The Case for Decentralized Data ScientistsThe Case for Decentralized Data Scientists
Big data in the enterprise separated subject matter experts from their data. AI is reuniting them, allowing everyone to be a data-scientist, which is ideal for organizational decision making.
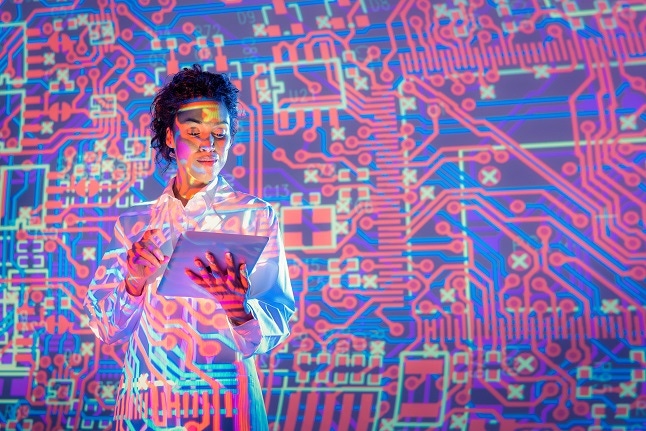
Long before the job title “data scientist” was coined (supposedly at Facebook to recruit talented data analysts), the job description existed, albeit less dressed up. These were folks in the marketing organization who knew how to answer questions about cohort retention by looking at login data, or they were folks in the InfoSec org who could study proxy server logs to figure out which employees posed insider threat risks.
Then came big data and with it, a huge leap forward in what could be known and consequently how data-informed a decision could be. However, not far behind were some unintended consequences. As the volume, velocity and variety of data grew -- your insider threat specialist could now not only analyze much bigger cuts of proxy logs, but also correlate it with badge-swipe data -- the sophistication of tooling for data analysis grew alongside and with it. That led to the need for increased sophistication of analysts.
Enter the data scientist. The mighty Microsoft Excel was able to keep up for a while, but it eventually got displaced by SQL GUIs and Python terminals as the source of truth of enterprise data moved out of spreadsheets and into structured databases. While this was lauded as a big step forward (which it was), one of the unintended consequences was the collateral damage to the ability of subject matter experts like the aforementioned marketing analyst and the infosec analyst to answer questions and make decisions about their business unit as easily as they used to.
Said another way, the technical sophistication needed to leverage big data in the enterprise brought with it a bifurcation of subject-matter expertise and technical expertise. This was as much a product of suddenly insufficient technical competence among the subject matter experts as it was the lack of walk-up usability of the early data analysis tools. Question asking and answering got fissured, with the former remaining in the business units and the latter moving to the emerging data science org.
Those of us who spent time in corporate America in the last 20 years probably are all too familiar with this world and its workflows. You fire off a request to your data team (“What % of users that got served this ad used our new module?”), they send back a few clarifying questions (“Should we exclude users that had been served other ads?”), you refine the ask a little (“Well, as long as the ads were at least a month apart”). They give you an ETA (“We’ll run the job overnight and you should the results tomorrow”) and you eagerly wait for the email from them the next morning with charts or even better, an attached csv.
This works when it works, but sometimes what you get back requires further analysis (“Oh sorry I do actually want to isolate this to users who had only ever been served this ad”), or worse, it isn’t what you asked for because something got lost in translation (“Something seems off … there are more users here than were served the ad … can you double check?”).
None of this is intractable; work can continue to get done. It does, however, take a few more cycles and a little more heartburn. Humans work best when the trial-and-error iteration loop is rapid and intuitive, which isn’t the case when intermediated by a third party with limited context. Multibillion dollar businesses like Palantir (where I spent some time) were created to attempt to close this gap, helping subject matter experts to recreate the rapid intuitive Q&A loop and drive faster and more informed decision-making. They’ve made a ton of progress, but the impact has not been as ubiquitous as it needs to be.
One of the wonderful things about living in a technology-driven world is that just as you start to get comfortable with the status quo, the ground starts to shift under your feet again. That’s the point I think we are at today. AI has popped its head out from the computer science research labs and given non-technical subject matter experts the ability to use their data again. We are in the first paragraph of the first chapter of a voluminous book, so I’m not foolish enough to make predictions. AI-powered tools today are abstracting away the technical complexity of interacting with huge enterprise warehouses and letting subject matter experts ask and answer questions like they could before the big data era.
The emerging modality of interaction is an AI agent that can interact with a business analyst in natural language, convert it into SQL, instantly return answers and charts, iterate on it as the analyst tweaks their ask until what is needed to make the decision is surfaced. Excitingly, the agent can learn from analyst feedback “No, we actually calculate revenue without returns” and begin embedding enterprise knowledge into its analysis for all other analysts at the org.
I’m excited for and optimistic about this undoing of the bifurcation of subject matter expertise and technical expertise; reuniting decision makers with the fodder they need to make decisions and create enterprise value. When everybody is a data-scientist, nobody needs to be.
About the Author
You May Also Like