What To Consider When Hiring Data Science TalentWhat To Consider When Hiring Data Science Talent
Dealing with the data science talent crunch isn't just about competing for talent; it also requires the right data science strategy to help the analytics team be effective and efficient.
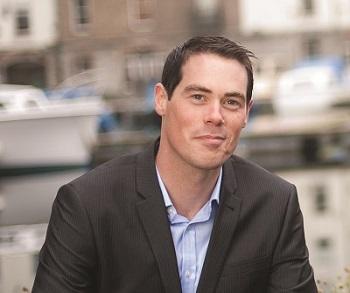
Let’s face it: The corporate world is still struggling with how to best leverage its data. It’s not a new challenge we face, but the problem is only exacerbated as more data is exchanged and created at petabyte scale. The proliferation of data, and its potential value for organizations have increased demand for data science professionals.
When a company seeks to hire a data scientist, it's typically seeking someone with skills in advanced programming and statistical analysis, along with expertise in a particular industry segment. The need is great, and the skills gap is widening. However, new technology presents greater opportunities for analysts to become data scientists. A recent report by Gartner predicts that more than 40 percent of data science tasks will be automated within the next three years. This is expected to increase productivity and usage of data and analytics by citizen data scientists.
According to Gartner, “Most organizations don’t have enough data scientists consistently available throughout the business, but they do have plenty of skilled information analysts that could become citizen data scientists.” When equipped with the proper tools, analysts can perform greater in-depth analysis and create models that leverage predictive or prescriptive analytics.
According to O’Reilly’s recent Data Science Salary Survey, data scientists don’t come cheap. How can today’s organizations harness the brains behind data science to get the most out of their investment, whether in talent or technology? Here are some things to consider when building your data science team:
Data science is a team sport. There are many facets to creating successful data science teams in a practical, operational sense. It’s rare to hire just one or two on staff, so remember that for data scientists as much as any other role, strength comes in numbers.
Outsource to innovate. If you do the math, a team of seasoned data scientists – let’s say only five – will cost you well over $1 million annually in fixed costs. Like many in IT functions, they’re likely to be pulled in many directions. Having a dedicated resource to optimize your systems with networks getting increasingly smarter with every interaction via machine learning is one way to ensure that projects are efficient while blending technology platform costs with the costs for data science talent that drives them.
Balance functional and strategic tasks. Part of the reason data scientists are so in demand is because they have concrete skills in predictive analytics that others – in IT and business roles – lack. That being said, you’ll need sufficient talent and resources to both write and maintain software and algorithms while also gathering insights from internal teams and customers to customize and optimize the logic behind them.
Set data scientists up for success with the right data management systems. High volume, omni-channel systems are very complex – and time consuming – to manage. Having a hub where data at the individual customer level is aggregated helps set the foundation for data scientists to really shine. Finding ways to automate processes so that the right data is available on demand will make any data scientist’s life easier and will make more possible under their strategic guidance.
Expect to "see inside the black box" of AI. A data scientist should be tasked with explaining the process of machine learning and artificial intelligence in layman’s terms to bring others into their realm throughout the enterprise. This is essential for gathering insights that make predictions stronger and actions more focused by design. And, as marketers take on greater oversight of data, it’s important that CMOs and other decision-makers find complementary talent and technology to help them see the big picture to explore all that’s possible with their data.
We know the power of data science at Boxever: Nearly 10 percent of our people are data scientists themselves. As we all innovate having the right team behind big data initiatives is essential to success. Are you confident you have the right one in place now and for the future?
Dermot O’Connor is co-founder of Boxever and vice president of product and engineering.
About the Author
You May Also Like