7 Things That Need to Happen to Make GenAI Useful to Business7 Things That Need to Happen to Make GenAI Useful to Business
Generative artificial intelligence is falling victim to hype -- and so are the companies that invested in it. Here’s what needs to change to turn the situation around.
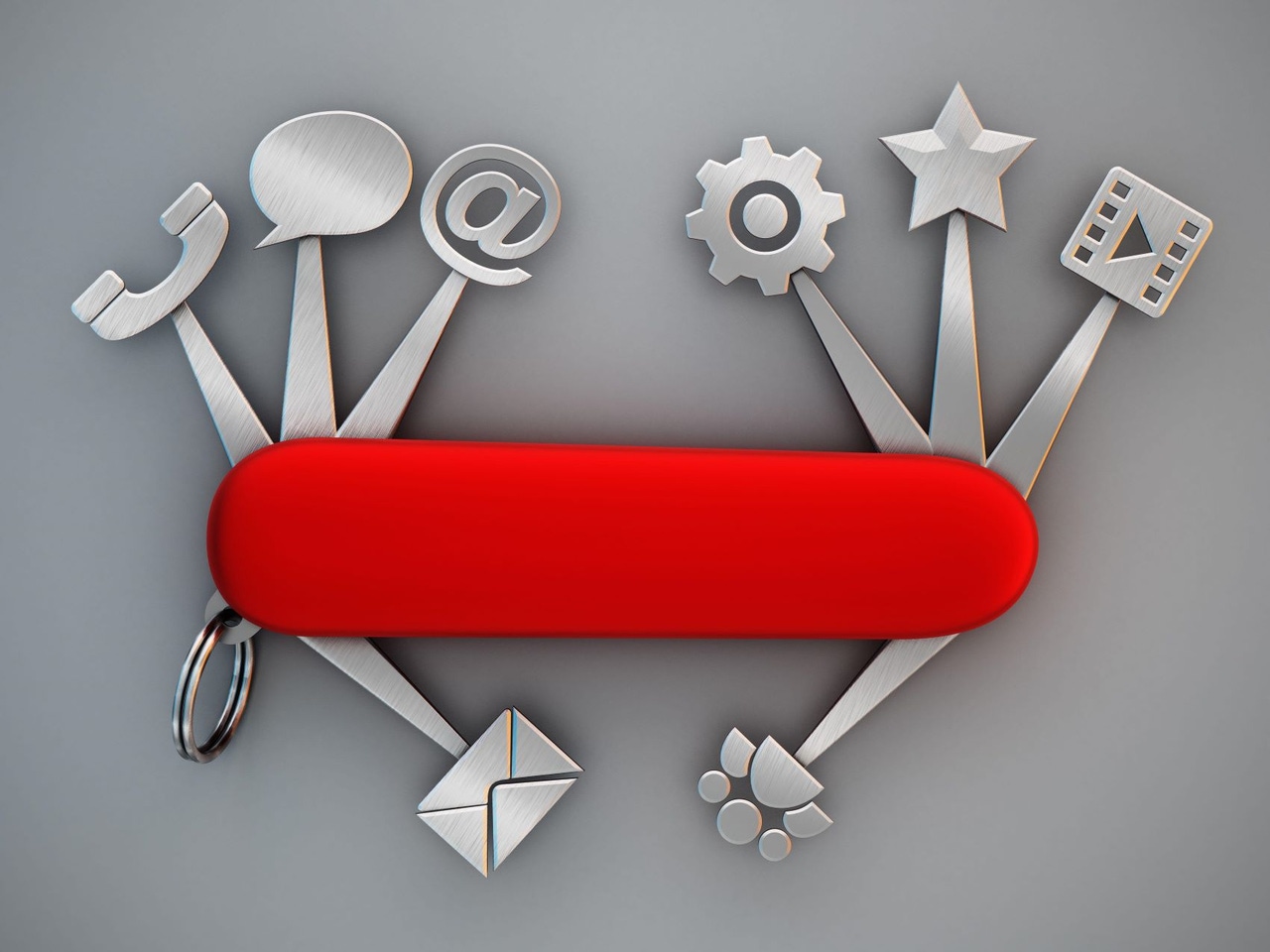
Generative AI is one of the hottest trends of the century. And also, the most disappointing trend for many companies.
“Productizing GenAI has proven to be incredibly illusive. Commercials for AI services insist you must adopt AI in your business processes in order to remain competitive. Meanwhile, the difficulties in actually productizing GenAI are now starting to have tangible effects on financial markets, as tech companies struggle to justify their capital expenditures on AI hardware and infrastructure. So, what gives?” says Sohrob Kazerounian, distinguished AI researcher at Vectra AI.
Some blame exaggerated hype for GenAI’s failings.
“It was inevitable that generative AI would eventually dip down into the trough of disillusionment -- no technology can fulfill that level of hype,” says Saurabh Abhyankar, EVP and chief product officer at MicroStrategy.
Others think the soar-to-crash cycle is common to new technologies where vendors over-promise and customer expectations are not pinned to reality.
“The disillusionment has occurred because many enterprises had inflated expectations from many of the great demos and hype. They thought these models would be as accurate as a spreadsheet or database and that they would be able to incorporate their own data easily. Many enterprises didn’t actually define success criteria for GenAI; enterprises report that 90% of proof of concept projects never make it into production,” says Naveen Rao, vice president of AI at Databricks.
But perhaps more to the point is the fact that far too many organizations have found far too few use cases that GenAI can do.
“GenAI is not a technological Swiss army knife, so to see value, enterprises must focus on what it does well, which is understanding and generating natural language within context,” Abhyankar says.
Even so, most companies stumble on being far too deep in the investment well to stop drinking from it now.
“Firstly, let’s remember that transformative technologies often stumble before they sprint, like you say. Consider the early days of the internet or even electricity -- initially dismissed, then indispensable,” says Lars Nyman, the chief marketing officer of CUDO Compute, a platform that supplies the computational horsepower behind many of today’s breakthrough AI programs.
The good news is that GenAI is not a complete loss -- and likely not a loss at all -- if a few measures are now put in place. Here’s what needs to change to turn the situation around:
1. The chase for more models and features stops
Losing interest in more models and features sounds counterintuitive but it actually isn’t. Organizations need time to learn the models and features that exist now to see clearly how they can be leveraged. The rush to constantly upgrade before you get your bearings borders on insanity from a purely business, capture- the-ROI perspective.
“There is an obsession over the glamour of data models and the instinct to constantly fine-tune ML algorithms to chase that elusive ‘perfect’ model for GenAI apps. But here’s the thing: This model-centric approach is fundamentally flawed because it causes data teams to overlook the foundation of effective ML -- the data itself,” warns Kevin Hu, the co-founder and CEO of Metaplane and an MIT PhD researcher in machine learning and data visualization.
Get your data right first. Know what you have and define what you need. Then pick a model and features accordingly. In most cases, you’ll find you need more than one AI model so that the combined capabilities perform to your expectations or goals.
2. GenAI breaks its mold
GenAI tools are marvelous. They make so much that’s pretty and so little that’s practical. And one big reason this happens is that despite the heft of the data and the might of the model, GenAI applications largely function as isolated standalones.
“Although GenAI as a standalone application has been intriguing, for it to take hold in the shorter term, the odds are good that it will happen due to the network effect of existing mega platforms: Google, Meta, Amazon, Apple, and the like,” says Eli Goodman, CEO and co-founder of Datos, a global clickstream data provider and a Semrush Company.
“GenAI’s real hook is its integration as a value-enhancing complement to existing everyday products and services. Whether it be Google AI-assisted search, Amazon shopping assistant Rufus, or Apple’s newly launched AI features in the iPhone 16, successful adoption within these already massive consumer systems is where GenAI will transform from exception to rule,” Goodman adds.
GenAI is also expected to become both the UI and the conductor in multi-agent architectures.
3. GenAI shows its true age
GenAI is not new. It just seems like it is because of how OpenAI’s ChatGPT made such an unexpected splash in the market and how fast everyone then jumped on it and other applications. Certainly, OpenAI created new models and ChatGPT was and is an impressive model, and other vendors and open-source projects have followed suit with their own creations. Even so, this AI category is established tech for the most part.
But in all that time, little progress has been made in establishing what to do with it.
“Many companies treat GenAI as a science fair experiment,” says Scott Gnau, vice president of data platforms at InterSystems, a provider of AI-enabled data management solutions for healthcare, financial services, and supply chain and logistics. “Doing it for the sake of doing it because everyone else is and they don’t want to be left behind. They never break out of the theoretical into the practical. Simply deploying GenAI without a clear strategy or measurable objectives will only lead to more roadblocks and further disillusionment.”
Once everyone stops gawking at GenAI like it’s a new toy and sees it as a fairly mature tech among other even more mature forms of AI, then organizations can begin to match capabilities and use cases on a far more sensible basis.
4. Companies begin to routinely break down their strategies and tasks
GenAI applications are typically based on large language models (LLMs) and built for general purposes. People have the impression that all the Internet data was dumped in it, and therefore any answer to any problem can be extracted. Oh, how magical! That leads to businesses treating the tech like it can do anything on demand. Well, it can. But it does it pretty badly more often than not.
“For organizations feeling disillusioned by AI investments, the answer lies not in throwing more resources at developing custom models, but in refining their data and problem breakdown strategies. Success depends on how well the problem is structured for the LLM and the data it’s fed -- not on creating bigger, better models,” says Yuval Perlov, chief technology officer at K2View, a data integration company.
It turns out that breaking down tasks is smart use of AI as it enables you to get better performance out of things like autonomous AI agents, multi-agent architectures, specialized models (like those in ChatGPT’s GPT store), mini models, and other small language models.
5. Data labeling happens at the source
On average, most companies do a lousy job of sorting and labeling data. This throws a huge wrench in the works whether its AI projects or more mundane work like advanced analytics or automation. Fix the data and all will be well everywhere. Standardizing how and when data is labeled will cure a lot of those ills.
“Unfortunately, most enterprises are applying data catalogs to define, label and map data to semantic concepts after data has flowed through several other steps and transformations. What they should be doing instead is definition and mapping data right from the source so that any person or program accessing the data downstream has a shared definition of it,” says Eric Best, CEO at SoundCommerce, a provider of a composable data platform for retail brands.
6. GenAI is widely viewed as augmented work
If you’re looking at GenAI as automation to replace all or most of your employees, you’re in for a bad case of sticker shock. It can’t. It won’t. This is not THE use case for GenAI.
“It’s often hyped as being able to do things that it doesn’t do well. An example is writing. Sure, it can write, but we can’t just let it go [off on its own],” says Phil Libin, former CEO of tech unicorn Evernote and current founder of the AI startup studio All Turtles, and its product mmhmm. “My basic philosophy here is that AI should be used in places where it can help someone be better at their job, even if it takes longer. If you are looking to replace a job with AI, that’s a waste of time. In fact, if you’re going to do that, then you shouldn’t be doing [or filling] that job in the first place.”
7. GenAI goes green
It’s no secret that GenAI, like all other forms of AI, are huge energy hogs. According to a report in Nature, estimates are that GenAI-driven search like Perplexity AI, Bing with Copilot, and Google with Overviews, uses four to five times the energy of search without AI. Further, large AI systems are likely to need as much energy as entire nations and will need enormous amounts of fresh water to cool their processors and to generate the electricity they consume.
“The technology needs to mature in terms of its energy efficiency and computing costs, making it viable for widespread use, not just in tech-centric companies but across industries. This is where innovations in distributed computing and quantum processing could eventually play a transformative role,” says Scott Dylan, founder of NexaTech Ventures, which focuses on early-stage and growth-stage AI startups.
About the Author
You May Also Like