The Automation Paradox: The Rise of Human-Led AutomationThe Automation Paradox: The Rise of Human-Led Automation
The more we automate data analytics, the more work is required of humans to cover edge cases, provide high-level scrutiny, and put meaning behind the insights.
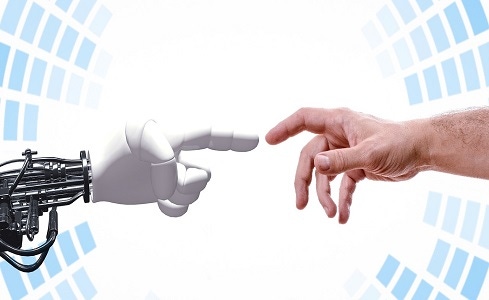
Data is the most critical resource an organization possesses. Data allows us to make informed decisions. It provides critical insights into our customers and the experiences we deliver. It helps create operational efficiencies that lead to lower costs and higher margins.
But, right now, we’re drowning in data. We have so much that it’s become difficult to sort good, relevant data we need from the noise we don’t. We’re spending a fortune collecting, managing, and analyzing data across the business, but we’re not seeing the ROI.
Thankfully, automation powered by artificial intelligence (AI) and machine learning (ML) is helping us get a better handle on our data. Software can now search through large data sets to identify the relevant data for each purpose. It doesn’t matter if we’re drowning in data -- the machines will tell us what’s good and what’s bad.
Or so we thought. Maybe automation isn’t the silver bullet we think it is.
The Problem of Machines
At the most basic level, automation enlists a machine to perform rote, repetitive tasks more cheaply and efficiently than a human. Whether it’s a die cut press punching out thousands of identical circles or AI recommending your next video, the principle is the same.
The digital age has brought trivial conveniences like reminders to order more laundry detergent to life-saving operations like donor matching. None of this is possible without automation. But machines can only get us 90% there. They’re great at consuming and analyzing large volumes of data but still have trouble with edge cases. Sure, we can continue to train algorithms to cover more of these exceptions, but at a certain point, the number of resources going into development starts to outweigh the benefit.
This ability to easily and seamlessly apply known principles and criteria to edge cases is what sets humans apart from machines. We’re precise thinkers. We can look at an instance and make a best judgement decision that’s almost invariably correct. Machines are approximators. They look at the whole and decide based on how similar use cases were handled previously, often delivering poor results.
Therein lies the AI paradox: The more we automate data analytics, the more work is required of humans to cover edge cases, provide high-level scrutiny, and put meaning behind the insights.
The Rise of Human-Led Automation
To drive AI in a sensible, efficient, and ethical way, enterprises need to let machines do what they excel at but make sure humans are there to provide supervision. Based on explainable AI, the notion that results need to be understood and explained by humans, this is a hands-on, continuous cycle that requires involvement in every phase of AI from problem definition and development to ongoing data governance.
Here are three considerations for putting the human touch back into AI-powered solutions:
1. Set corporate values
AI is only as good as the data you feed into it. If existing processes are implicitly biased, then any algorithm based on these historical precedents will carry those biases over to automated processes. Enterprises first must define the values they care about, ensure human compliance, and then apply those values to automated processes.
2. Put humans at the source of teaching
In machine-trained learning, AI creates and trains an algorithm without human intervention. Machines don’t have ethics or morals and can’t make judgement calls. All they know is what’s been taught to them, and, like a game of telephone, these lessons tend to be watered down the further they get from a human. Making humans train algorithms is a win-win. Humans can identify and teach edge cases for machines while machines offload much of the manual, tedious tasks.
3. Ensure human-led governance
AI models need to be continually monitored, measured, and recalibrated. Left alone, these models can unintentionally shift based on outside factors. Called drift, these shifts can lead to unintended and undesired results. Similarly, ethical AI, a component of Explainable AI, ensures that machines operate under a system or moral principles defined by developers. If models drift far enough, they can lose their ability to act as intended. While monitoring drift can be done by machines, any issues that arise need to be escalated to a human who can make a judgement call whether to intervene. Subsequent training should also be handled by humans, ensuring that the algorithm is recalibrated to return optimal results. It’s clear that humans—with the right subject matter expertise—are the best judges of model drift. Only they, not machines, have the high-level experience, cognitive ability, and understanding of critical nuances to make these judgement decisions.
Keeping Machines Honest Requires a Human Touch
AI has the power to transform the way we work, live and play, but we still need humans to instill common sense and supervision that only people can provide. Putting the human touch back into automation requires a commitment that starts with defining and enshrining corporate values and continues through algorithm development, training, and ongoing governance. Machines will one day take on a much larger role in our daily lives, but we still need humans to keep them honest.
About the Author
You May Also Like