MIT's Eyeriss GPU Could Transform IoT, AIMIT's Eyeriss GPU Could Transform IoT, AI
MIT researchers have developed a GPU called Eyeriss that could enable algorithms to run locally and instantly, instead of sending raw data into the cloud. This means that a future version of Siri could get you answers much faster.
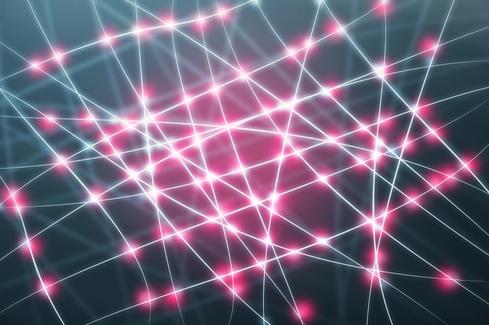
12 Ways To Connect Data Analytics To Business Outcomes
12 Ways To Connect Data Analytics To Business Outcomes (Click image for larger view and slideshow.)
Ask Apple's Siri, "What does the fox say?" and there's a notable pause.
It's not because Siri, too, is stumped by the question, but because your speech is raced off to Apple servers in the cloud, where its meaning is analyzed by a collection of processors -- known as a "neural network," for the way their operation mimics the human brain -- and an answer is hunted down.
Now, researchers at the Massachusetts Institute of Technology have developed a way to move that neural network onto your smartphone, and other devices.
At the International Solid State Circuits Conference in San Francisco, which took place earlier this month, MIT researchers presented a chip design called Eyeriss that's 10 times as efficient as a mobile GPU and so could allow mobile devices to run artificial intelligence algorithms locally, without a major drain to the phone's battery.
Processing the data locally means the algorithms can run without a WiFi connection -- the need to upload the data is why Siri, and Google Now don't work when a device doesn't have reception. It would also produce more instant results, as well as privacy benefits. Rather than sending raw data into the cloud, a device could send only the conclusions it reached.
In-device neural networks could be useful in "battery-powered autonomous robots,", as well as in furthering the Internet of Things., according to MIT's Feb. 3 press release. While everything from vehicles to appliances, civil-engineering structures, and livestock has sensors that report to a networked server, "with powerful AI algorithms on board, networked devices could make important decisions locally," MIT explained.
Other chipmakers are pursuing the same goal. At CES 2016, Qualcomm showed off a new Snapdragon 820 Automotive chipset, which enables cars to begin understanding objects around them, through a neural network, or so-called "deep learning."
Eyeriss' efficiency comes from a design that includes 168 cores, each of which has its own memory and can store and analyze data, while in a traditional GPU, a single memory bank is shared by all the cores.
Each of Eyeriss' cores can also communicate with those directly beside it, so all data doesn't need to be routed through the main memory.
[Read how Google is using machine learning to make smartphones smarter.]
And finally, Eyeriss features special circuitry that enables each core to maximize the amount of work it can do before going back to the main memory for more data.
At the conference, researchers had Eyeriss perform an image-recognition task, marking the first time ever that a neural network was demonstrated on a custom chip.
In a neural network, each node is trained to perform particular manipulations, in an effort to find correlations between raw data and labels applied by humans. With a chip like Eyeriss, MIT explained, an already trained network could be exported to a device.
In MIT's statement, Mike Polley, senior vice president of Samsung's Mobile Processor Innovation Lab, called Eyeriss "very important," and additionally applauded the MIT researchers' paper for "carefully considering how to make the embedded core useful to application developers by supporting industry-standard [network architectures] AlexNet and Caffe."
The MIT Research team is headed by Vivienne Sze, the Emanuel E. Landsman Career Development Assistant Professor in MIT's Department of Electrical Engineering and Computer Science. Sze did not share a timeline for Eyeriss' availability beyond the lab.
Are you an IT Hero? Do you know someone who is? Submit your entry now for information's IT Hero Award. Full details and a submission form can be found here.
About the Author
You May Also Like