Text Data Quality: Mistakes and MoreText Data Quality: Mistakes and More
I wrote recently on Text Data Quality, looking at issues that affect analytical accuracy, that "the basic text data quality issue is that humans make mistakes, and the challenge is that people's natural-language mistakes defy easy, automated detection." This topic and related non-erroneous vagaries of human language bear further exploration...
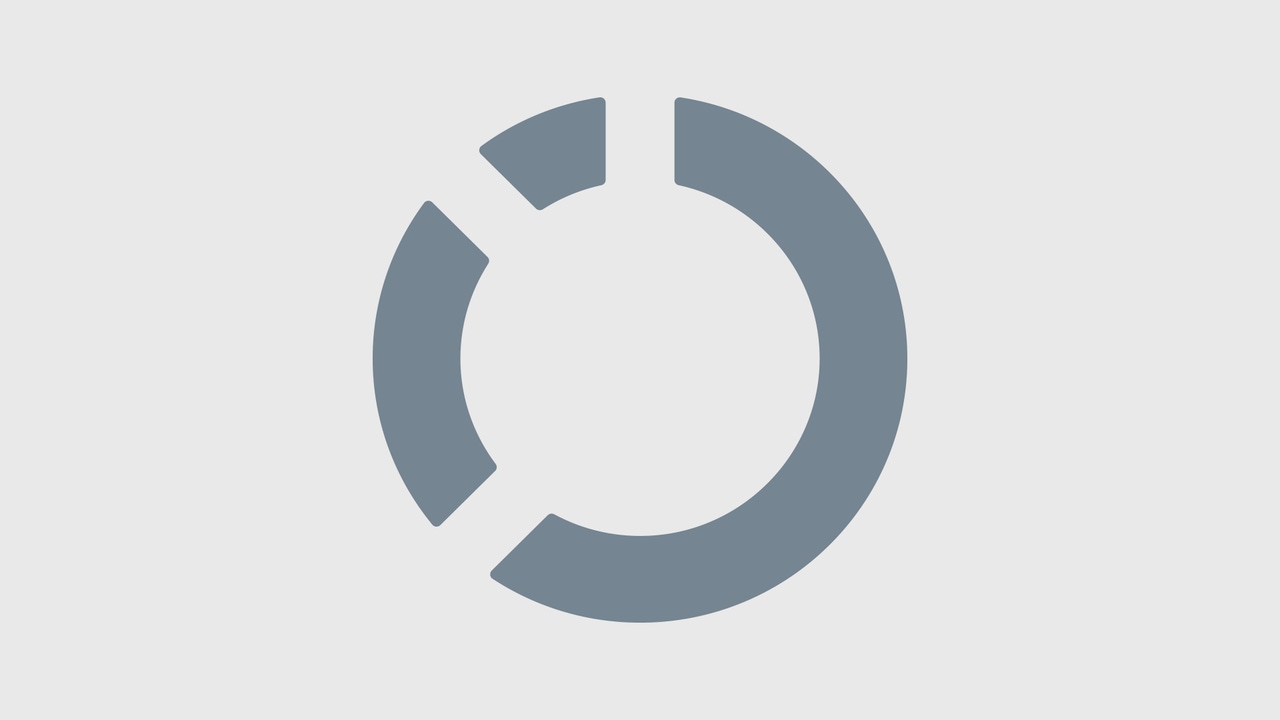
I wrote recently on Text Data Quality, looking at issues that arise in working with textual information that affect analytical accuracy. I wrote, "The basic text data quality issue is that humans make mistakes, and the challenge is that people's natural-language mistakes defy easy, automated detection." The topic of mistakes -- and the related topic of the non-erroneous vagaries of human language -- bears further exploration.
This current follow-on was prompted by a tweet of Manya Mayes's, "Text mining/social media analysis-there are at least 4 ways to misspell a word, and in some cases (company/brand names) upwards of FIFTEEN!" Indeed, in an article On Text Data Quality Manya posted to SAS's "The Text Frontier" blog -- Manya is chief text mining strategist at SAS -- she provides examples that recap "The Ten Transgressions of Text" per a presentation she gave at last June's Text Analytics Summit.It's likely that most everyone who works with database systems -- for text or for transactional or operational data -- has encountered the "transgressions" Manya describes. I know I have. I had one BI client, a professional association whose membership-processing software provided a free-text field rather than a drop-down for entering the state of residence. The database held for Pennsylvania (for example): Pennsylvania, Penn, Penna, Penns, PA, Pa -- several of those with and without the period that indicates an abbreviation. The answer is to come up with a standard coding and constrain the user's choices, but there's simply no constraining people who post to the Internet.
If you want to analyze Net-sourced information correctly -- or e-mail, contact-center notes, transcripts, etc. -- you have to handle both misspellings and variants including abbreviations. Want to see what I mean? Matt Hurst, who is a scientist at Microsoft Research, posted a blog article last March titled Watching the Watchmen, tracking blogosphere attention to various Watchmen movie characters using Nielsen BuzzMetrics' BlogPulse application. I commented on Matt's blog that, never having read Watchmen, I would have naively misspelled the names of two of the characters Night Owl and Silk Specter rather than Nite Owl and Silk Spectre. It seems the BlogPulse application isn't smart enough correct the spelling errors.
Can we quantify all those language variations that Manya Mayes groups under the "transgressions" heading? (In the world of structured databases, this would be called data profiling.) I'm sure there are studies out there that examine misspellings and use of abbreviations, slang, spelling variants, etc. in various types of materials and contexts. Eszter Hargittai's Hurdles to Information Seeking: Spelling and Typographical Mistakes During Users' Online Behavior is the only such research I'm familiar with, a look at spelling or typographical errors made by a sample of 100 Internet users during their online activities. Hargittai's 2002 research found "Almost a quarter (23%) of respondents made at least one typo during their search session, over half (52%) made at least one spelling mistake, and almost two-thirds (63%) did one or the other."
To me, understanding of error and language-variation frequencies gets much more interesting when it moves beyond description to application, for instance, in the "Did you mean:" suggestions that you get from Google and similar systems, which are surely driven not only by dictionary and lexicon look-ups but also by examining the statistical characteristics of terms that are not found by look-up and their associations.
Although I can't quantify the occurrence of text data quality issues, I do know that it's possible to discover opportunity in the vagaries of natural language. Manya Mayes classifies spelling errors and other language quirks as "transgressions." From another perspective, they present opportunities. Errors can be features, potential assets in automating sense-making processes, as I explore in my Text Data Quality article. In the end, I find the ability of text analytics tools to discover value in human communications, complete with imperfections and irregularities, to be truly compelling.
Hold the dates: The 2010 Text Analytics Summit, the 6th U.S. summit, is slated for May 25-26 in Boston. I will again chair the conference. If you have an idea for a talk, please get in touch.I wrote recently on Text Data Quality, looking at issues that affect analytical accuracy, that "the basic text data quality issue is that humans make mistakes, and the challenge is that people's natural-language mistakes defy easy, automated detection." This topic and related non-erroneous vagaries of human language bear further exploration...
About the Author
You May Also Like