Your Data Is Biased, Here's WhyYour Data Is Biased, Here's Why
Biased data can lead to bad decisions. Most business leaders aren't aware of the problem just yet, but they need to be because they're ultimately responsible.
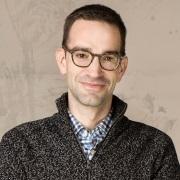
Bias is everywhere, including in your data. A little skew here and there may be fine if the ramifications are minimal, but bias can negatively affect your company and its customers if left unchecked, so you should make an effort to understand how, where and why it happens.
"Many [business leaders] trust the technical experts but I would argue that they're ultimately responsible if one of these models has unexpected results or causes harm to people's lives in some way," said Steve Mills, a principal and director of machine intelligence at technology and management consulting firm Booz Allen Hamilton.
In the financial industry, for example, biased data may cause results that offend the Equal Credit Opportunity Act (fair lending). That law, enacted in 1974, prohibits credit discrimination based on race, color, religion, national origin, sex, marital status, age or source of income. While lenders will take steps not to include such data in a loan decision, it may be possible to infer race in some cases using a zip code, for example.
"The best example of [bias in data] is the 2008 crash in which the models were trained on a dataset," said Shervin Khodabandeh, a partner and managing director of Boston Computing Group (BCG) Los Angeles, a management consulting company. "Everything looked good, but the datasets changed and the models were not able to pick that up, [so] the model collapsed and the financial system collapsed."
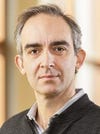
Shervin Khodabandeh
What Causes Bias in Data
A considerable amount of data has been generated by humans, whether it's the diagnosis of a patient's condition or the facts associated with an automobile accident. Quite often, individual biases are evident in the data, so when such data is used for machine learning training purposes, the machine intelligence reflects that bias. A prime example of that was Microsoft's infamous AI bot, Tay, which in less than 24 hours adopted the biases of certain Twitter members. The results were a string of shocking, offensive and racist posts.
"There's a famous case in Broward County, Florida, that showed racial bias," said Mills. "What appears to have happened is there was historically racial bias in sentencing so when you base a model on that data, bias flows into the model. At times, bias can be extremely hard to detect and it may take as much work as building the original model to tease out whether that bias exists or not."
What Needs to Happen
Business leaders need to be aware of bias and the unintended consequences biased data may cause. In the longer-term view, data-related bias is a governance issue that needs to be addressed with the appropriate checks and balances which include awareness, mitigation and a game plan should matters go awry.
"You need a formal process in place, especially when you're impacting people's lives," said Booz Allen Hamilton's Mills. "If there's no formal process in place, it's a really bad situation. Too many times we've seen these cases where issues are pointed out, and rather than the original people who did the work stepping up and saying, 'I see what you're seeing, let's talk about this,' they get very defensive and defend their approach so I think we need to have a much more open dialog on this."
As a matter of policy, business leaders need to consider which decisions they're comfortable allowing algorithms to make, the safeguards which ensure the algorithms remain accurate over time, and model transparency, meaning that the reasoning behind an automated decision or recommendation can be explained. That’s not always possible, but still, business leaders should endeavor to understand the reasoning behind decisions and recommendations.
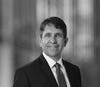
Kevin Petrasic
"The tough part is not knowing where the biases are there and not taking the initiative to do adequate testing to find out if something is wrong," said Kevin Petrasic, a partner at law firm White & Case. "If you have a situation where certain results are being kicked out by a program, it's incumbent on the folks monitoring the programs to do periodic testing to make sure there's appropriate alignment so there's not fair lending issues or other issues that could be problematic because of key datasets or the training or the structure of the program."
Data scientists know how to compensate for bias, but they often have trouble explaining what they did and why they did it, or the output of a model in simple terms. To bridge that gap, BCG's Khodabandeh uses two models: one that's used to make decisions and a simpler model that explains the basics in a way that clients can understand.
BCG also uses two models to identify and mitigate bias. One is the original model, the other is used to test extreme scenarios.
"We have models with an opposite hypothesis in mind which forces the model to go to extremes," said Khodabandeh. "We also force models to go to extremes. That didn't happen in the 2008 collapse. They did not test extreme scenarios. If they had tested extreme scenarios, there would have been indicators coming in in 2007 and 2008 that would allow the model to realize it needs to adjust itself."
A smart assumption is that bias is present in data, regardless. What the bias is, where it stems from, what can be done about it and what the potential outcomes of it may be are all things to ponder.
Conclusion
All organizations have biased data. The questions are whether the bias can be identified, what effect that bias may have, and what the organization is going to do about it.
To minimize the negative effects of bias, business leaders should make a point of understanding the various types and how they can impact data, analysis and decisions. They should also ensure there's a formal process in place for identifying and dealing with bias, which is likely best executed as a formal part of data governance.
Finally, the risks associated with data bias vary greatly, depending on the circumstances. While it's prudent to ponder all the positive things machine learning and AI can do for an organization, business leaders are wise to understand the weaknesses also, one of which is data bias.
About the Author
You May Also Like