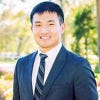
Justin Nguyen
Second, the business may not be ready. Organizations need to establish a clear understanding of requirements and capabilities needed for data science initiatives, Nguyen said. That includes conducting thorough due diligence to define achievable use cases.
Third, organizations need to make sure their tech stack is up to date, and their data pipelines and processes are scalable. That's what's required to truly operationalize AI projects in an organization.
"The biggest mistake I see companies make early on is around picking the right use cases," Nguyen said. "Picking your early use cases is extremely important because it sets you up for success down the road."
A good first use case is one where the value of the project can be measured in terms of dollars saved, dollars made, or some other similar metric. Nguyen also recommends that this first use case sets you up for a quick win.
"Your early win doesn't just deliver that value, but it also gives you a tangible and compelling narrative," said Nguyen. "It aligns the organization toward a vision. It builds excitement. It creates that momentum you need to keep going and to drive all these big technology and big process changes."
Next comes planning, and Nguyen said that this is where many organizations trip. First you need to hold a brainstorming session with leaders from across the organization to look at possibilities and pain points. Then you prioritize based on business value and technical feasibility. That means looking at the data you have and whether it's accessible and accurate, examining the technology you have in place to do the work, and taking stock of the skills and capabilities of your organization.
It seems like a lot of work, but don't let that slow you down.
"Begin early, be agile, and start small," Nguyen said. "AI starts today. Don't wait until everything is perfect and the stars are aligned before you begin. Start now and build with incremental wins."
He recommends taking this approach because AI is about progression, not perfection. You will learn and progress and improve over time.
"The earlier you start, the more cycles for improvement you have," Nguyen said.
Now is a great time to start, because more people are excited about artificial intelligence all across businesses, regardless of department.
"The fact is there's a shift happening right now where many people are starting to really become interested in AI," Nguyen said. "They enjoy talking and hearing about AI."
That's something that you can leverage to further the cause within your organization. Nguyen recommends regularly and repetitively evangelizing, educating, and empowering business users. When you do that stakeholders appreciate the AI development efforts and they also start appreciating the importance of data quality, capture, and accessibility.
"AI is a team sport," Nguyen said. "Engage and empower the rest of your business. Take others with you on your journey because you'll go farther than you could alone."
About the Author
You May Also Like