5 Big Data Myths, Busted5 Big Data Myths, Busted
Be right, not fast. Think business, not IT. Don't worry about dirty data. A big data guru shares contrarian advice about the worst whoppers.
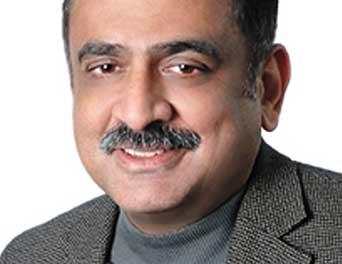
Myth 4: Big companies are so diverse, it's impossible to agree on big data projects.
Here's where you need to look for common denominators. The most obvious example is usually the customer. In its work with British Airways and its Know Me loyalty program, Opera Solutions helped the airline see the connections across nine systems silos that each held data related to the customer experience.
"Just making a connection between the baggage-claim system and the loyalty system helped the airline take the simple step of sending an apology letter when a bag is lost, where previously they couldn't," says Gupta.
Customers aren't the only connection point among otherwise disparate data sets. Products, suppliers, and partners can also be axes of big data integration and insight. Here again, the advice is to find a focal point.
Myth 5: Big data demands data scientists, who are expensive and hard to come by.
Opera Solutions has studied data scientists' practices and has found that 80 percent of their efforts involve finding the signal in the noise -- that is, the time-consuming work is in capturing data and finding the patterns therein. But this is information management work that can be done by information management professionals, not data scientists. As for the remaining 20 percent of work that does require data science expertise -- the choosing of algorithms and statistical methods -- companies have to focus on making these choices repeatable.
Time-series analyses, for example, show up in many big data projects, including marketing optimization, trading and financial services, route optimization, inventory forecasting, and many more. Constructing a time-series analysis for the first time -- the combining of datasets that will feed the analytic system -- may require a lot of time-consuming upfront data management work. But the actual data science work -- determining the right algorithms and analysis techniques -- is not nearly as laborious or time-consuming.
The main point is that once you've done something once, it can be automated and repeatable, according to Gupta. "The mistake people make is starting all over again with each new project. You have to create a repeatable process or it will never scale."
So don't think of big data initiatives as requiring a colony of hard-to-find, expensive data scientists. Create a repeatable process and embed the intelligence gained along the way into software. That way your data science needs will start small and get smaller as you gain experience.
There's no single migration path to the next generation of enterprise communications and collaboration systems and services, and Enterprise Connect delivers what you need to evaluate all the options. Register today and learn about the full range of platforms, services and applications that comprise modern communications and collaboration systems. Register with code MPIWK and save $200 on the entire event and Tuesday-Thursday conference passes or for a Free Expo pass. It happens in Orlando, Fla., March 17-19.
About the Author
You May Also Like