A Path from Pilot to Machine Learning ProductionA Path from Pilot to Machine Learning Production
Getting machine learning from the pilot into production is a hot issue in 2019. MLOps is one of the ways enterprises are doing it.
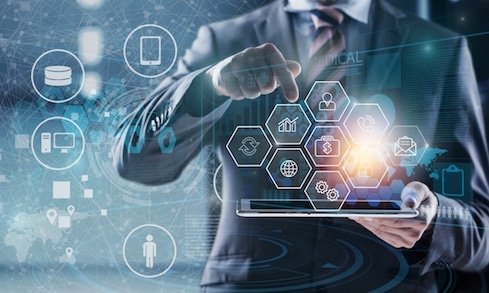
Inside IT organizations, getting machine learning technologies from pilot into production is one of the hot topics of 2019. At this point, many organizations have run successful pilots. Yet many more have still haven't achieved the value promised by machine learning because it isn't integrated into organizational processes.
A Gartner study showed that only 47% of machine learning models are making it into production. Organizations are employing a few different methods to get their machine learning investments to production. One way is by employing systems integrators, who may have more experience with getting models to work with particular enterprise software or processes. Another way may be implementing an MLOps practice.
The name MLOps, inspired by the DevOps practice and philosophy, is an approach to put machine learning into operations. DevOps itself has many definitions, depending on who you talk to, but overall it is about making software development work better for everyone by enabling collaboration among multiple groups, incorporating Agile-type processes, and making use of feedback for new iterations of the work.
MLOps follows a similar path, but for implementing machine learning in organizations. The practice has been gaining in enterprises, and many of its followers congregated at the recent MLOps NYC conference. Microsoft's head of open source machine learning strategy, David Aronchick, was among several experts who presented at that conference. He also spoke with information about the state of MLOps in the enterprise today.
"Getting machine learning into production is the No. 1 problem," Aronchick told information. "That's why there are initiatives around MLOps. That's exactly what it is designed for."
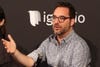
David Aronchick Image: Joao-Pierre Ruth
Organizations have been dabbling in machine learning for a while, according to Aronchick, and it's gotten easier to stand up a pilot project. Today, machine learning pros can get onto GitHub and download models to jumpstart the effort. But they may quickly learn that their pilot is not really ready for prime time.
"It takes forever to get [machine learning] out and add it to your app or your software development effort," he said. "The enterprise has not kept pace."
MLOps brings data scientists into the software development process. There are open source tools to help with this process, Aronchick said. Amazon offers a set of such tools. He said Microsoft has been also working to offer a rich set of hosted tools for MLOps on Microsoft Azure to help organizations with their machine learning pipeline. The first of these were unveiled in December 2018 when Microsoft released machine learning as a service on Azure. In May the company announced the MLOps extension to Azure DevOps. So far Microsoft's services include hosted training, a machine learning model management service, automated testing and drift analysis, and help with rolling out to production, among other services.
For enterprises, the challenges are still about integrating these kinds of tools into the organization's operations.
"We've made great progress," Aronchick said.
Beyond systems integrators and MLOps, enterprises may also want to try some of their traditional methods for implementing IT projects.
For instance, Gartner VP and analyst Eric Brethenoux recommended an old IT "battle tested" analytical model development cycle during the Gartner Data Conference in March. First, select use cases, then prioritize those use cases, then stack rank those use cases.
Core skills and job titles for your operationalization team will include source system experts, system architects, system administrators, application developers, and process engineers, he said.
Read these related stories:
Takeaway from MLOps NYC: Open Source Frameworks Need TLC
Getting Machine Learning into Production: MLOps
A Practical Guide to DevOps: It's Not that Scary
AI, Machine Learning, Data Science: What Enterprises Are Doing
About the Author
You May Also Like