Selling Data Preparation PlansSelling Data Preparation Plans
Data preparation is a key background function but can also be mission critical. So how do you sell it to the leadership team?
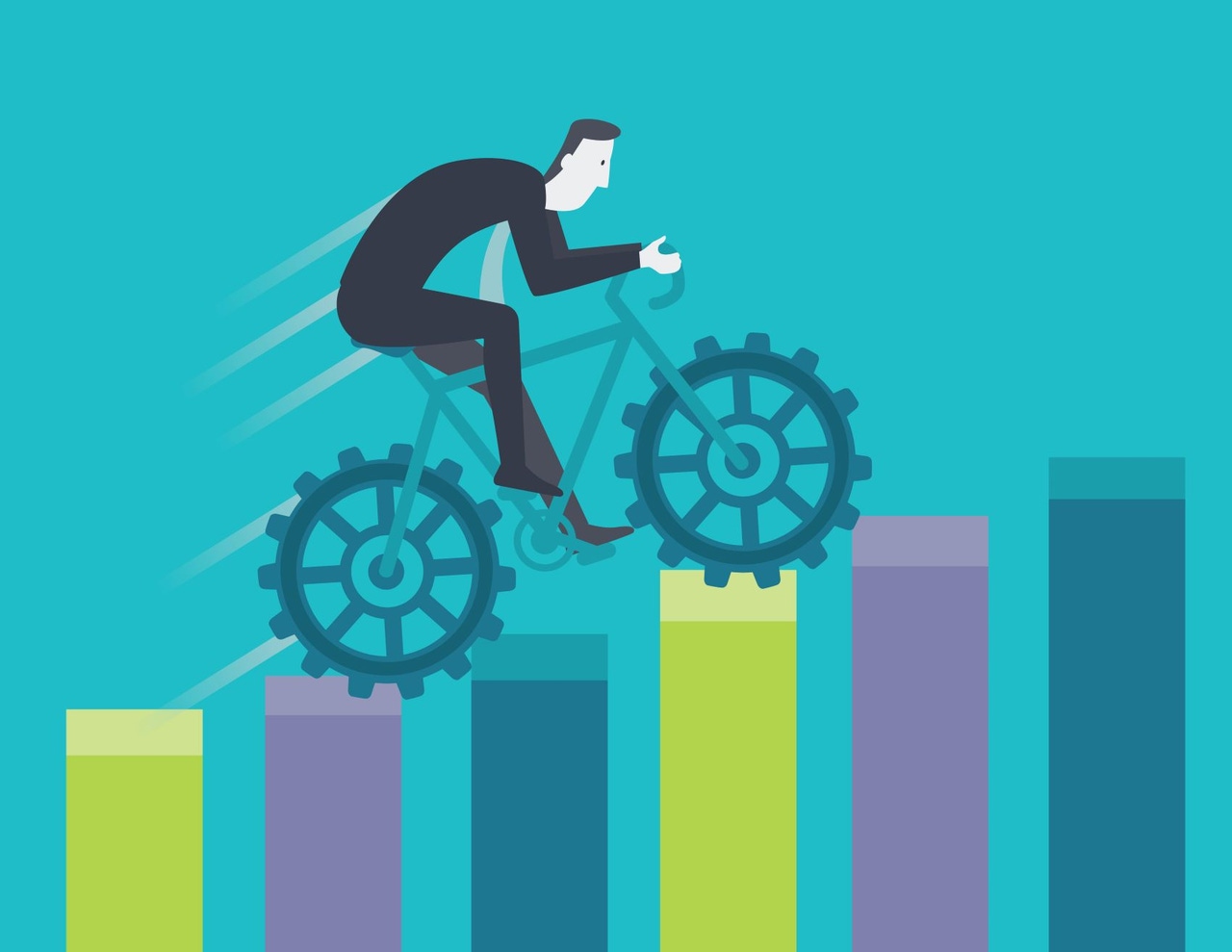
Earlier this year, an acquaintance went in for his annual physical, and his doctor detected an unusual heartbeat. Immediately, the EKG machine was wheeled in, and he had an EKG right there in the office. A few days later, he went to the same facility so the cardiology department could run a stress test. Cardiology brought in an EKG machine, and he asked, “Wait, I just had an EKG a few days ago. Don’t you have that record?”
Cardiology said “yes,” but even though the cardiology specialists had the EKG results from his doctor’s office upstairs, they wanted to run their own test.
My friend’s outcome was fantastic, and they found his heart normal. But when this person, who was also an IT professional, shared his story with me, we both scratched our heads at why the cardiology department insisted on performing its own EKG. We surmised that they simply didn’t trust the data they already had.
This is a familiar story that many CIOs have faced since digitalization initiatives started: What can you do, and how can you promote user digital literacy if you can’t trust the data?
The market knows this, too. It’s why there are countless offerings for data preparation and cleaning tools that extract, transform, and load (ETL) data that is turned into quality data that then is placed in centralized data repositories that everyone can use.
This sounds good, but how easy is it to put data preparation into practice?
Two years ago, the Harvard Business Review wrote, “Companies have been working to become more data-driven for many years at this point, with mixed results. These efforts play out over time in organizations, and persistence, resilience, execution, and a relentless drive to employ data to make more informed business decisions are what distinguishes those companies that prevail from those who continue to struggle. But while the mission may remain steady, the particulars change.” HBR went on to say that only 26.5% of organizations they surveyed reported that they had created an organization that was data-driven, and 91.9% of executives said cultural changes were a challenge to becoming data driven.
For CIOs, these changes and challenges are most obvious when it’s budget time. How can you cost-justify a major investment in staff time and data cleaning and preparation tools when it is all “back office” work that no one sees an upfront benefit from?
It’s difficult. And what most CIOs and data practitioners have discovered is that you can’t justify a major data preparation investment in most cases. That is, unless the need for accurate, timely and relevant data presents so much risk to the systems the company runs that you can’t avoid it.
Hospitals and medical clinics can’t afford to have data that is corrupt or less than accurate if they are running artificial intelligence or analytics on possible causes of a certain condition. That also applies if they are using AI to uncover the best courses of treatment for a certain type of cancer, or if they need to verify and have confidence about cardiac results.
Air traffic controllers can’t afford to have inaccurate information about flight patterns, incoming and outgoing air traffic, availability of runways, or current weather conditions. Electrical power companies need up-to-date information about the soundness of their power distribution network and their ability to failover to other sources should a natural disaster or outage occur. All are examples of mission-critical, data-driven use cases that require nothing less than data that is accurate, relevant, complete and timely.
The takeaway for CIOs is that they can gain funding and backing for data cleaning and preparation if they link what is normally perceived as a back-office data cleaning exercise to mission-critical system development that will succeed only if the data is good.
An excellent litmus test for a data preparation proposal is to try it in informal meetings or even in a presentation to the CEO and the board to gauge their response. If data preparation clearly is a mandate for an application such as AI for cancer diagnosis or treatment, it will get approved, barring a budget affordability issue for both the data preparation and the system.
That is also the time to explain why data preparation could become an ongoing exercise as the system grows.
As an example, one European medical clinic began with a cancer diagnosis and treatment AI-based system that analyzed all the patient data that was available from in-country data sources. Wanting to expand its patient data, the clinic then opted to expand patient data feeds from all European countries. The data expansion required incoming data from each new source to be vetted, cleaned, formatted, and loaded into the clinic’s central data repository so the AI system could process data that was trustworthy and of high quality.
There are many examples where the data in a single application needs to be grown or expanded to be useful, and more data preparation is needed. These are the best places to apply data preparation, because quality data lays the foundation for trust in mission-critical systems, and everyone in the business understands why it is mission-critical.
About the Author
You May Also Like