5 Data Science Sins To Beware5 Data Science Sins To Beware
Repent, ye data scientists! Avoid these five big data evils -- or pay with your immortal soul.
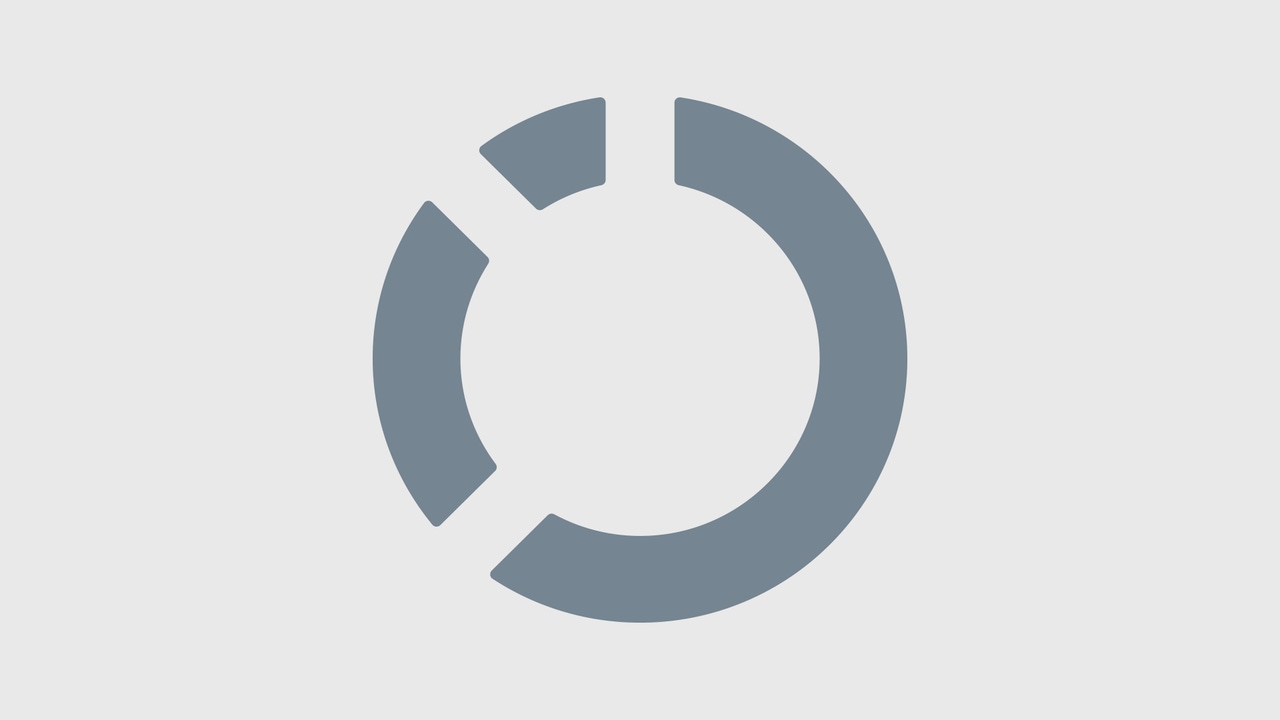
OK, perhaps our fire-and-brimstone headline goes a bit overboard. Then again, maybe it is time for a dose of data science atonement, particularly if you're guilty of any of the five deadly sins summarized below.
According to Michael Walker, founder and president of the nonprofit Data Science Association, a professional organization of data scientists with more than 500 members, these big-data sins are all too common. In fact, the Association's recently penned Code of Professional Conduct is designed to establish a set of ethical standards for the burgeoning data-science industry.
Not all big-data professionals are guilty of the five deadly sins, of course, which Walker summarized in a phone interview with information. So here they are. Do any of these data-science transgressions hit home?
Sin #1: Cherry Picking
This is where a data scientist includes only data that confirms a particular position and ignores evidence of a contradictory position. "I see this all the time," Walker said.
[ For more on ethical best practices for big-data professionals, see Data Scientists Create Code Of Professional Conduct. ]
Cherry picking is all too common in university research, according to Walker, who referenced a 2005 paper, "Why Most Published Research Findings are False," by Stanford professor John Ioannidis. "What [Ioannidis] argues, in a nutshell, is that the overwhelming majority of research that he reviewed could not be replicated," said Walker.
Here's a hypothetical scenario that illustrates cherry picking in action:
"[Researchers] create a hypothesis they want to test out," Walker said. "So they run it 999 times, and it fails. There's no evidence to confirm their hypothesis. Then they tweak it, run it again, and all of a sudden they find evidence to confirm their hypothesis." But when these same researchers publish a paper proclaiming their success, they don't mention the 999 times they failed. "I think that's very unethical," Walker said.
Sin #2: Confirmation Bias
This is where researchers favor data that confirms their hypothesis.
"When you're dealing with very large data sets, you're going to find more relationships, more correlations," said Walker. And that can lead to causation confusion, especially in high causal density environments. In other words, a lot of different variables could be the cause of something.
A lot of data scientists are under pressure to produce results that favor their employer's or client's hypothesis, Walker pointed out, a situation that can lead to inaccurate, misleading or just plain wrong data analysis.
Sin #3: Data Selection Bias
"This means the skewing of data sources," Walker said. "A lot of times [data scientists] fool themselves in this regard."
How so? By measuring only data that's available. "Oftentimes, what's most valuable or most appropriate for you to be looking at is data that just isn't available yet," said Walker. "And that can really skew the results of the science."
When examining big-data research, it's always important to ask this key question: Who is paying for the data science? "Whoever's paying for it will probably want to skew the data to favor their interests," Walker added.
Sin #4: Narrative Fallacy
"A lot of data scientists feel the need to fit a story into connected or disconnected fact," said Walker. "So they come up with a story, and then they go looking for data that they can plausibly interpret to fit that story."
Real data science doesn't -- or shouldn't -- work that way. So what's the right approach?
"You have a hypothesis, you collect the data, you run experiments … and then you let the chips fall where they may," Walker said. "And you interpret them according to the scientific method, and give [your findings] to the decision makers."
Sin #5: Cognitive Bias
This is where you're skewing data to suit your prior beliefs rather than relying on the evidence.
"This is very dangerous, yet I see it all the time," Walker said. "It's human nature. We all have prior beliefs. We all have biases, even though the best of us try to recognize them and control for it."
In short, data scientists need to focus more on the evidence. "We need to really look at the data to get the facts and the evidence out of it, so that we can make better decisions," he said.
Walker himself 'fesses up to sometimes falling into these data science traps. "I see things I thought were true, and then I see evidence they're not. I was wrong about the way I thought about something," he said. "We need to be humble and look at the evidence. And we need to train people to do that more."
Emerging software tools now make analytics feasible -- and cost-effective -- for most companies. Also in the Brave The Big Data Wave issue of information: Have doubts about NoSQL consistency? Meet Kyle Kingsbury's Call Me Maybe project. (Free registration required.)
About the Author
You May Also Like