Is BI The Key To Personalized Medicine?Is BI The Key To Personalized Medicine?
The right business intelligence tools can help clinicians zero in on each individual's healthcare needs, but there are important caveats to bear in mind.
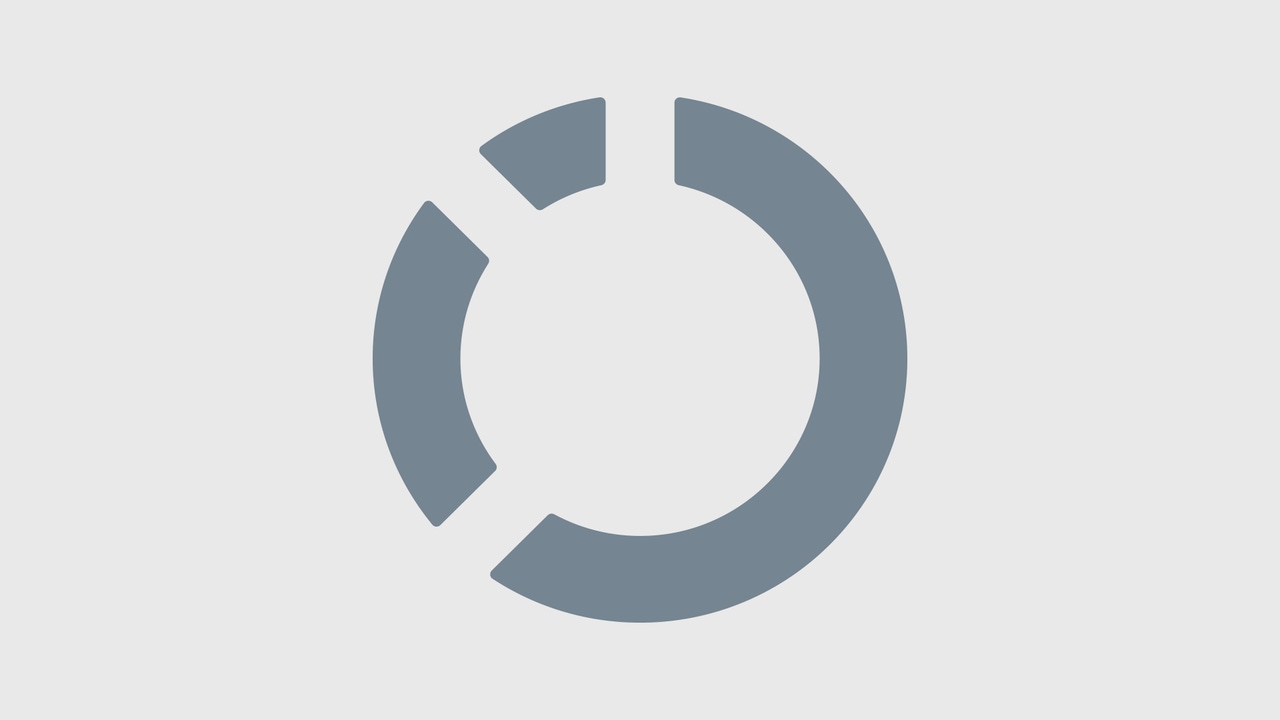
For as long as I can remember, individualized therapy has been the Holy Grail of clinical medicine. But given the complexity of human disease, "The Impossible Dream" might be a more fitting metaphor. That's the case because it's been so difficult to get the clinical analytics systems needed to individualize treatment to communicate with many electronic health record (EHR) systems.
Consider two contrasting examples. Lyme disease is a fairly straightforward condition to understand and treat. It's caused by the bite of a tick that has been infected with a microorganism called Borrelia burgdorferi. If it's caught early on, it can usually be cured with the right antibiotic. One cause, one cure, and rarely any need for a personalized regimen.
Coronary artery disease (CAD), on the other hand, is much more complicated. Among the contributing causes are excessive intake of saturated fat, elevated serum insulin levels, obesity, hypertension, diabetes, hypothyroidism, a sedentary lifestyle, a history of smoking, and genetic mutations. And the degree to which each of these factors contributes to CAD varies widely from patient to patient.
The problem is figuring out what percentage of each patient's disease is caused by each causative agent. For Mrs. Smith, 10% of the disorder may be diet-induced, 50% genetic, 20% lack of exercise, and 20% low thyroid hormone levels. For Mr. Jones, the percentages may be quite different. Thus the need for personalized medicine, which ideally would evaluate all the variables, case by case, and come up with a plan to treat them.
Can IT tools do the statistical gymnastics needed to figure out how to manage individual patients with such multi-factorial diseases? A team of computer experts from Archimedes, a healthcare modeling organization in San Francisco, may be headed in that direction.
Individualized Clinical Guidelines
In a study published in the May 2 issue of Annals of Internal Medicine, researchers from Archimedes report on an electronic "cardiovascular guidelines calculator" they developed. They used it to estimate the likelihood of a patient developing a myocardial infarction or stroke, based on an individualized risk profile. The calculator tapped into the EHRs of more 2,700 people enrolled in the long-term Atherosclerosis Risk in Communities study and extracted data on their age, sex, blood pressure, family history, presence of diabetes, and smoking status.
The software then generated individualized treatment guidelines and compared them to the generic guidelines provided by the Joint National Committee on Prevention, Detection, Evaluation, and Treatment of Hypertension (the JNC 7). The goal was to see which set of guidelines has the most potential to improve clinical outcomes and reduce costs.
They found that the individualized EHR-assisted guidelines could prevent 43% more myocardial infarctions and strokes "for the same cost as treatment according to the JNC 7 guidelines."
Douglas Owens, MD, professor of medicine at Stanford University, praised their findings but also offered an important caveat, saying that: "... making tailored guidelines easy to use is crucial. If clinicians have to find or enter data, they are unlikely to use individualized guidelines." In other words, EHR integration is the key to making this EHR/business intelligence partnership work. Mass. General And Personalized Medicine Massachusetts General Hospital researchers have also been trying to marry clinical analytics tools to EHRs in the hope that it will individualize patient care. They started by looking at a database of patients being treated at 12 outpatient practices in eastern Massachusetts, practices that all share the same EHR system. Mining the patient data for clusters of risk factors for diabetes and cardiovascular disease (CVD), they identified patients with central obesity (a concentration of fat in the abdomen); elevated blood pressure, plasma glucose, and triglycerides; and low HDL cholesterol. Their analysis revealed thousands of patients without full-blown diabetes that had at least three risk factors for metabolic syndrome, a precursor for diabetes and CVD. Those in the highest risk group were much more likely to develop full-blown diabetes within three years (11%), when compared to patients with no risk factors (2.4%). Put another way, the EHR system was able to use clusters of risk factors to identify patients at more risk of developing diabetes, and thus in greatest need of individualized preventive therapy. While results like these bring us closer to the dream of personalized medicine, two important caveats apply here. Both these studies were able to identify only a few of the risk factors that make a person susceptible to disease. They ignored genetic predisposition and diet, among other things. And just as important, given the fact that the average doctor in private practice spends about eight minutes with each patient, if your EHR doesn't play nicely with your clinical analytics program, doctors just aren't going to take the time. Recommended Reading: Can IT Really Nail A Complex Medical Diagnosis? Will Social Media Tools Transform Clinical Research? Three Mobile Medical Apps That Turned My Head Medical Data Mining Strengthens Drug Safety GE Healthcare Taps SAS For Patient Safety Analytics Patient Safety Initiative To Leverage Health IT See more by Paul Cerrato
The Healthcare IT Leadership Forum is a day-long venue where senior IT leaders in healthcare come together to discuss how they're using technology to improve clinical care. Find out more.
About the Author
You May Also Like