Lessons from the Netflix Prize competitionLessons from the Netflix Prize competition
The $1,000,000 Netflix Prize competition has produced interesting results, even if no winner, 15 months in. Some of those results are a bit surprising; others we should have expected but didn't anticipate. So while participants haven't yet bettered the accuracy of Netflix' Cinematch recommendation algorithm by 10%, the threshold to win the $1 million prize, we can still take away lessons about predictive-analytics fundamentals.
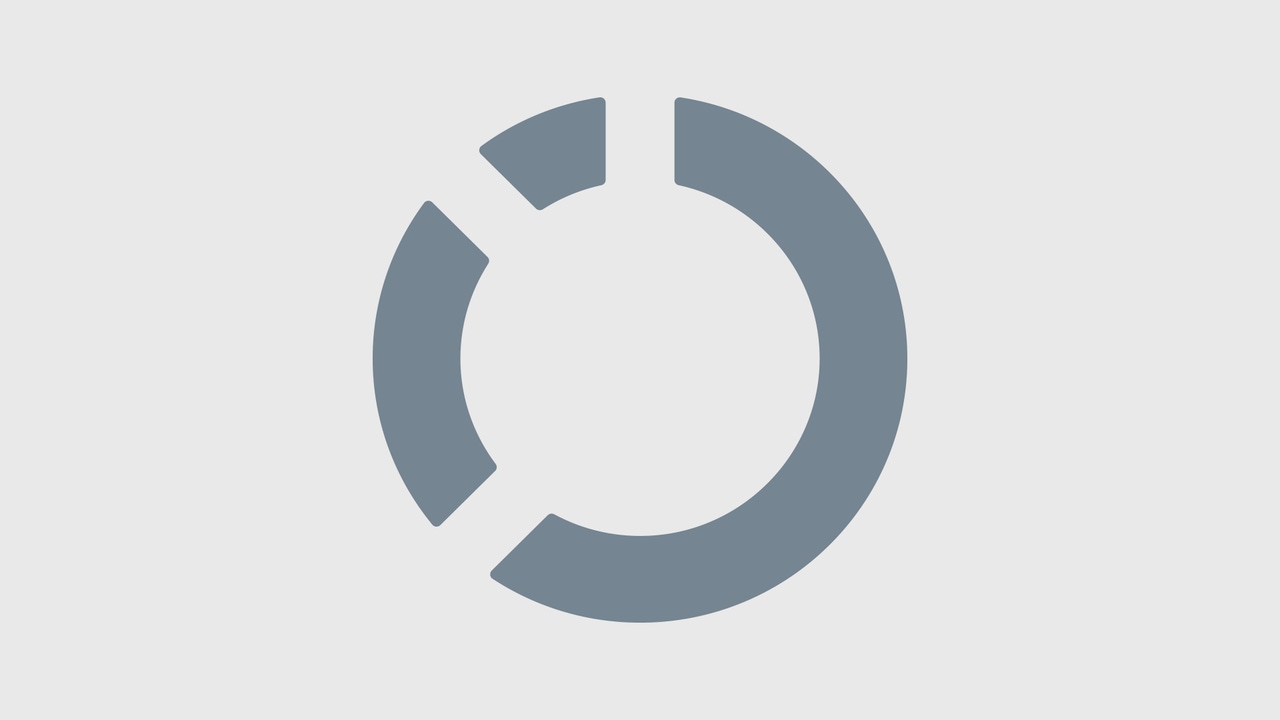
The $1,000,000 Netflix Prize competition has produced interesting results, even if no winner, 15 months in. Some of those results are a bit surprising; others we should have expected but didn't anticipate. So while participants haven't yet bettered the accuracy of Netflix' Cinematch recommendation algorithm by 10%, the threshold to win the $1 million prize, we can still take away lessons about predictive-analytics fundamentals.
I recently checked on competition status after receiving a note from Alex Lupu, VP Marketing USA for Scio Systems; Alex has been keeping me apprised of his company's progress toward launch of property-lease abstracting and analysis tools. Like Alex I'm into text analytics, and I liked his take that "intelligent communication between customer and the [Netflix suggestion] system" could provide an alternative route to better recommendations. Alex sees analysis of "'open questions' that allow customer to write a sentence or two" about movies as potentially beneficial in complementing traditional, pure-numbers predictive modeling. Alex says "assuming the customer is a static entity seems wrong to me, thus looking at databases only is not of much help."Coming from another angle, the thought that you can fit a training set without truly worthwhile real-world implications, knowledge-discovery guru Gregory Piatetsky-Shapiro seemed to agree: "Since the contest is based on a fixed data set, it is theoretically possible to find the optimal solution for it after a few million tries (:-). However, after the progress reached about 7% it slowed down significantly."
Gregory publishes KDNuggets and chairs the Association for Computing Machinery's Special Interest Group on Data Mining and Knowledge Discovery. He went on to tell me, "I think one of the main surprises is that information about movie genre, language, actors, director, etc. turned out to be unnecessary. All the information about movies is captured in ratings. Yehuda Koren, one of the winners of the Progress prize, told me that did not use any of the auxiliary movie info, contrary to my expectations."
So more data isn't necessarily better.
Check out two additional sources: Tom Slee's July 29, 2007 analysis, The Netflix Prize: 300 Days Later, and if you're really getting into this stuff, the Netflix Prize Forum.
Lastly, there's the unexpected but should-have-known-better result: clever people found a way to break the anonymity of the Netflix Prize dataset. Arvind Narayanan and Vitaly Shmatikov published a paper this fall that demonstrates that a small amount of non-anonymous information about an individual's movie viewing, for instance from posted Internet Movie Database (IMDb) reviews, can be matched to anonymized Netflix competition records. These findings have implications whenever supposedly-privacy-protected real-work records are public released.
Even without a winner 15 months in, the Netflix Prize competition has advanced not only approaches to recommendation engines, but predictive-analytics practices in general.
Seth Grimes is an analytics strategist with Washington DC based Alta Plana Corporation. He consults on data management and analysis systems.The $1,000,000 Netflix Prize competition has produced interesting results, even if no winner, 15 months in. Some of those results are a bit surprising; others we should have expected but didn't anticipate. So while participants haven't yet bettered the accuracy of Netflix' Cinematch recommendation algorithm by 10%, the threshold to win the $1 million prize, we can still take away lessons about predictive-analytics fundamentals.
About the Author
You May Also Like