A Robot That Can Throw Bananas? Why CIOs Should Take NoticeA Robot That Can Throw Bananas? Why CIOs Should Take Notice
Scientists are now developing models that allow robots to learn from experience. Pay attention, because deep learning is poised to drive significant advances.
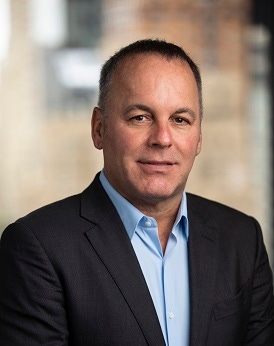
Robots don’t often make the news -- humans are still far better at that -- but the March 26 ‘TossingBot” announcement was an exception. As shown in this video, researchers at Google, Princeton, Columbia, and MIT developed a robot that can learn to grasp and throw random objects like bananas and ping pong balls into boxes outside its pre-coded natural range and improve performance through self-learning.
“This robot, like many others, is designed to tolerate the dynamics of the unstructured world,” Google student researcher Andy Zeng blogged. “But instead of just tolerating dynamics, can robots learn to use them advantageously, developing an ‘intuition’ of physics that would allow them to complete tasks more efficiently?” Through deep learning, he wrote, “our robots can learn from experience rather than rely on manual case-by-case engineering.”
The announcement is interesting because it illustrates the extent to which deep learning is poised to drive significant real-world advances well beyond TossingBot’s playful purview. As robotic technology grows more sophisticated, it is increasingly set to transform a variety of industries, including manufacturing, healthcare, transportation, and agriculture. And CIOs and other IT leaders are paying attention.
Robotic process automation (RPA) is one of the top 10 technologies for business-transformation-minded CIOs in 2019, according to a KPMG report.
As Forrester put it in a report, “Automation technologies such as artificial intelligence, robotic process automation, and physical robots give CIOs the chance to help their company rethink its business model and drive customer obsession. Rather than seeing automation as a cost-cutting move, customer-obsessed CIOs consider these technologies tools for reshaping customer experiences.”
While teaching a robot to perform tasks based on a pre-stated set of instructions is as old as robotics itself, the ability for robots to learn progressively from layers of data and train themselves based on deep learning algorithms is truly futuristic.
It may be a stretch to say that deep learning in robots -- the ability to learn by example rather than through task-specific instructions -- has reached human-like intelligence yet. The reality is the technology isn’t that mature yet, but it certainly has earned comparisons with animal intelligence in its ability to recognize patterns and adapt to the environment on the fly.
Think of it this way: If TossingBot can replicate human-being movement by understanding how to throw random objects into a box -- accounting for variables such as differences in shape, size, mass, and developing its own throwing strategies through trial and error -- it still may not be as intelligent as you but is as smart as your cat.
The notion of self-teaching robots may bring to mind HAL, the computer-run-amok in the blockbuster movie “2001: A Space Odyssey,” but examples of the technology’s beneficial applications abound.
Take agriculture for example. In mid-March, a federal jury in San Francisco unanimously declared that the herbicide Roundup was a "substantial factor" in causing a man’s non-Hodgkins lymphoma. The verdict was the second in the U.S. since August to find a connection between Roundup’s main ingredient glyphosate, and cancer.
Pesticides are pervasive in our food because the standard practice today is to routinely coat entire fields of crops with them. However, deep learning robots can navigate around a farm all day, quickly determine which plants are healthy and which needs pesticides, and spray only the ones that need them. This approach is a radically more efficient use of pesticides that greatly reduces the amounts used and reduces unnecessary spillage. In fact, the Small Robot Company in the UK offers a set of robots doing exactly that.
Other areas where these smarter robots can make an impact include construction, especially in situations such as a skyscraper building where wind and other conditions can present challenging variables; manufacturing, where they can be more precise in detecting flaws; healthcare, as a virtual assistant to surgeons; and in disaster response scenarios where every second counts.
Make no mistake: This is not easy. Programming deep learning for robotics is difficult for a variety of reasons -- primarily involving data volume, data speed, and computational limitations. CIOs and other IT leaders must keep this in mind before dedicating precious resources to it. However, TossingBot shows scientists are developing cutting-edge models that allow robots to learn from experience rather than a programmer’s instructions.
Expect to keep seeing headlines trumpeting robot advances. The number of publicly available deep learning datasets, such as these, keeps growing, helping experts break through technical barriers in robotic mapping and navigation, which require extreme levels of real-time information gathering, processing, and execution.
There’s more help from the Robot Operating System (ROS), a set of software libraries and tools aimed at simplifying robot development. And Amazon's Deep Racer is an autonomous driving development platform that lets developers explore machine learning methods that can handle very complex behaviors.
CIOs and other IT leaders who think deep-learning robots can help them should cultivate extreme curiosity about the technology and experiment with the freely available models and tutorials out there. You don’t necessarily need to be an expert in deep learning or someone who has been in the field for many years. Deep learning is still in its infancy and in a way, we are all students.
Tom Canning is VP of devices and IoT at Canonical Group, the developer of the open source OS Ubuntu. Prior to joining Canonical in 2017, Canning held several senior sales positions in the UK and the U.S., including at HP, Cisco and, most recently, Spigit. He is based in London and holds an electrical engineering degree from the University of Ottawa.
About the Author
You May Also Like