How to Elevate Your Organization’s Use of Data AnalyticsHow to Elevate Your Organization’s Use of Data Analytics
Here are three best practices for leveling up your organization’s use of analytics and attaining ROI with an enterprise analytics program.
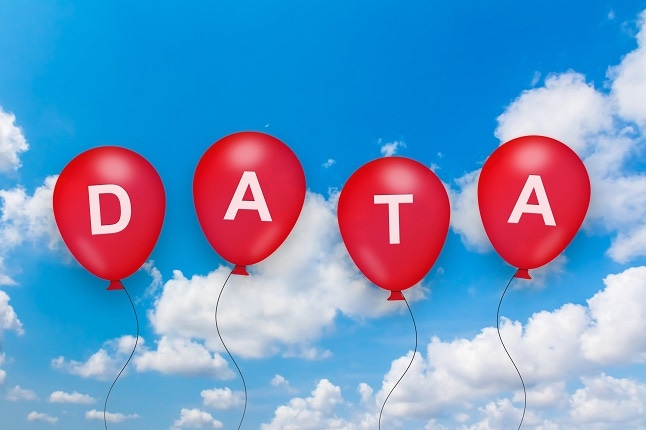
Enterprises from every industry and at every scale are working to leverage data to achieve their strategic objectives -- whether those are to become more profitable, effective, risk tolerant, prepared, sustainable, and/or adaptable in an ever-changing world. An enterprise’s analytics must grow at pace with the business and its needs to achieve agility and resilience. Otherwise, it will be hamstrung or tripped up.
Often the technical debt accumulated from years of workarounds and gap-fixing existing processes seems too expensive and challenging to rip and replace with more capable modern tools and processes. However, the demand for mature, modern data analytics is becoming too strong to ignore.
Here are the three best practices for leveling up your organization’s use of analytics and attaining ROI with an enterprise analytics program:
1. Get all data under management
Enterprise data, the foundation for analytics, is arguably only useful to very narrow interests if it is not managed to a standard. I’ve found that the efforts around enterprise data not managed to a standard I’ll call “under management” is rarely providing ROI.
Under management means the data is in a leverageable platform. The platform was built, or is now, designed for wide access, or at least it interfaces with a platform that is. This means the data is built mindful of the data warehouse(s), the data lake(s), the operational hub(s) and the master data management hub(s). There are a few finite reasons why the data for an application may not be wholly in one of these structures, having to do with security or specific data transformations desired by the application, but the leverageable platform should be the first option. All enterprise data elements should be in a leverageable platform somewhere so make sure you are not building the one data store for elements that are not leverageable elsewhere.
Beyond leverage, the data should be in an appropriate platform for its profile and usage. Post-operational analytics data should be in columnar, analytics databases with high non-functionals (availability, performance, scalability, stability, durability, secure), captured at the most granular level, at a data quality standard (as defined by data governance) and enabled for self-service.
Having all enterprise data, as well as all applicable third-party data, under management at a granular level may seem like overkill, but it underscores the importance of data to company initiatives, drivers, and strategies. Every element is undoubtedly valuable. If that is not apparent, there should be activities concurrent with the management activities with the goal of demonstrating the importance of data and how that data can be made available to business interests.
2. Big data tooling for big data
In years past, we tried to force-feed growing unstructured big data into relational data warehouses with high cost and limited success. Now that the competitive horizon is firmly focused on analytics with big data, with the expectation that the other data is already in good shape, using the right tools for this data is important. There is a different class of tooling that is required. These tools primarily focus on the ingestion problem with big data.
This starts with the data platform for big data, which is primarily cloud storage, and we refer to a repository there as a data lake. The data lake is common and centralized storage for the enterprise. There is no defined data model into which the data is formed so all data can land there. A data lake is primarily for analytic data, and it can retain data for history very well. Today, data scientists are a primary user group for the lake, but over time, this is changing to include the analyst community.
3. Manage the change to an analytics culture
To get the most ROI out of an analytics program, technology roles need to be disintermediated from between the data architecture and the users. The users need to be empowered with self-service data access, capturing their own insights rather than requesting insights. The interactivity with data, in the limited window users have, will be greatly enhanced by a self-service approach. However, not all users want the change this may represent.
Managing the change to using self-service and using analytics for all business functions requires change management. Despite any direction from the executives, users will be on a gamut from embracing change to refusing to change. Late adopters mostly just need some space and time. They need examples of peers excelling with analytics. They need reinforcement of any data- or analytics-driven cultural direction. Using self-service analytics is part of raising the foundation of the company today and is required and inevitable.
Getting all data under management, including big data in big data tooling, and managing the change to an analytics culture are the best ways to level up your organization’s use of analytics and attain ROI with an enterprise analytics program.
About the Author
You May Also Like