Leveraging AI, Machine Learning to Enhance Cloud InteroperabilityLeveraging AI, Machine Learning to Enhance Cloud Interoperability
Organizations looking to improve interoperability between clouds could consider artificial intelligence and ML technologies to help automate tasks, but the undertaking requires a clear roadmap.
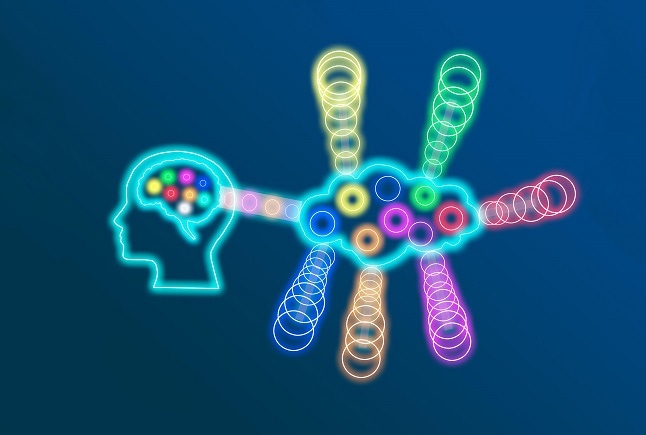
The multiple benefits companies can reap from a multi-cloud strategy, including flexibility and agility, can’t be fully achieved unless IT leaders improve interoperability and visibility into those clouds.
Meanwhile, businesses that are relatively mature on their journey of adopting artificial intelligence and machine learning technologies have recognized their organizations will be hybrid- or multi-cloud now or in the foreseeable future.
Between changes in the business, growing cloud costs, data sovereignty regulations, worries about cloud lock-in, and legacy infrastructure, no organization can or should want to be on a single cloud.
Davis McCarthy, principal security researcher at Valtix, says cloud service providers (CSP) have proprietary protocols and APIs that don’t seamlessly integrate with every organization’s tech stack. “Something like secure networking in the multi-cloud is particularly challenging because each CSP handles data differently,” he says.
The use of AI/ML can help standardize datasets and apply expert-level context and pattern-matching to detect security threats, resource consumption and maintain compliance.
“Supporting another cloud is, at best, only an acquisition or regional expansion away,” says Thomas Robinson, COO at Domino Data Lab. “Since no cloud vendor has an incentive to make it easy to transfer data and workloads between clouds, or to on-prem infrastructure, the result is data silos.
Using AI/ML to Automate Tasks
Anant Adya, EVP and GTM head for Cobalt at Infosys, explains effective and efficient cloud interoperability often requires creative solutions by the cloud engineering team in charge.
“AI and ML can improve cloud interoperability by automating repetitive or redundant tasks, allowing engineers to focus on implementation over rote administration,” he says. “Especially in the context of a continued talent shortage, including data scientists and cyber security experts, the potential for more effective allocation of staff resources is high.”
He adds AI and ML will be key for effectively scaling multi-cloud solutions and enabling organizations to harness their own data estates more quickly.
“Once internal experts have defined factors like data formatting standards, AI/ML can be deployed to implement them in all departments by their respective leaders,” Adya says.
Robinson notes since none of the cloud vendors supports or is likely to support a high level of interoperability, organizations should implement container-based platforms specifically designed for AI/ML -- either from vendors who specialize in providing these platforms or by building their own from open-source components.
Establishing a Cloud Center of Excellence
Leadership on AI/ML integration will inevitably vary for each business and depend on company size, geographic expanse, its industry sector, and core business objectives.
However, Adya recommends that all organizations establish an internal cloud center of excellence (CoE). The cloud CoE should be a cross-functional team of skilled experts, focused primarily on governing cloud usage.
“The cloud CoE should drive AI/ML integration across the four hubs of activities: business, technology, operations and governance by establishing best practices for AI/ML integration and setting organization-wide standards for AI/ML implementation,” Adya says.
McCarthy says when AI/ML is used in a project with a goal to enhance cloud interoperability, the data pipelines should be established by a data engineer, with a data analyst collecting, testing, and presenting the results.
A subject matter expert on the use case’s content should be leveraged to maintain or confirm accuracy.
“Data-heavy projects suffer from scope-creep because the value of the analytics is realized for the first time at the beginning of the project, and everyone wants to add a use case,” he cautions. “Have a well-defined scope and stick to it.”
Robinson notes that many analytics and data science executives will need to take the lead in getting their organizations to implement the hybrid- or multi-cloud MLOps platforms they use to scale their organization’s development and deployment of AI/ML solutions.
“In theory there is a role that AI/ML can play in improving cloud interoperability. For example, solutions that can automatically direct workloads to the environment where it makes the most sense based on physics, cost, and regulation considerations,” he says.
From his perspective, the use of AI/ML to enhance cloud interoperability is of questionable value, because it is difficult to create such solutions. “It’s hard to get the data, hard to build models that would work accurately, and there isn’t a giant benefit over manually allocating workloads across these environments,” he says.
Clearly Defined Goals for Implementation
Adya advises balanced teams, with representatives from key stakeholders across the company, should clearly define goals and priorities for the implementation of AI/ML for cloud interoperability.
“Following implementation of AI/ML solutions, the same group should continue to observe outcomes and results and measure them against measurable KPIs,” he explains. “Staff, including AI team members and ordinary users, must be sensitized to above mentioned KPIs and established best practices, to flag potential issues early.”
He says all organizations that wish to increase their cloud interoperability should investigate investments in AI and ML.
However, the companies that will most likely benefit from AI/ML investment are medium- and large-sized companies that operate across a wide geographic expanse, with multiple cloud platforms, and are looking to meet high legal and cybersecurity requirements.
“AI/ML will ease cloud interoperability, enabling more organizations to leverage the benefits of employing specialized cloud platforms,” Adya notes. “In response, we may see increased cloud platform specialization, enabling companies and vendors to meet business needs more accurately.”
What to Read Next:
Google Cloud Unveils AI Tools to Streamline Preauthorizations
About the Author
You May Also Like