Stopping Identity Fraud Before It OccursStopping Identity Fraud Before It Occurs
A startup says its pattern-recognition software can spot fraudulent credit applications missed by other technologies up to 40% of the time.
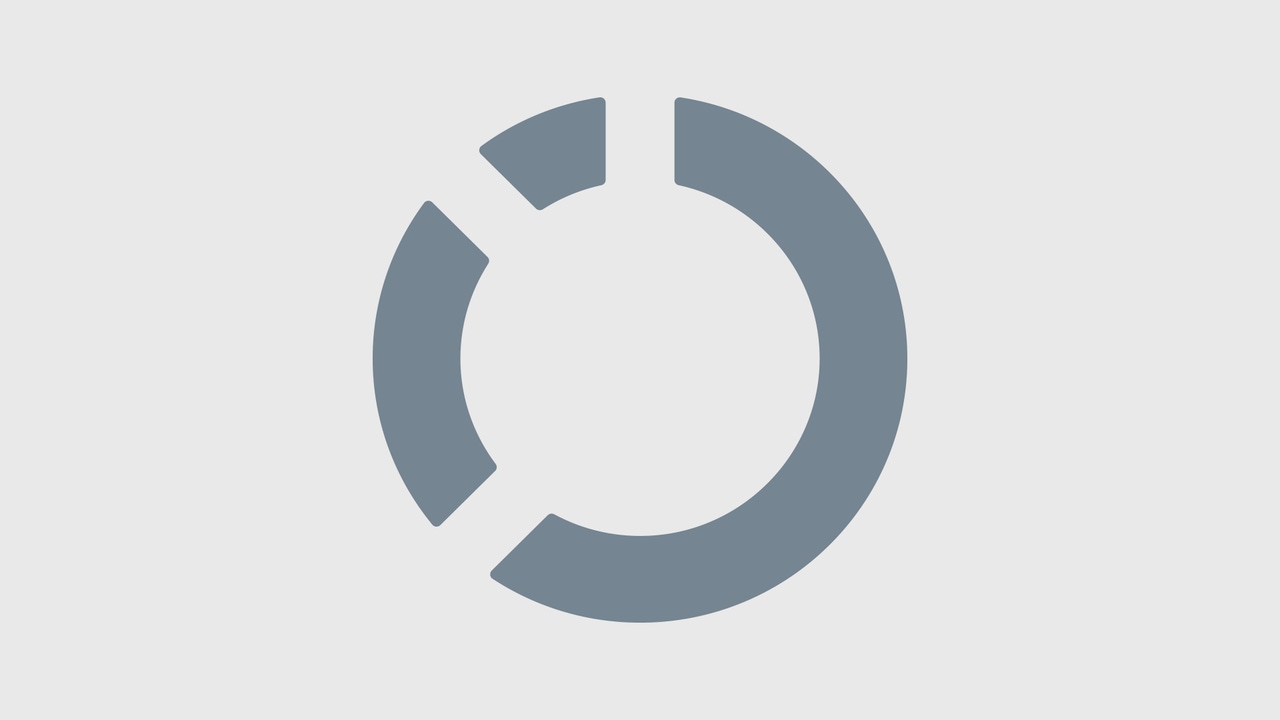
Michael Gray, a former Los Angeles County sheriff who trains detectives to investigate identity theft, says it can take up to 800 hours for consumers to clear their names with stores and credit and financial companies--all just to prove there isn't a $7,000 plasma HDTV sitting in their living room.
It's arguably harder for police to suss out identity theft. Investigators have to wade through complex paper trails and forensic analyses. "If this makes a 5% dent in identity theft, it would be great," Gray says.
All the better, he says, to nip what he calls "a nasty crime" before it occurs. Enter startup ID Analytics Inc., which says its software can spot up to 40% of fraudulent apps missed by current techniques used by financial services companies to spot fraudulent applications before they're approved.
Admittedly, that's not even half of the incidents the company looks at, but damage done by thefts is so big, 40% is still a savings. Identity fraud will cost financial companies $4.2 billion this year and $8 billion by 2006, according to market research firm Financial Insights.
Before founding ID Analytics, its CEO, Bruce Hansen, was president of HNC Software, which made apps for fraud prevention, medical-bill review, and risk and rules management. HNC was bought by Fair, Isaac and Co. Inc. Allen Jost, ID Analytics' chief technology officer, patented fraud-detection scoring while at HNC.
The company says it has studied more than 200 million credit applications--including 10 million known or suspected fraudulent applications--collected by credit-account issuers, retail banks, and wireless-service firms to spot commonalities among phony applications. From this database, the company says it developed pattern-recognition software.
Hansen says analysis revealed that 60% to 80% of fraud losses were originally misclassified as bad debt.
Dennis Behrman, retail financial analyst for Financial Insights, says each company taking an application essentially is working in the vacuum of its own data, unable to spot trails through other companies. It would be helpful, for example, if a bank approving a retail checking account knew that the applicant got cell-phone service just three days before. Or that the same or a very similar Social Security number was used to start three retail charge accounts, a credit card, and establish a new residence at the same time.
It's that kind of activity Jost says his firm tries to spot.
ID Analytics houses the application data on its site, and that data is updated in real time. The company connects over a secure line to a lender's batch or real-time application-processing system. "We do our analysis and give each application an ID Score" in seconds, Jost says.
The ID Score works much the same way as credit-worthiness scores. The higher the score--up to 999--the more likely the application is fraudulent. According to Hansen, roughly 70 variables on credit applications have proven valuable in spotting suspicious activity.
Graph Theoretic Anomaly Detection is the pattern-recognition technique ID Analytics' software uses to create each score. The technique looks for fraudulent "signatures" within an application. Things such as name, address, phone number, and Social Security number are analyzed against the ID Analytics' database. "We can see trends no [individual client] would be able to see looking at their own data," Hansen says.
"I think it's going to significantly change the way we predict fraud," Behrman says.
Police are "reactionary" when it comes to identity fraud, says police trainer Gray. Something that prevents it from occurring saves everyone, except the perpetrator, time and anguish.
One financial-services exec, who asked that his name not be used, has used the service and says, "I can say it works, and it's working better than our initial expectations."
About the Author
You May Also Like