Pacific Northwest National Laboratory's MDM ExperiencePacific Northwest National Laboratory's MDM Experience
CIO Jerry Johnson shares master data management pros, cons, and lessons learned.
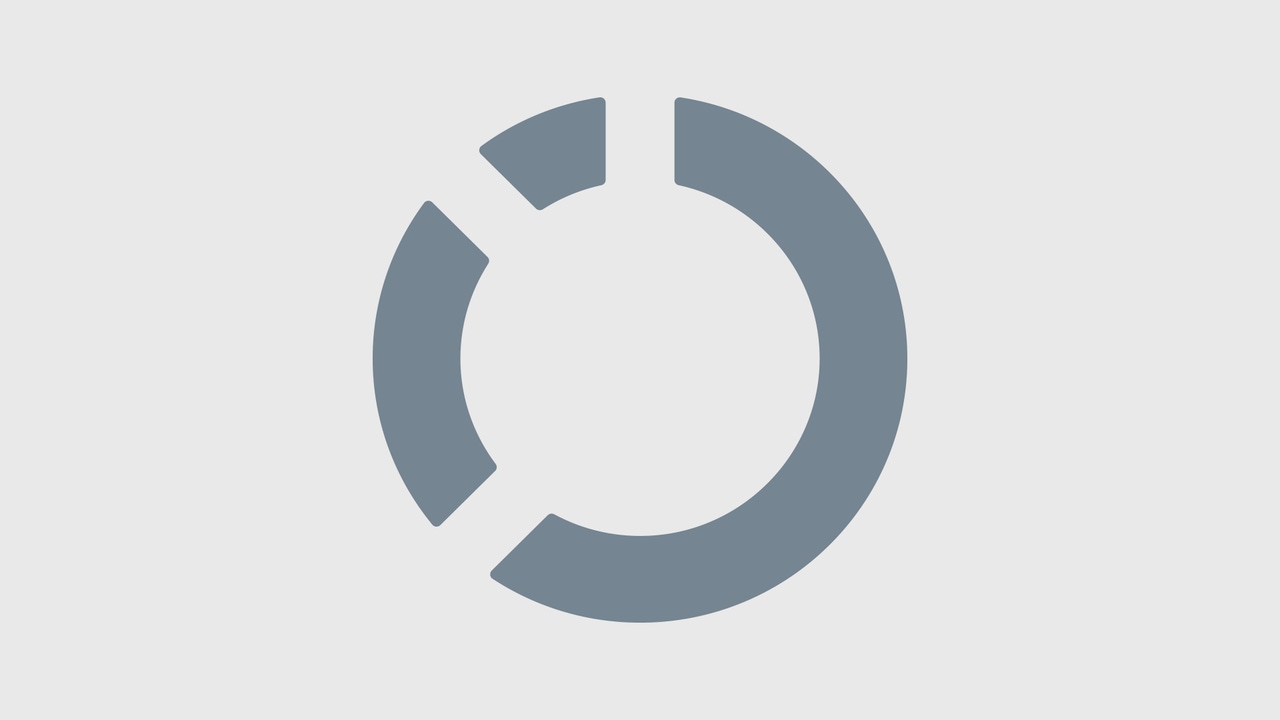
The long-running master data management program of Pacific Northwest National Laboratory comprises four main elements:
>> Data administration: Policies, processes, people, and tools (such as a data dictionary) help PNNL deliver authoritative, high-quality data resources, says CIO Jerry Johnson. This function defines the master data, eliminating redundant and nonauthoritative sources, and it ensures that data consumers know where to go for the "right" data for their applications and that they're authorized to have access.
>> Operational data store: PNNL established this source of reference data to avoid a performance hit on the main data store, provide a level of abstraction, and better manage access. For instance, information on employees is sourced in the agency's PeopleSoft HR system, but PNNL didn't want the time-reporting system dragging PeopleSoft to its knees each afternoon when 5,000 staffers recorded their daily time sheets, Johnson says. And the agency didn't want to have to update its time reporting system every time it did a PeopleSoft upgrade. Consequently, reference data like this is accessed by dependent transactions systems through an operational data store.
>> Data marts: PNNL established these sources of data for reporting to reduce the impact on transaction source systems and increase security, but also to improve response times and help with reporting.
>> Data warehouse: Contains transaction data from many systems. It supplies data to PNNL's operational data stores and data marts and is a single source for more detailed or ad hoc analyses of business data.
Quality And Consistency
Johnson says the main benefits of this architecture revolve around quality and consistency. PNNL doesn't need to do duplicative data entry, and the system is easy for developers to use; they simply write relational queries. It also enabled PNNL to deliver relatively painless application integration. "The operational data store allowed us to adopt a philosophy of implementing 'best in class' applications for each business function," he says. While this seemed like a win at the time, eventually PNNL ended up supporting more than 300 off-the-shelf and custom apps. That complexity has driven up maintenance costs.
In addition, delays in populating operational data stores delays business processes. "We initially refreshed HR data into the operational data store nightly," says Johnson. "But that meant that a new employee was not visible to reliant systems until the next day, so they couldn't have a computer account provisioned or begin their online training on their report day. As you begin to shorten the refresh times more and more, the impact on the transaction systems, and the cost of intermediate processing through the data warehouse, begin to exceed the cost and impact of having reliant systems simply access the source transaction system."
Johnson suggests having Web services deliver reference data from source transaction systems to the systems that consume the data. Because IT controls these services, you can modulate their impact on the transaction system, manage security, and provide a level of abstraction that protects consumer systems from changes in source systems. PNNL focused on siloed business processes and applications, then glued them together through data management. "We're paying the price for that," Johnson says, "and are now working to understand, model, and optimize our core business processes and the data they need."
Continue to the sidebar:
Three Guidelines For Implementing MDM Return to main story:
Mastering Master Data Management
information: July 11, 2010 Issue
Download a free PDF of information magazine
(registration required)
About the Author
You May Also Like