To Solve Your Data Science Talent Gap, Embrace DiversityTo Solve Your Data Science Talent Gap, Embrace Diversity
With so many companies competing for data science talent, taking an inclusive strategy to data science isn't just good for business -- and more ethical -- it is a necessity.
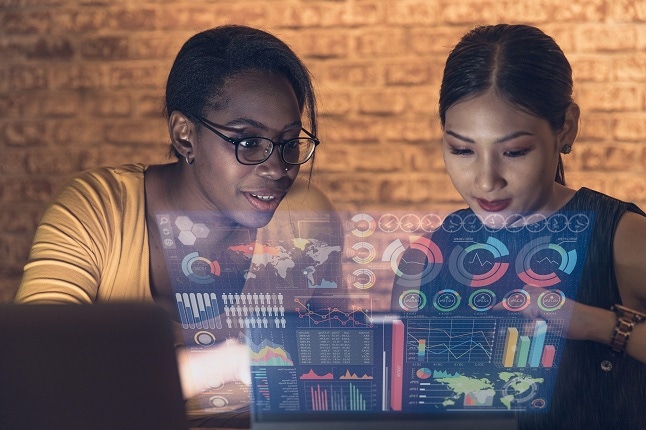
Being a data scientist has been one of the ‘best jobs in America’ for several years now. It regularly outranks roles like software engineer and product manager, not just because it is more interesting and, according to Tom Davenport, sexier, but because of the insatiable demand for data scientists. Glassdoor reports that job postings for data scientists have grown 480%, and it is no exaggeration that every fast growing organization needs more data scientists. They are the crucial ingredient for turning raw data into innovative new products and services, and data-driven business transformation.
So, how can organizations scale their data science capabilities in the face of such scarcity? The answer is inclusivity.
‘Normal’ Data Scientists Do Not Exist
The notion of a “normal” data scientist is a myth. Today’s data scientists come from all walks of life with a variety of skills, experience, and training. Their backgrounds range from computer science and applied physics to bioinformatics and beyond. Some come from non-quantitative backgrounds and have become data scientists by dint of experience and training programs. Between them, this ragtag bunch have perhaps the widest variety of skills, tools, methods, and methodologies of any group ever lumped together as a profession.
What may look like a flaw, is actually a strength because there is no such thing as a “normal” data science project, either. Whereas one project may involve a deep learning-based computer vision model, another may end up using traditional rules-based text analytics. No data scientist, irrespective of background, will come trained in both methods, let alone the large and growing universe of data science techniques.
Further, every data scientist will be regularly called upon to apply skills from a range of other disciplines. And these benefits are on top of the regular innovation, creativity, and decision-making improvements that the melding of these diverse perspectives brings about. However, to scale data science teams and access these benefits, organizations need to overhaul the way they recruit and support their data scientists.
People, Process and Technology Strategies
Every organization that wants to scale their data science capabilities needs to embrace this diversity of data scientists because there simply are not enough of them with a given profile to hire them exclusively. However, most organizations persistently work against their own interests. They attempt to over-standardize data science job descriptions and career paths and seek to limit data scientists to a narrow set of tools. All these actions limit the organization’s ability to scale.
Instead, organizations need to plan for and establish a workplace that is inclusive of all data scientists. To do so, they must tackle the people, process, and technology aspects of data science diversity:
People: Recruit diverse profiles. Recruiters and hiring managers need to explicitly seek candidates from a range of different academic backgrounds, experiences, and skills. Rather than a one-size-fits-all profile they should create a range of profiles, ideally aligned to your different types of current and future projects. Recruit individuals based on their transferable skills and their demonstrated ability to learn and apply relevant methods as witnessed by their past accomplishments. Above all, resist the temptation to screen candidates based on keywords linked to specific degrees, tools, programming languages, and frameworks.
Process: Encourage a diverse community. Once you’ve recruited a diverse team, you need to nurture it. It is all too easy to create a narrow data science career track with a set list of competencies at each rung. The result is unhappy data scientists, low productivity, and turnover. Instead, create individualized career paths with a high degree of flexibility. Cultivate a sense of belonging by establishing formal and informal channels for collaboration within and across data science teams.
Technology: Empower them with a diverse toolkit. Every data scientist comes with tools that they have spent years developing expertise in. Yes, every good data scientist wants to learn new ones, but there is no faster way to frustrate a data scientist, and reduce their productivity, than by preventing them from using the tools that they know and the tools that are most suited to the project. You do not need to standardize tools to drive collaboration and productivity. The best data science platforms today enable you to use all the mainstream data science IDEs, languages, packages, distributed compute frameworks and hardware whether they are open source or proprietary. In addition, these same platforms enable data scientists to collaborate and share results, code, model artifacts, etc. irrespective of the tools they were created with. And they provide the governance, security, and shared access to infrastructure to boot.
Data science-driven companies reap benefits across the board -- in increased revenue, operational efficiency, and innovation, but in order to achieve this, businesses need talent to make sense of their data and build models to power new applications and streamline processes throughout the organization. With so many companies competing for data science talent, taking an inclusive strategy to data science isn't just good for business -- and more ethical -- it is a necessity. Data is not the strategic resource of the 21st century, data scientists are.
Read more about:
Diversity, Equity, and Inclusion (DEI)About the Author
You May Also Like