10 Reasons Why Multi-Agent Architectures Will Supercharge AI10 Reasons Why Multi-Agent Architectures Will Supercharge AI
AI agents are single-tasked AI bots. Several can be orchestrated to work as a team to solve complex problems. GenAI is both the UI and the conductor directing the agents through automation. And that is how the world will change seemingly overnight. Here’s how and why.
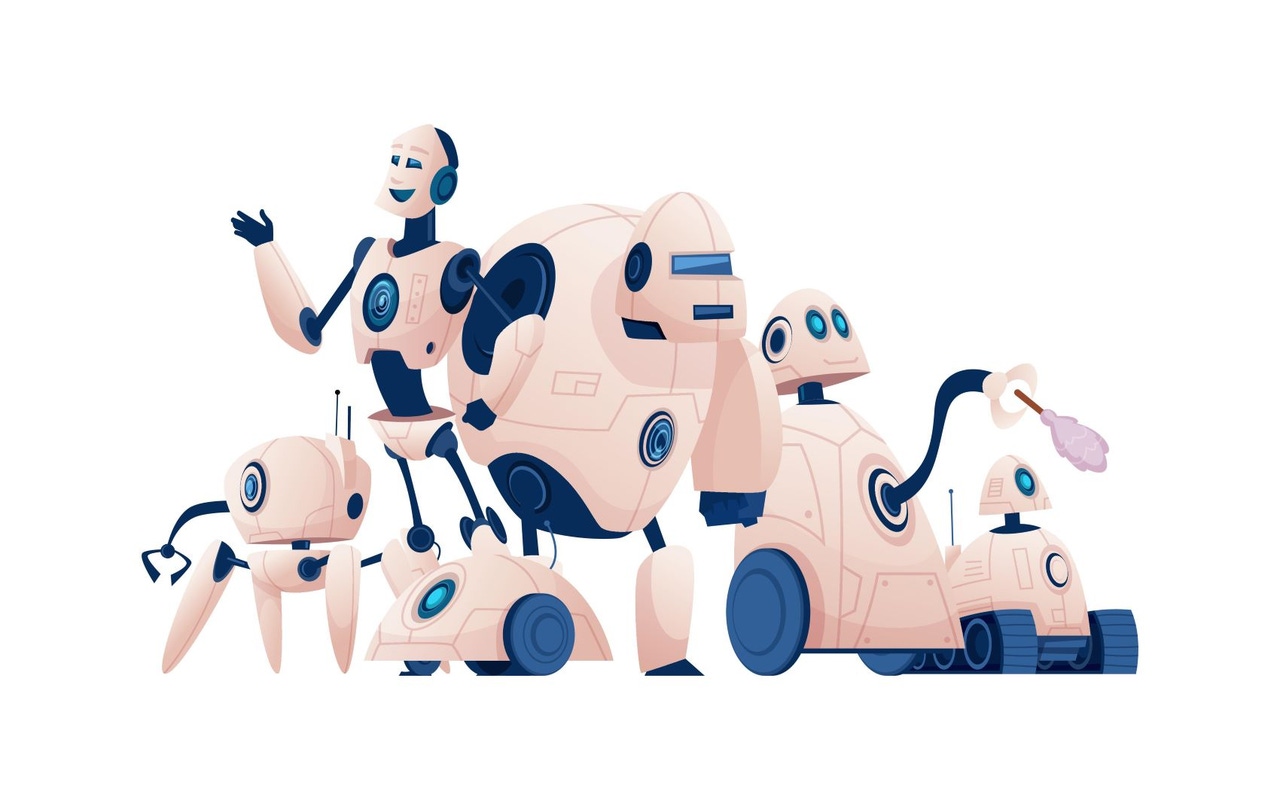
Frustrations with generative artificial intelligence flaws run high and solutions are in high demand. First came the move from large language models to small language models to curb errors through data and task specializations. Then came mini model versions. Now there are highly specialized autonomous AI agents (bots) that work in teams called multi-agent systems.
“This shift leverages specialized AI agents to handle narrow tasks more accurately and cost-effectively, as each agent doesn’t need to be state-of-the-art but simply good enough for its specific role,” says Jesal Gadhia, head of engineering at Thoughtful AI.
The applications for multi-agents appear limitless but they are not a panacea for all AI use cases.
“It’s worth noting that while agents are an important piece of the AI puzzle, they do not replace some LLM-based GenAI tools, nor do they replace predictive AI/ML tools,” says Paul Harmon, senior manager, data science, at Atrium, a consulting firm for data, CRM, and analytics/AI.
“In some cases, AI-based tools may be better used to augment humans, providing insights and recommendations -- in such cases an agent may not be needed. But in cases where AI-driven actions can replace rote tasks, agents can provide some significant productivity gains,” says Harmon, who's also a distinguished member of the American Society for AI.
Ultimately, the concept is to leverage a blend of AI models and data to create a more powerful and targeted mix of tools to handle even the most complex and delicate tasks. For example, consider multi-agents in a customer care scenario for a telco company.
“Because the care agent and network agents leverage different domain-specific contexts, and may also leverage different underlying models and infrastructure, the outcome will theoretically be the ‘best of both worlds’ in terms of accuracy and speed,” says Anthony Goonetilleke, group president, technology and head of strategy at Amdocs, an Israeli multinational telecommunications technology company. “From an end-user perspective, their experience will be more fulfilling as they will not only simplify their care interaction, but also realize a meaningful outcome from the interaction in significantly faster time.”
So much for the high view, let’s now take a closer look at what all of this means to the rest of us:
1. Seismic shift from application to system integrations
Companies are eager to move beyond using GenAI in improving employee productivity to tackle complex use cases but they’re mostly hitting a wall in the process. It won’t be until most or all of an organization’s technology is fully integrated by AI bots that the real magic will occur, let alone at scale.
“Today, the challenge for GenAI is that business operations are integrated, but software systems are not,” says Babak Hodjat, CTO of AI at Cognizant. “Multi-agent architectures create a “system of systems” that allows LLMs to interact with one another. AI agents -- generative AI LLMs wrapped around any software, function, module, or app -- interact with one another within this network, functioning as a virtual working group that can analyze prompts and draw information from across the business. The result? A comprehensive solution not just for the original requestor, but for other teams as well.”
2. Shift of artificial thinking from one agent to a hive mind
GenAI flaws and limitations are glaringly evident. Among them is difficulty in managing complex tasks and long, complicated inputs. Switching from one GenAI tool or “single agent” approach to a “multi-agent” approach instead enables a collection of AIs to each focus on a single task that they can do well.
“The limitations of single agent AI in handling complex, multifaceted tasks have become apparent,” says Loris Degioanni, founder and CTO at real-time cloud security firm, Sysdig. “This inherent limitation has driven a shift towards agentic AI, where multiple agents work collaboratively, much like human teams. This shift is also fueled by advancements in AI technologies that enable more sophisticated coordination and decision-making across agents, and 2024 has become the year when these systems have gained widespread recognition.”
3. Multi-agents already exist but largely haven’t joined forces yet
Use them if you’ve got them, right? Multi-agents are “out there” they just aren’t organized yet in bigger systems. It’s not that doing so is necessarily an easy task, it’s just that it would be a terrible waste of potential if no one bothered to do so.
“In some ways, multi-agent architectures are already here,” says Deon Nicholas, CEO of Forethought AI. “Most systems are leveraging dozens of separate prompts under the hood, so in a sense these systems are already multi-agent. Unlike humans where each human has a distinct separate consciousness, it’s all kind of working in concert ... For example, at Forethought, we have one agent for resolving customer service issues, and a completely separate agent for evaluating the quality of the conversations. This ‘supervisor’ or ‘evaluator’ agent is being used to check the answers of the first, generate further insights, and even provide policy updates for future training of the model. To the customer, it all appears as one cohesive ‘agentic’ system,” Nicolas says.
4. Multi-agent systems won’t replace single-agent applications
The switch to multi-agent systems will not trash any former investments in single agent applications. For example, if your company has already built a GenAI chatbot for HR and another one for customer service, you get to keep using them. Similarly, any investment made by any stakeholder can be protected and bolstered.
“AI can help optimize or create compromise for data gathering -- specifically where satellite vs. drone vs. individually gathered sampling is acceptable or required. It is a complicated space with many views. A multi-agent model can ensure each stakeholder has control over their own reasoning and needs,” says Yvette Kanouff, partner at JC2 Ventures.
Kanouff points to the use of AI in land reclamation for mines, oil, and gas companies as a real-world example.
“This is a complicated space with many views and stakeholders. A gas company will want to focus on compliance of land reclamation and the seed and regrowth of a disturbed site. The state may wish to focus on animal habitat and the larger ecosystem. Then there are longer term considerations such as soil, animal migrations, and population trends. Each of these areas may desire their own reasoning and planning and outcomes. For a multi-stakeholder situation like this, a multi-agent architecture is perfect,” Kanouff adds.
5. Early adopters are already adopting multi-agent architecture
Not only do multi-agent systems exist in primary forms, but early adopters are already adopting them and looking for bigger system siblings.
“This transformation is likely to gain significant momentum in the next 1-2 years, with early adopters already implementing such systems across industries like finance, healthcare, and manufacturing,” says Thoughtful AI’s Gadhia.
The swift uptake is due to more than the momentum started by generative AI tools.
“The multi-agent approach offers increased accuracy, cost efficiency, scalability, and improved reasoning capabilities, allowing enterprises to tackle more sophisticated problems,” Gadhia adds.
6. GenAI can be used as the orchestrator of multi-agents
This concept is relatively straightforward: Use Generative AI as both the user interface and the system orchestrator so that all AI agents work together -- or not -- within a highly flexible framework.
“This creates a powerful ecosystem. Consider the example of underwriting, a process that involves multiple complex steps,” says Ryan Rosett, co-CEO and founder of Credibly, a GenAI-powered lending platform for small businesses.
Here’s how such an ecosystem might work in a real-world scenario.
“In the next wave of enterprise AI architecture, orchestration can be designed such that each agent is tasked with searching for, extracting, interpreting, and analyzing information for a specific step in the underwriting process. These results are then shared with the orchestrator, which reviews the outputs, evaluates the collective feedback, and determines the subsequent actions. The orchestrator can choose to reject findings, conduct further research, or proceed to the next step,” Rosett explains.
7. Multi-agents are a better fit for existing business processes
A key upside to using multi-agent systems is that they are not disruptive to the business. In fact, they naturally fit with existing business structure and processes.
“What’s really interesting about multi-agent systems is how they fit naturally into how businesses already work. Just like companies have different departments or teams handling specific tasks, multi-agent AI mimics this setup -- but does it faster, more precisely, and with less oversight. It doesn’t disrupt the current way things run but instead enhances it, taking care of tasks within the established framework of the organization,” confirms Jyot Singh, founder and CEO at RTS Labs, a boutique technology and engineering consulting company.
8. Multi-agent systems are rolling out already
When generative AI first made a huge splash on the market, companies weren’t sure what to do with it. Use cases were rare beasts and rarely performed as expected. But that isn’t the case with multi-agent systems because no one is looking at those as an all-purpose tool.
“For instance, Salesforce is rolling out Agentforce, which extends their existing generative AI-based Copilot and Prompt Builder from a conversational tool in CRM to more of an automated bot that can do things like handle service cases, help coach sales reps with guided selling, and provide organizations with the ability to create custom AI-based bots (agents),” says Atrium’s Harmon.
9. Many AI agents can help curb the hallucinations of the one
Make no mistake, generative AI will continue to hallucinate. That flaw is inherent to its creative nature and overall human mimicry. To give that truth a bit of dramatic flair, to kill the hallucination is to kill the hallucinator, meaning the GenAI. However, multi-agents pose a compelling way to curb some of its bad behavior.
“You are reducing the number of AI hallucinations through the use of focused AI rather than broadband AI, you are reducing complexity through that same focus and simplicity of the individual AI capabilities in its specialist field, you are essentially creating a team, through that teamwork up into the manager-AI for the decision or report to be made,” explains Mark Molyneux, EMEA CTO at Cohesity.
10. Multi-agents are already giving rise to a digital AI economy
The digital economy is already getting a turbo-boost from AI autonomous agents. So much so that some are already thinking and talking about it as the “digital AI economy.”
“By 2030, we could see a digital AI economy that surpasses the US economy in value,” says Sri Satish Ambati, CEO + co-founder of H2O.ai. “Open-source multi-agent architectures will be the backbone, especially as enterprises move from isolated AI tools to AI systems that act autonomously and collaboratively. The ability of agents to learn from failures and negotiate with other agents will allow businesses to unlock value across industries like never before. The AI transformation isn't coming; it's already started, and multi-agent systems are the next logical step.”
About the Author
You May Also Like