4 Ways to Overcome AI Obstacles4 Ways to Overcome AI Obstacles
Four out of five organizations haven’t scaled their AI. Here are some ways to change that.
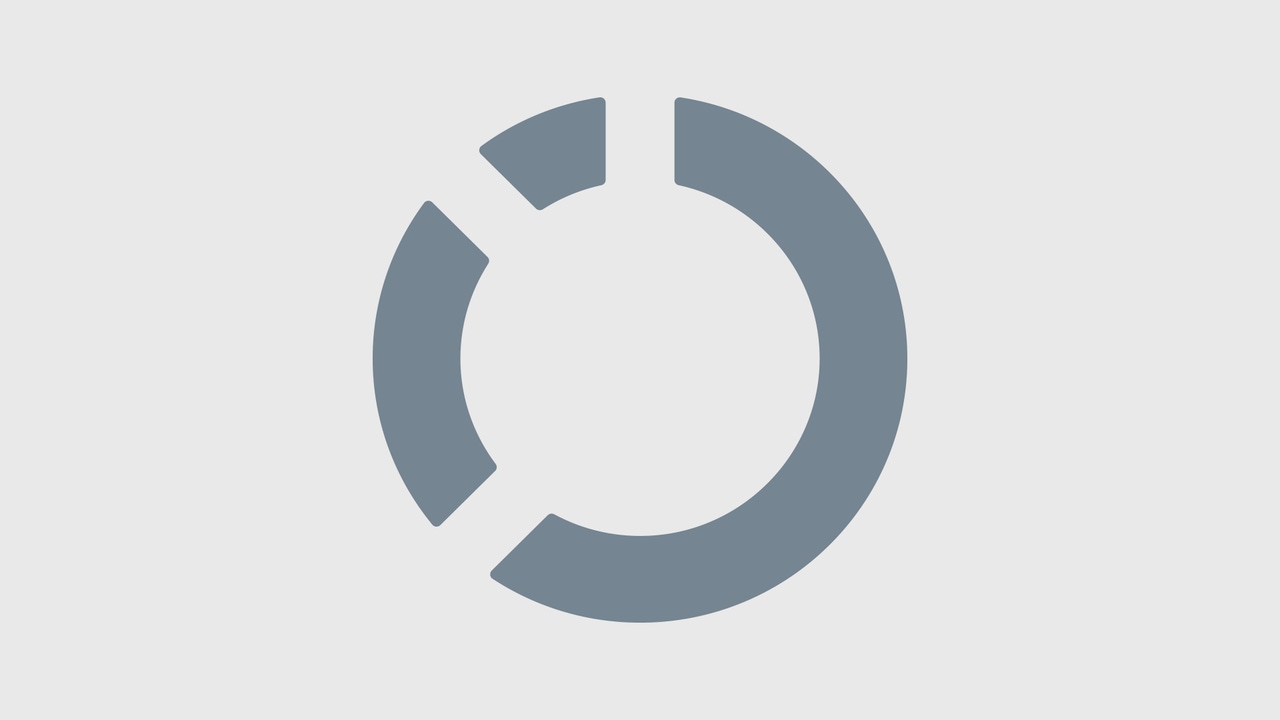
Even as the pandemic tightens technology budgets, there are plenty of companies eager to leverage the highly beneficial capabilities of AI. They hire data scientists, identify use cases, and build proofs of concept. Yet, according to a recent research report from Capgemini, four out of five organizations fail to successfully scale these AI programs from the pilot and initial production stages.
When scaled effectively, AI programs can provide payback that is several times greater than the initial investment, all within the first six months. But without scaling their programs, most organizations aren’t reaping the benefits and showing the value of their AI implementations. This lack of value during difficult economic times results in less additional funding to continue to expand the AI program -- even though the returns could save far more money in the long run.
It’s clear that all companies investing in AI are hoping to maximize its success and capabilities, but other factors are holding them back. Here are four ways organizations can overcome the obstacles that prevent them from scaling their AI programs:
1. Buy-in from leadership
Building AI models is one thing but getting them into production is another. It requires additional resources, including the right people and architecture to support it (more on that in a bit). One thing working against AI deployments is that there is a lack of support among executive leadership given the number of steps and investment needed to execute effectively to achieve the highly beneficial end results. AI teams must prioritize demonstrating the value of their programs and showing accurate forecasts for the future benefits to get buy-in from leadership to keep pushing forward and scaling these initiatives.
2. The right people and skillsets
For companies to successfully get their AI models into production, they’ll need more than just data scientists on staff. Data engineers must build the pipelines, and machine learning (ML) engineers are needed to get models in production. Companies also will need business analysts to capture the insights from the data and translate the numbers into relevant takeaways for the business. Organizations that only invest in bringing data scientists on board will have a difficult time getting their AI programs to scale.
3. Technology
To get AI models into production and start running operations, companies will need the technology and architecture to support them. This includes everything from setting up environments to develop models that easily integrate with code repositories, to creating docker containers and setting up continuous integration (CI) triggers to rebuild docker images of ML steps. Then, teams can execute the pipelines to deploy the models to production (CD).
4. Operating model
In many cases, data scientists and engineers are scattered throughout an organization, aligning with specific IT or business functions. This is practical in theory, but it also creates silos, with these AI employees lacking visibility and connection with their counterparts across the company, creating a ‘my model culture’. Organizations must create an AI-centric operating model. In our organization, we refer to it as the AI Center of Excellence. The Center of Excellence takes care of the end-to-end life cycle of AI projects, ensuring that they get from concept to completion -- or in AI terms, from pilot to production to scale. Most companies lack an operating model that is structured for AI program success.
The benefits of AI are clear for those who have harnessed the ability to capture them. Getting in position to capitalize on this revolutionary technology capability takes time, effort, and investment, but the rewards can significantly outweigh the initial work to get there. Organizations that earn buy-in from leadership, hire the right talent and skillsets, implement the proper technology architecture, and coordinate the proper operating model to execute will overcome the most common pitfalls of AI scalability.
{image 2}
Dan Simion leads the AI & Analytics practice for Capgemini North America. He has more than 25 years of experience in data science, advanced analytics, and technology-enabled applications and solutions. Dan’s focus areas are artificial intelligence and machine learning, and his publications include "Marketing Analytics Capabilities," "Harnessing the Power of Private Label," and "Systems and Tools to Track Marketing Effectiveness.”
About the Author
You May Also Like