A New View on IntelligenceA New View on Intelligence
Given the growth of XML and the advantages of EII, more companies will change their view of on-demand intelligence.
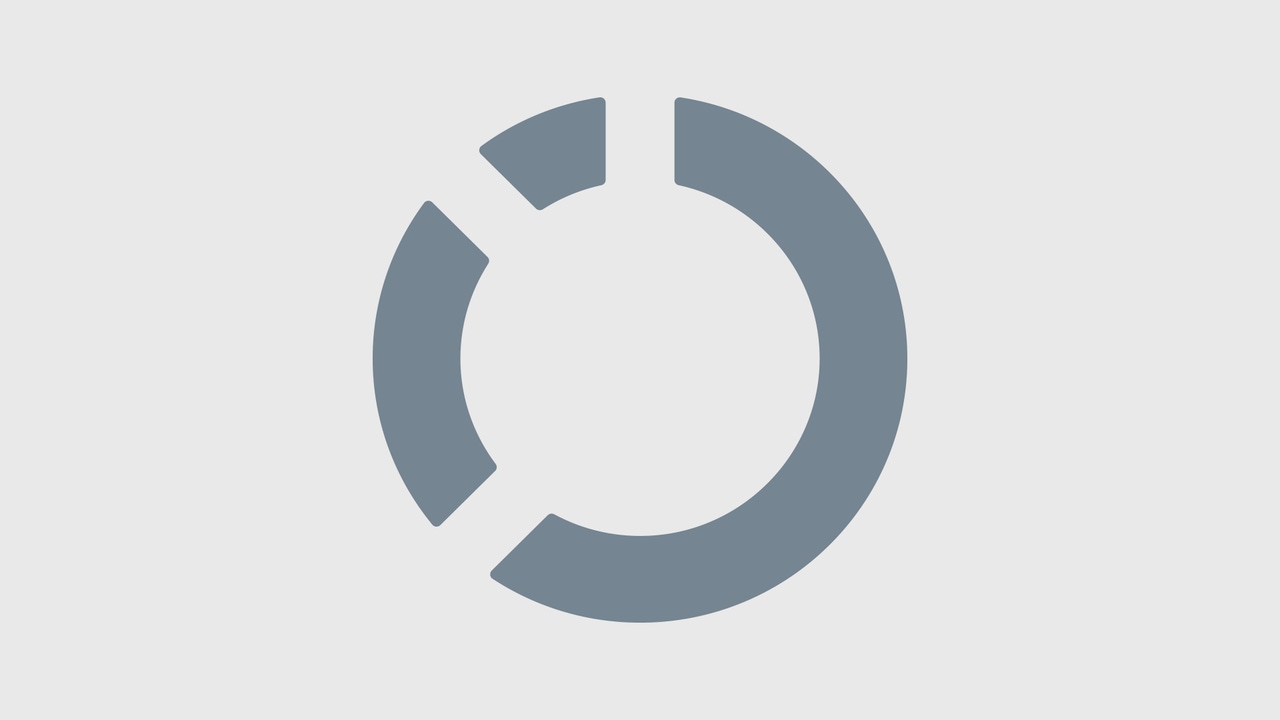
Editor's Note: This month, we present opposing views of the "EII for business intelligence" issue from the vendor community. In the following article, Tim Matthews, co-founder at Ipedo Inc., contends that EII provides the perfect foundation for "on-demand intelligence." To learn about the opposing point of view, read "EII: Dead On Arrival," by Andy Hayler, founder and chief strategist at Kalido, who makes the case that using EII as a business intelligence tool is ill advised.
Enterprise Information Integration (EII) has steadily gained momentum as a must-have tool in the arsenal of data architects. Collecting information from an array of disparate sources and fusing it together in a unified view is just the ticket for a range of applications, including operational dashboards, risk management systems, compliance applications and more.
In addition to the deployment benefits of EII — it's light, flexible, and on demand — there is another key benefit: the ability to gain operational intelligence from non-traditional sources. This ability unlocks powerful advantages that companies have within their information assets, and gives an edge to companies that know how to harvest key information.
The Emergence of EII
What makes EII more than the latest acronym brewed up by vendors and their analysts? Because the technology is relatively new, there are still some skeptics. But the answer is that EII’s time has come, and it is a truly useful technology that fits perfectly with today’s technical landscape and economic climate.
Conceptually, the idea behind EII is quite simple. EII integrates information at the information level. If this definition seems circular, then contrast it with other common approaches. Enterprise Application Integration (EAI) integrates at the application level, moving information from one packaged application to another. Portals integrate at the presentation level, providing a collection of information to users through an integrated Web interface. What's lacking is the ability to collect information from multiple back end data sources — including packaged applications — and make it available to multiple potential user interfaces. EII integrates the information itself into one, unified view. This is not possible with EAI or by using a portal. EII fills the gap.
From a technical viewpoint, the EII approach is also quite different. It uses a distributed query approach to collect and integrate information from multiple sources. This is commonly referred to as federated query. With EII, queries are distributed to data sources and then the results are joined, or federated. This is quite different from other integration technologies. EAI typically passes messages from one application to another over a hub or bus. ETL involves moving data physically from one location to another, creating redundant copies of data in data stores, with their own infrastructure and administration costs. In many cases, the replicated data is summary data, in which case details are no longer available. Basically, EAI and ETL are both push mechanisms. EII is a pull mechanism, where a federated query goes out and finds the data needed by a user application and puts it into user view with context.
Adding Intelligence: The Next Level
What is enticing about EII is the potential to take a powerful additional step. Since the information is fetched and put into a common context, it is possible to add intelligence to the integration. In other words, why stop at integration when the data can deliver real-time intelligence? This is called ‘on-demand intelligence.’
There are many situations where on-demand intelligence could be useful: in operational dashboards, where there is a desire to see how certain functions are performing; in risk management systems — especially in financial institutions — where positions can change trade by trade; in consumer or retail operations, where some hourly metrics could help plan truck routes or even advertising buys.
While this may seem heretical to some data warehouse administrators, fear not. The idea of on-demand intelligence is complementary. First, there will be no replacing OLAP for deep analytical processing. On-demand intelligence allows less complex, but timely and useful, intelligence to be gleaned. Because of this, there is no need to retrofit or adapt an existing data warehouse to perform a task for which it was not created. Finally, an EII tool could access a data warehouse as a source that could be combined with information pulled from other systems, giving new reach and usefulness to the information it contains.
The Role of XML
XML is the perfect ‘information architecture’ for on-demand intelligence because it spans disparate data sources. Everything from Web content to documents, to messages and structured data can be represented in XML. It is also a tagged language: the information elements are surrounded by tags that explain their meaning. Tags specified in XML schema help define specific instances of XML data and give the tags specific meaning for an application, or even for an industry. These tags ease many issues around data meaning and common naming conventions that have plagued data architects for years. Today, major initiatives in almost every industry support standardized XML schema. The FDIC requires all insured banks to report their financials in a format called XBRL (eXtensible Business Reporting Language). The insurance industry mandates that its members exchange policy information in ACORD XML. Wall Street uses an XML format called FpML (Financial Products Markup Language) to trade exotic financial derivatives.
A key component of the architecture is a query language designed for XML. Called XQuery, it is something of a hybrid — a powerful language with grammar similar to SQL, but more widely applicable. It can be used to query any data source that can be represented in an XML model, which includes relational databases, XML document collections, Web Services and a variety of document formats which can have XML equivalents, such as Microsoft Word and Excel. This provides a unified query language for accessing a variety of data sources.
Harvesting Information from Databases, Documents and Messages
With an information model as expansive as XML, organizations can derive useful intelligence from a range of sources. Three in particular are of interest: databases, documents and messages.
The majority of structured information inside of large IT shops is stored in databases, typically relational databases. Representing relational data in XML is quite natural. The flat structure of a relational table barely stretches XML’s capabilities. Since XQuery has all the equivalent constructs as SQL, it has sufficient capabilities to query these sources. In an EII context, this is done by setting up what’s known as an ‘XML View’ over the relational database. The XQuery federator hits the XML View, recognizes the underlying source as a relational database, and translates the XQuery into a SQL query that is passed down to the relational database. Once the SQL query is finished, the results are passed up through the XML View, thus returning the results in XML. So even though the relational database is remote, it appears locally and is queryable through the XML View.
XML documents are even easier to work with. Since these are already in XML form, an XQuery engine simply processes the query directly against a single document or a collection of documents. The query itself can be complex, given the hierarchical and sometimes changing nature of XML. In many cases, organizations have large collections of XML documents. For example, it is not uncommon for mortgage companies, using the MISMO (Mortgage Industry Standards Maintenance Organization) XML industry format, to generate millions of XML documents every year.
There are, of course, many documents inside of a company that are not in XML. These can be converted to XML using a variety of tools. Perhaps most useful, though, are Microsoft Excel 2003 and Microsoft Word 2003. These allow any user to save a report or spreadsheet as XML, which makes lots of useful corporate information available for harvesting.
Messages are just another form of documents. These are likely already in XML form, based on an industry standard, like ACORD for the insurance industry, or FIXML for the financial derivatives market. The key with message processing is often speed of processing, depending on the application. Message flows can be excellent sources of real-time intelligence, be it specific information or aggregates over an hour or a day.
Gathering Intelligence Using Rules and Analysis
We now have a technique for integrating (EII), an information model (XML), and a set of data sources. The next step is to put it all together to gain on-demand intelligence.
We’ll cover two techniques for gaining on-demand intelligence from our databases, documents and messages. The first is rules analysis. This performs various rule checks against these sources to get an immediate picture of business operations — things a risk or operations manager would be interested in. The second is data and content analysis. This is useful for users who want to perform analysis across a variety of sources, which might be used in a planning or reporting function within an organization.
On-demand intelligence can be put into practice in almost any transactional business that has operational data in relational databases, reports in document form, and incoming transactions in message form. For example’s sake, we’ll consider a bank processing loans.
Rules checking via XML is a powerful way to make sure business information adheres to business practices. It checks combinations of XML tags to make sure that values make sense. In our mortgage example, costly operational mistakes could be avoided by checking to make sure <Loan Due Date> is after <Loan Creation Date>, for example. Rules checking via XML should not be confused with XML Schema validation, which only makes sure the syntax is correct — that is, that the document is properly formed and contains all the fields it is supposed to. Rules checking via XML is a semantic check that looks at the values of fields. An operations manager may, for example, want to make sure that loans over a certain value are not done with certain banks. This could be done by checking that <Issuing Bank> is not on a list that is not allowed to loan more than <Loan Amount>.
Banks, like many organizations, have hundreds, even thousands, of these kinds of rules. Performing these kinds of checks on messages coming in can be an immediate source of operational on-demand intelligence. Using XQuery, rather than hard coding in Java or C#, makes them easier to deploy and keep up to date. This is because XQuery is declarative in nature, where you state the result you want to achieve rather than coding how you would go about achieving it. This results in compact code that is easier to read and maintain. The concept can even be extended to pull in reference values from other data sources (EII just for rules checking!).
Performing data and content analysis across information sources is quite straightforward. XQuery is used to search across information sources and discover patterns or trends. In our example, we’ll use collections of loan documents, a relational database with customer information, and approval messages coming in from a partner bank.
A very simple starting point would be to federate a query over the collections of MISMO XML loan documents. It is very easy to write a query that examines the loan documents submitted during a given day and returns the number of normal versus jumbo loans per state. A bank manager could very quickly assess on an intra-day basis where the business is.
Adding another key piece of data, we’ll extend the integration to include information about the customer from a relational database. The idea is to pull the latest info on the customer’s credit score and any purchase history with the loan. Now, for example, not only could we know loans by size and state, but we could also make sure our on-demand intelligence flagged any jumbo loans for customers with poor credit history. This is done by modifying our query to accommodate this.
Adding in the message stream from a partner bank, we could then mine this stream to give the loan officer a heads up display of all similar loans approved or bought by the bank that day. This would be a nice bit of additional information for the user, to help in the decision making process.
The power of on-demand intelligence is the ability to fuse information from disparate systems together, and then provide some additional processing on top. In this way users can get access to the information that they need, as well as the intelligence they need to give them an edge.
New Information Sources, New Intelligence
There is no doubt EII is an important new tool for data architects. It fits right in with and complements existing environments to fill the gap in integration of disparate data sources to end-users. Using EII and an XML data model, organizations can then take the next step to on-demand intelligence and deliver a valuable business advantage to their users.
The future for on-demand intelligence couldn’t be brighter. The availability of information, whether XML Views on relational databases or XML versions of Word and Excel documents, continues to grow inside companies. The number of industries moving to standards based on XML also continues to grow. Many of these were only standards body projects a few years ago. Today, they are in production use by major institutions, and in some cases mandated for use by government regulators or agencies.
Given the growth of XML and the advantages of EII, more companies will change their view of on-demand intelligence. They won’t just want it, they’ll demand it.
Tim Matthews is Co-Founder at Ipedo Inc, a leading vendor of Enterprise Information Management software. He has written extensively on data management and integration in publications such as XML Journal, DevX, and Tech Target, and presented widely on EII.
About the Author
You May Also Like