IoT and Predictive Analytics: What We’re Driving TowardIoT and Predictive Analytics: What We’re Driving Toward
Learn how research of predictive analytics can impact models used in the transportation sector.
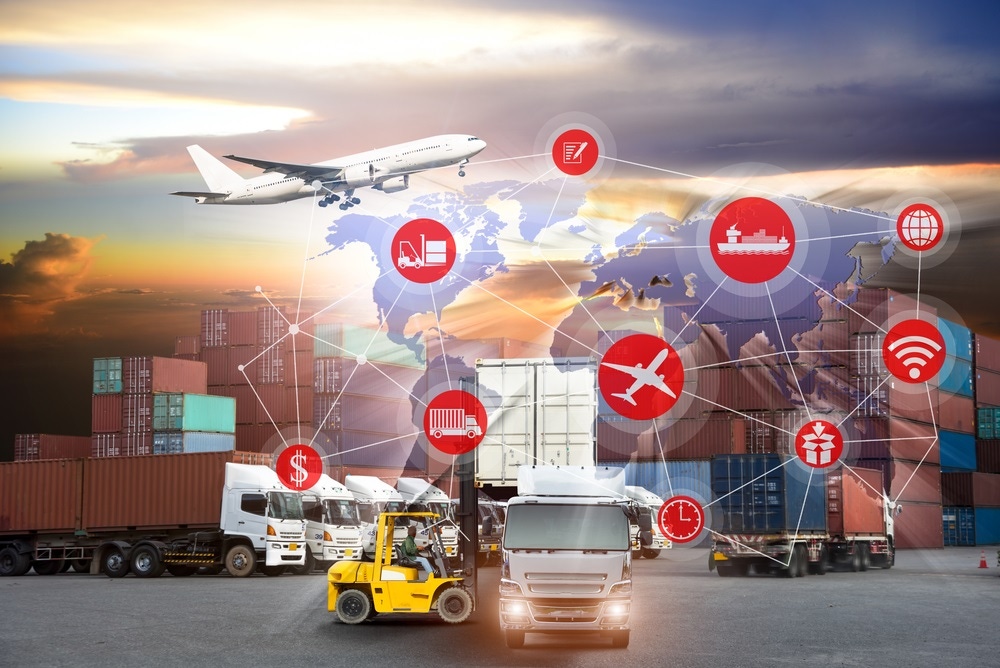
When you hear the phrase "Internet of Things”, you may picture smart watches, smart speakers, and home appliances of every purpose. Some companies, however, imagine the logistics of their own things -- transportation fleets -- and how to best manage them with their distribution operations. Predictive analytics plays a central role in deriving value from the real-time data of IoT devices. With vehicles being networked, what opportunities are businesses driving toward for strategic advantage?
One prominent example comes from Amazon. It announced last fall that it is expanding its Amazon Air program to include 50 cargo jets and a new $1.2 billion distribution hub in Kentucky. Stock market analysts had been anticipating Amazon’s entry into transportation and logistics. CNBC reported that Amazon even named the category for the first time in its stockholder report. As a result of their strategies, Amazon has announced one-day shipping for its Prime customers.
Amazon’s forays present more than an opportunity to usher the retail market into primary one-day delivery. Applying predictive analytics and IoT strategies to fleets will transform transportation-dependent companies into a profit laboratory for examining how logistics can serve customers through better real-time metrics and data-influenced decisions to deploy resources. Successful trials will improve margins, from more accurate shipping information for clients to more efficient purchase of fuel, maintenance parts, and essential support services.
Many industries have come to rely on data for driving an analytics value chain that leads to a competitive business advantage. Those with an exposure to transportation, however, have particularly exciting opportunities in data model exploration because more sophisticated analysis techniques can rely on meaningful real world data. The end result is superior assessment of value derived from operations.
Exploring advanced models such as logistic expressions and Markov’s Chain can reveal relationships through classifying data. Logistic regressions yield a binary solution based on a weighted scale of the observations, while a Markov Chain progressively classifies observations based on a series of probability conditions.
The challenge that analysts generally face is to create a cohesive narrative from data that indicates which relationships will consistently support good business decisions or improve features of a product or service.
One way predictive analytics is changing transportation is in how it is forcing firms to evaluate how they arrange data sourced from electronic logs, video event recorders, electronic control modules, and other vehicle sensors. Organizing these sources is critical for triaging which transportation challenges to solve and means finding relationships among the data that can be made into useful experiences.
For an automotive example, think of a Corvette. Specialty versions of the vehicle offer a Performance Data Recorder that enables telemetry overlays of vehicle data on video from a high-definition camera.That data, sourced from various system activities, is used to analyze driver sessions on a race track, enhancing a customer wish (a racing experience from their car).
Exploring data organization will rise as autonomous vehicle fleets become more prominent on public roads. Vehicles have historically managed this data in one format or another, but until now there were no opportunities to consider data from a network, moreover with consideration of a central repository or local platform to host data. Autonomous vehicles generate real-time data creation, which can inform managers with real-time logistic decisions.
But tons of data, each source using a very different structure type, are generated. Autonomous vehicles generally rely on three sources for data: object detection sensors, LIDAR to identify lane markings, and cameras to recognize signs, people and objects. Data exploration can lead into extensive data cleaning to identify null values and determine if sensor glitches are appearing among the observations. All of this can derail model development early in the process.
Thus applying predictive analytics to different data types and sources calls for managing multiple stakeholders and establishing a manageable data ecosystem. The race in predictive analytics is not so much gaining an analysis -- there are literally thousands of tools on top of the three major cloud solutions. The race today is about how to best manage data sources, machine learning tools, and collaboration among teams.
The rise of predictive analytics and machine learning trends in transportation also spotlights questions regarding where data should be best stored for analysis. Which data is best accessed from a centralized location or onboard the vehicle? A shift in when and where analysis happens also occurs. For analytics, it is no longer a question of “if” in-house data-driven cost controls are needed; it’s a question of where the information associated with cost-control metrics should be warehoused for the quickest queries.
Security and social issues
Other questions will arise as smart devices arrive on the market. IDC stated in hosted event, FutureScape for Internet of Things, that by 2020 40% of IoT created data will be stored “at the edge.” In other words, in or near a given device. Decisions on data storage location dictate strategic choices as to where data security should be emphasized and what kind of data quality exists in a transportation network and its analytics ecosystem.
The demand for an ecosystem will also spur researchers to examine societal transportation issues related to infrastructure, such as how communities can best manage traffic flow against pollution and quality of life concerns. With open data available in portals and machine learning solutions being more widely available online, researchers have the means to create an analytics ecosystem where teams can collaborate and be transparent in their findings and debates.
Businesses are realizing that their future growth will depend on harnessing data from their assets and the analysis of network activities. The stock market speculation surrounding Uber’s recent IPO illustrates that having access to data is no profit guarantee. Some stock analysts see Uber as the next Amazon due to its data access and its capability to offer other services beyond car sharing. Others decry that its considerable debt offsets any potential to leverage its data into profits. Even more detrimental, Uber delayed its autonomous car program after an infamous auto accident that killed an Arizona pedestrian in 2018. Application of data does not guarantee safety, let alone a successful business model.
Firms influenced by the digital transformation of transportation can find themselves cruising on a financial easy street if they develop the best data model ecosystem that explores operational gains while serving customers for the long haul.
About the Author
You May Also Like