Master Connected Intelligence In 8 Key StepsMaster Connected Intelligence In 8 Key Steps
Connected intelligence enables new value ecosystems by harnessing a network of data continuously from humans and machines. To master connected intelligence, technology architecture and delivery leaders will need to consider these steps.
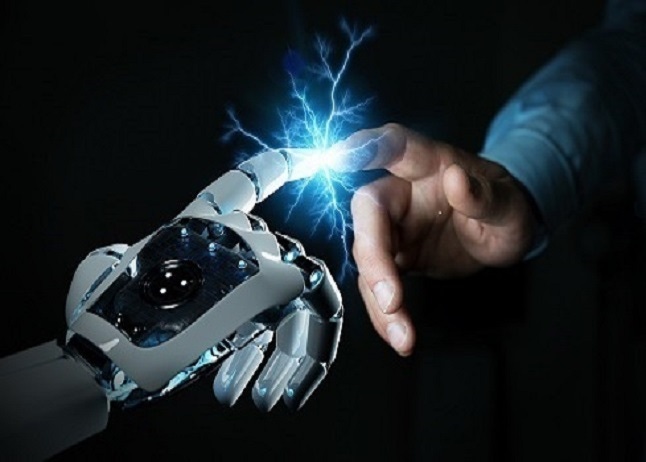
When it comes to emerging technologies, there’s promise and there’s reality. In some cases, promise is simply so high that the technology itself can never achieve it. In other cases, the new technology quietly finds its way into the market through both a growing need and practical applications.
Connected intelligence lies somewhere in the middle. Its vision is to leverage AI where humans and machines connect within a digital environment, to share knowledge, and to shape experiences for exponential business growth. It removes business and technical silos, opening AI to traverse venues, activities, and decisions. Connected intelligence is not a brand-new concept, but it’s becoming more prevalent given that two-thirds of enterprises are adopting AI and nearly half are adopting edge computing, according to Forrester. The vision of connected intelligence is finding its way into several real-world applications in the following ways:
Retail adapts to market disruption. Big box stores, disrupted by pandemic-driven buying trends, have connected supply chain data to inventory, e-commerce, and customer experience systems to set the right expectation for when critical items and orders will ship.
Medicine becomes personal. Healthcare providers can now connect to patients through telehealth visits, receive vitals from medical and personal devices, and link to pharmacy information and insurance to ensure that care plans are followed and remain effective.
Automotive delivers new experiences. Car companies have reimagined the driver experience, with electric vehicles addressing the car’s performance, learning driver and passenger experience preferences, and connecting to emerging charging station networks with amenities that customers want.
These examples show the real-world global potential for connected intelligence. So the question becomes: How can organizations transition from their current data strategy to a more connected intelligence approach? With connected intelligence, the linear and point deployment of AI models gives way to AI inferencing across distributed and complex flows of raw data, events, and model outputs in real time. This ups the ante on organizations’ enterprise data capabilities.
To evaluate organizational strength and readiness around data-connected intelligence, technology architecture and delivery leaders will need to master the following eight competences:
Discover and source to represent the business in data. Gathering data must be iterative and continuous to create, train, and optimize models. New data and data types (text, voice, image, audio, video) should augment and improve machine-learning (ML) models as data becomes more representative of the environment where AI is deployed. Marketplaces and exchanges can enable trusted data sourcing through data scientist self-service.
Capture and ingest data for quality and relevance. Fresh data is a prerequisite for performant AI. Data scientists need representative data moving into their sandboxes and training environments. In a connected intelligence model, data capture happens through streams to handle ephemeral data (e.g., time series). Data fabrics flex to match and shape data flows to keep data and insight in time with the digital experience and outcomes.
Curate and model data for better context. Forrester finds that 62% of global data and analytics decision-makers source external data. This requires constant classification, labeling, and certification of data to understand and govern data for self-service use. AutoML on structured data, computer vision, and behavioral ML on data use can combine to scale and speed up data curation and modeling to meet data scientist and connected intelligence solution demands.
Transform and prepare data for increased relevancy. Shaping data for ML is fraught with business logic, security, privacy, and regulatory considerations. Data scientists, data engineers, and data stewards collaborate and share transformations and preparation steps to streamline the influx of data. DataOps and data governance tools use ML to set data standards, schemas, and controls while providing the transparency and traceability of the data flow for impact and root-cause analysis.
Test and train to engender trust. Make AI testing holistic across data services, data models, business logic, governance, and service levels for data, metadata, and ML models and solutions. DataOps, ModelOps, and DevOps can play a critical role in end-to-end and granular testing and tracing of data flows affecting business logic and routing, as well as the ML model itself.
Deliver and deploy for scale. Consistent use of continuous integration and delivery (CI/CD) processes and practices keep DataOps, ModelOps, and DevOps connected and collaborative. Connected intelligence is built component by component and delivered as a product by data engineers, machine-learning engineers, and software engineers. Feature store platforms, data fabric, cloud, and edge computing systems create the backbone to deploy each component quickly, easily, at scale, and properly governed.
Execute and act dynamically to drive outcomes. Data and ML models must stay responsive and continuously adapt to business conditions and decisions. MLOps, data governance, and continuous lineage analysis ensure that connected intelligence is meeting service-level agreements. CI/CD allows dynamic versioning of data and model components to optimize the system, and monitoring and alerts provide an early warning system of potential outcome degradation.
Observe and evaluate for refinement and ongoing governance. Anomaly detection by DataOps helps preemptively identify and quarantine data on capture to mitigate upstream AI degradation or risk. One global financial firm has unified anomaly detection between data and ML to effectively enhance risks and threats for known and unknown bad-actor behaviors. Data observability and MLOps tools bring context to data anomalies and performance impact to mitigate AI governance and responsible AI risk conditions.
Ultimately, by understanding these eight key aspects of a connected intelligence model, technology architecture leaders will be able to both shift to an AI-first mindset and leverage AI to build the new, transformative experiences that business leaders envision and that customers demand.
To learn more about building a connected intelligence strategy and ask Michele Goetz specific questions, join the live webinar Navigating The Data Pitfalls Of Connected Intelligence on July 14.
About the Author
You May Also Like