To Meet AI/ML Needs, Leaders Need the Right ToolsTo Meet AI/ML Needs, Leaders Need the Right Tools
Deployment of AI and ML technologies can be a boon for businesses, but CDOs and analytics leaders need technical, financial, and human resources to bring projects to fruition.
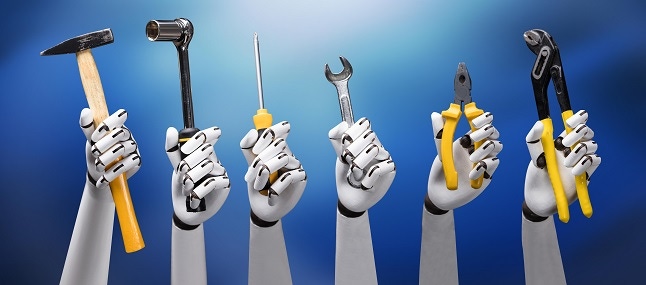
Chief data officers and analytics officers are facing several challenges when it comes to selecting and implementing AI/ML tools to help drive digital transformation efforts and streamline processes.
For example, they may struggle to find the right tools tailored to their specific needs, have difficulty integrating these tools with existing systems, and may not have the necessary data infrastructure to support these tools.
Additionally, a shortage of skilled data scientists and machine learning engineers can make it challenging to implement AI/ML tools effectively.
The use of AI/ML tools can help facilitate automation of repetitive tasks, identify patterns in large datasets, and generate accurate predictions and insights to inform business decisions.
For CDOs and analytics officers, AI/ML tools can process large amounts of data quickly and accurately, uncovering valuable insights and patterns that can be used to improve business performance across industries.
CDO Leaders Require a Range of Resources
Marinela Profi, data scientist and analytics product lead at SAS, says to successfully navigate the complexities of AI/ML initiatives and meet business goals, CDO leaders require a range of essential resources.
“They need skilled data science and machine learning experts to design, implement, and maintain AI/ML models,” she explains. “They also require access to clean, structured, and relevant data is critical to train and test these models.”
She adds a robust IT infrastructure that can handle machine learning algorithms’ data processing, storage, and computational requirements is essential.
“Collaboration tools and analytics platforms are necessary to ensure efficient and effective teamwork,” Profi says. “Additionally, it’s critical to develop and enforce governance and security policies that cover data privacy, bias mitigation, and algorithmic transparency.”
Profi notes the immense interest in AI/ML is also leading to a tech talent shortage that can complicate efforts to drive innovation using these tools.
“There’s a shortage of skilled data scientists, machine learning engineers and other technical professionals,” she explains. “To address this challenge, organizations need to invest in low/code no-code tools to increase productivity of their teams.”
Discerning Between Generative AI, Deep Learning
Mike Loukides, vice president of emerging tech content at IT training services specialist O’Reilly Media, says when discussing AI/ML tools, it’s important to be clear whether one means generative tools like ChatGPT or older neural networks and “deep learning.”
“ChatGPT and its friends are all the rage and have made it difficult to talk about other tools and techniques,” he says. “But while these generative tools are impressive, they really aren’t designed for data analysis.”
He says CDOs and data analysts need to investigate the more traditional automated ML (AutoML) tools, noting all the cloud providers have AutoML services that will take a data set, build a model, and (in some cases) deploy the model with a single click.
There are also standalone tools from companies like Databricks, DominoDataLab, Dataiku,DataRobot, and many others.
“What’s important about these tools isn’t that you load them up with data, and a model for your problem pops out,” he explains. “Anyone working with data knows that you get answers by performing experiments -- repeatedly.”
This requires varying the training data, varying the parameters, testing the results, and then going back and starting again.
“That’s a lot of work -- and for the most part, it’s dull, non-creative work,” Loukides says. “AutoML tools give analysts the ability to run lots of experiments, many times more than they could run if they had to set up each experiment by hand, or even with some simple scripts. That’s really a superpower.”
Building Knowledge, Securing Executive Buy-In
Krishna Subramanian, COO and president of Komprise, adds a machine learning solution is only as good as its training data.
“Therefore, it is vital to have people who understand ML algorithms and who can decide what data should be used to train the AI/ML, what use cases it should work on, and how to automate the workflow,” he says.
He adds that since AI/ML is still nascent, there are not many CDOs and data scientists with deep knowledge of AI/ML tools and processes.
“By starting small and showing the time efficiency and value of automating an immediate pain point such as identifying potential security threats through anomalies or enriching data for analytics, you can get executive buy-in to fund larger AI/ML projects with more resources,” he advises.
Profi admits that as a CDO or analytics leader, convincing executives to provide necessary resources can be daunting but offers some advice to increase their chances of success. “To start, communicate the value of these resources and explain how they can help your organization achieve its goals and improve its bottom line,” she says. “Using data and metrics to support your argument can be especially persuasive.”
Additionally, developing strong relationships with executives is key, as is understanding their priorities and concerns. Seek opportunities to collaborate with them on initiatives that align with their goals.
“Lastly, demonstrating the impact of data-driven insights through case studies and success stories can be incredibly beneficial,” Profi says. “Don’t forget to educate executives on the importance of AI/ML. Helping them understand how data can make your organization more competitive and efficient is a crucial step in securing the resources you need.”
What to Read Next:
5 Ways to Embrace Next-Generation AI
About the Author
You May Also Like