How AI and IoT Are Reshaping AgricultureHow AI and IoT Are Reshaping Agriculture
Agriculture is quite literally the foundation of civilization. Agriculture 4.0 is important to the future of civilization.
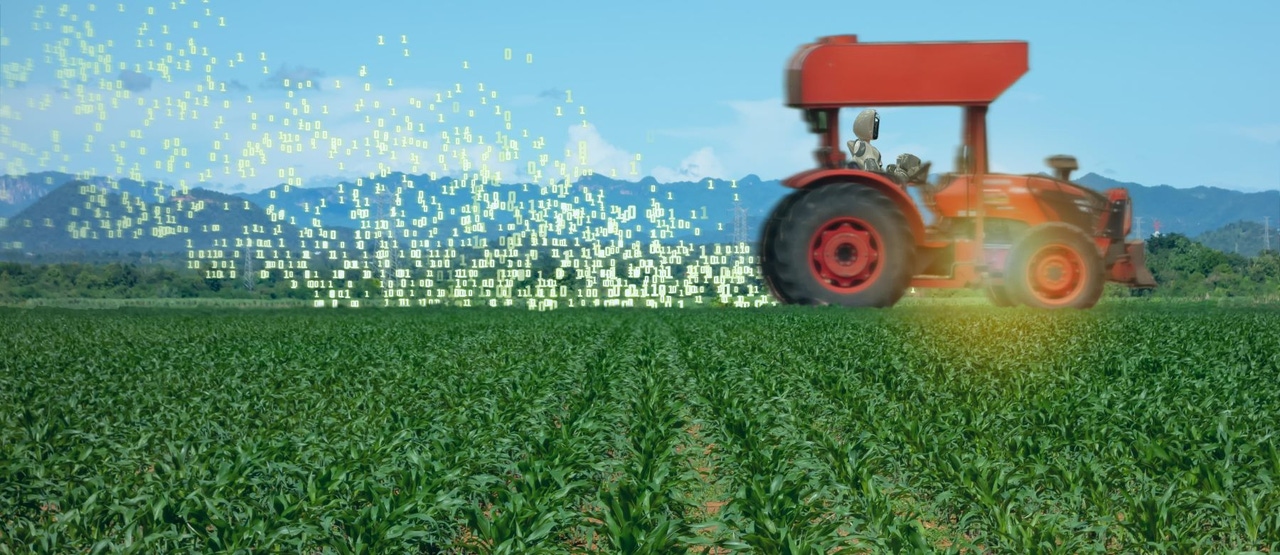
Agriculture -- the cultivation of domestic plants and animals -- is believed to have begun in earnest some 12,000 years ago in the so-called Fertile Crescent of the Middle East. The management and harvest of plants likely began much earlier, but the techniques developed in this region allowed humans to form large settlements that developed into the complex, urban centers that now define our species. This was the first of a series of agricultural revolutions.
The increasing sophistication of agriculture in the ensuing centuries has supported an ever-burgeoning population. A second agricultural revolution began in Britain in the 17th century and included the introduction of new irrigation techniques, fertilizers, and means of transporting agricultural products. And the projected population collapse of the 20th century was averted by the Green Revolution, or third agricultural revolution, beginning in the 1940s, which saw huge increases in crop yields due to new fertilizers and pesticides.
Now, a fourth revolution is in the offing. Technological advancements including deployment of the Internet of Things (IoT) -- digitally enabled devices that collect and transmit data -- and artificial intelligence are creating new efficiencies that are likely to again fundamentally change the practice that has allowed our species to dominate the planet.
Gathering centuries worth of historical data and integrating it with new information collected by novel devices will allow farmers to radically refine their planting, watering, pest management, and harvesting strategies -- leading to increases in yields and decreases in detrimental environmental effects. There are already nearly 100 million connected devices in use by farmers today and that number will only grow as the people who feed us continue to digitize their operations.
Here, information investigates the rise of IoT and AI in farming operations, with insights from Lisa Avvocato, vice president of global marketing for AI and computer vision data annotation company Sama, Mike Flaxman, vice president of product at big data analytics company HEAVY.AI, and Valeria Kogan, founder and CEO of Fermata, which offers AI solutions for monitoring plant health.
(Editor's note: This feature story covers AI's contributions to agriculture. However, AI also competes with agriculture, for resources like land and water. These issues will be covered in more depth in upcoming information sustainability coverage.)
Meeting the Needs of a Growing Population
The global population today exceeds 8.1 billion. That number will increase by a projected 2 billion in the next 30 years.
In a time of increasing drought driven by climate change, the rising costs of fuel, more stringent environmental regulations, the prevalence of tenuous monocultures, and onslaughts of invasive pest species, the harrowing question of how the world’s exploding population will be sustained again rears its head. While birth rates are dropping worldwide, the overall population continues to grow -- and it is hungry.
The production of food has so far kept pace with the increase despite shortfalls in the developing world. According to the United Nations, in 2022, 2.4 billion people experienced some level of food insecurity.
And growth rates have declined in the last decade. Production will need to increase by as much as 110% in order to feed the coming generations according to some estimates.
Farmers will find themselves increasingly reliant on digital technology to manage their crops and boost their yields. The innovations of the Green Revolution alone are no longer sufficient to meet the exponential increases in demand. Now, farmers are using some sort of digital assistance on more than 50% of the acreage devoted to growing corn, cotton, rice, sorghum, soybeans, and winter wheat in the U.S. -- and those who are not will need to adopt these technologies quickly if they are to compete. So-called precision agriculture appears to be the only way forward.
The Basics of AI and IoT Technology in Agriculture
AI’s use in farming has slowly accelerated since the first publications on the topic in the 1970s and 1980s. Research took off in the early 2000s, in line with other AI applications. Now, the collection and analysis of big data, both historical and contemporary, is creating major efficiencies for growers of nearly every imaginable crop.
Data on humidity, prevalence of pests, rainfall, soil moisture, and temperature is collected using a wide variety of technologies. Broad data sets can be drawn from geographic information systems. And more specific data can be collected by unmanned ground vehicles (UGVs) and unmanned aerial vehicles (UAVs) equipped with specialized red, green and blue (RGB), light detection and ranging (LIDAR), thermal, multispectral and hyperspectral cameras as well as by stationary wireless sensors. It is then analyzed by machine learning and deep learning programs.
“That data would be completely overwhelming if it weren't for AI being able to organize it,” Flaxman explains. “You have terabytes of data, but you need a way to filter through the boring stuff. You need to be able to monitor routine conditions, but you also need to be able to catch exceptional conditions.”
The interaction of varying types of data can lead to more exacting analysis, he adds. “There’s an ability now to do what the military has done for years, which is what they call tip and cue. The tip part is when you're surveilling, and you see something that's suspicious. That cues you to use another resource to figure out what’s going on. The technology is getting really good at that tip-and-cue rhythm where you have some technology used for wide area surveillance and then some detailed technology you bring in to figure out what’s really going on when there’s a problem.”
The technology has gotten to the point where users can pose natural language queries to these programs and get a visual representation of the answer -- map depicting certain qualities for example -- in return, he notes.
Some of the same technologies used to collect the data can actually implement solutions too -- a variety of robotic technologies including UGVs and UAVs equipped with visual detection technology can identify and remove weeds and spray pesticides in targeted areas, for example. Seeds can be planted at ideal depths by UGVs. These devices, with the addition of robotic attachments, can also be used to harvest produce with greater exactitude than other mechanized technologies by using image recognition, resulting in fewer damaged fruits and vegetables.
Synthesis of Historical and Contemporary Data
Farmers have been recording data for thousands of years -- since the beginning of written language. The Sumerians began recording their crop yields as early as 3500 BCE. In the United States, nationwide agricultural statistics have been collected since 1863 -- that year the US Department of Agriculture established a Division of Statistics.
AI models can leverage the information documented by our ancestors and make predictions about factors that are crucial to agriculture today -- particularly climatic factors like heat and rainfall and the availability of native resources such as groundwater and soil nutrients.
This data can then be integrated with information gathered by IoT sensors, UAVs and UGVs and observations from satellites such as the American Landsat system and European Sentinel-2 to generate even more specific and useful forecasts. Indeed, this is why these new technologies are collectively referred to as precision agriculture.
.jpg?width=700&auto=webp&quality=80&disable=upscale)
Mike Flaxman, HEAVY.AI
“AI is really good at cleaning up dirty data,” Flaxman says. “Water sensors get stuck. Aerial imagery captures clouds. Every data source, every sensor has its little challenges.” AI programs filter out the static and generalize the most useful information.
Decision support systems (DSS) designed specifically for farms known as farm management information systems (FMIS) can help to integrate these various information sources and generate usable recommendations for planning of planting, management, harvest, and sale using machine learning technology.
These recommendations can reduce costs, lead to greater yields and contribute to more sustainable use of pesticides, fertilizer and water. They can also help farmers to anticipate market demands and pricing and plan accordingly, leading to higher profits and less waste.
Several key applications of IoT and AI have been identified.
Management of Water Resources
AI has proven particularly useful in determining when and how to irrigate crops. Liquid Prep, a product developed by IBM, uses data on water retention from soil sensors integrated with data from weather forecasts and data on the water needs of particular plants in order to provide recommendations on when a particular field should be irrigated and how much should be administered. The recommendations are made available on an app.
Other systems integrate data on evaporation, humidity, soil and ambient temperature as well as satellite and drone data that can indicate early signs of drought stress.
“The resolution of all those sensors is increasing,” Flaxman says of satellite data. “The resolution has a spatial component that people are used to, but it also has, very importantly for agriculture, spectral resolution. If you think more like a honeybee, honeybees clue in on flowers because they can see a lot further into the infrared than humans can. When vegetation is under drought stress, you can see it in those infrared bands weeks before the human eye can see it. Those weeks are precious, because that's enough time to give you the ability to deploy a countermeasure.”
AI assessment of the exact water needs of different areas of a farm, which have distinct terrain and soil parameters, can ensure plants receive optimal levels of moisture -- neither overwatered nor underwatered. This in turn can lead to better absorption of fertilizers and reduce the incidence of pests that thrive under overly damp or overly dry conditions.
Runoff, which in addition to being wasteful, carries contaminants into the water supply, is also reduced. The management of water will become increasingly important as droughts increase, groundwater resources are depleted, and the quality of arable land decreases over time. As it is, less than 1% of the world’s freshwater is available for use; some 70% of groundwater is used for irrigation.
In addition to managing current crops, the analysis of these types of data can be utilized by AI programs to assess which types of crops might be more efficiently grown under future conditions. AI has identified drought-tolerant varieties of soybean, for example. One study even aims to develop microbes that can mitigate the effects of drought on soybeans in part using AI analysis.
Detection and Mitigation of Weeds and Pests
Weed, pests, and disease pressure can lead to significant crop losses. The early detection of these problems, before they damage crops beyond repair, is crucial for the maintenance of profitable and sustainable agricultural operations.
AI can be highly effective in analyzing aerial images taken by drones and satellites for subtle spectral changes that indicate the early onset of disease or a pest infestation. Even a decade ago, sugar beet diseases could be detected by AI analysis with accuracy of up to 90%.
“AI is trained on high-quality datasets of thousands of examples of different pests and diseases for various plants. In our products, we use deep learning and neural networks to analyze the visual data and identify pests and diseases,” Kogan says. “Normally, people called scouts walk through the greenhouse or fields and look at every single leaf of every single plant to identify the abnormalities. Of course, this is very hard work; scouts miss things and eventually, 30% of harvests are lost on average due to late reaction to pests and diseases.”
With AI detection, areas that are affected can be rapidly identified and treated with appropriate pesticides while unaffected areas can be monitored, thus reducing both resource use and environmental contamination. With AI identification, Avvocato says, “you only spray quadrant one. In the past, you were spraying pesticides across your entire field.”
Detecting weeds, which may outcompete crops, is also crucial. They take essential nutrients and water resources from the desired plants, reducing vitality and yields. Farmers spend some $26 billion on herbicides annually in the US to address the problem. In some cases, this may amount to nearly two-thirds of their annual expenses.
The use of AI technology allows for weeds to be targeted precisely, without affecting nearby plants or requiring the aerial broadcast of herbicidal compounds.
“Crops and weeds can look very, very similar, especially in the early stages,” Avvocato says. “One of the challenges is getting enough high-quality images, because these crops and weeds look so similar. We need the image quality to be able to define whether something is a weed that we need to spray a pesticide on, versus the early stage of a crop.”
_(002).jpg?width=700&auto=webp&quality=80&disable=upscale)
Lisa Avvocato, Sama
One project found that a camera affixed to an all-terrain vehicle could run through fields and identify weeds, to which herbicide was then applied directly with rates of precision of up to 78%. Another used deep learning to identify weeds with up to 98% accuracy, with the goal of removing them using non-chemical means. Targeted mechanical disturbance by tines or the application of sparks that essentially fry the plant have been used. More radically, the LaserWeeder uses deep learning and robotic technology to identify weeds and destroy them with lasers.
AI can also be used to detect the emergence of disease in livestock. Cameras can be used to detect signs of pathogens in fish farms, for example. And smart collars can monitor heart rate, respiration, and other vital signs of mammals, thus detecting indications of ill health before they become acute.
Soil Conditions and Planting
In addition to monitoring soil moisture and drainage, AI can assist in analyzing its nutrient content, composition, and texture using data gathered from IoT devices and historical sources. Classifying soil types and how they align with the needs of various crops can assist farmers in deciding which species, and specific varieties, to plant, how deep seeds should be planted, and how they should be spaced.
AI programs can also refine the selection of fertilizers and other soil amendments by identifying deficiencies and aligning them with the needs of the plants and predict pathogens and pests that favor specific soil conditions.
Monitoring Growth and Managing Harvests
AI technology can further assist farmers by monitoring plant growth and comparing it to known growth patterns to estimate the health and maturity of crops and their yields under various circumstances.
Researchers have used deep learning to monitor the ripeness of strawberries with accuracy of up to 99%, for example, thus ensuring that they are harvested at exactly the right time and increasing customer satisfaction with the product. Another program used AI to detect grapes as they formed, identifying the bunches with an accuracy of 75% and enabling vintners to estimate their harvest early in the season.
AI combined with IoT technology can also be used to conduct the harvest itself. One study used image recognition combined with a robotic arm to successfully pick ripe tomatoes 89% of the time. And produce can be sorted by quality once harvested using image recognition techniques. One research team sorted hazelnuts according to size and level of damage with accuracy of up to 96%.
Factors such as quality, size, and storage life can be aligned with market prices and competition using AI analysis too, allowing users to predict potential buyers and likely income.
Limitations and Challenges
While AI analysis of major crops is generally advanced, the availability and accuracy of programs for less common ones is uneven, detecting anomalies requires extensive tuning of AI detection programs.
“The quality of data still remains a huge challenge due to the absence of ground truth -- you have to rely on agronomists' opinions on the images as you can’t do a lab test for every single diagnosis,” Kogan says.
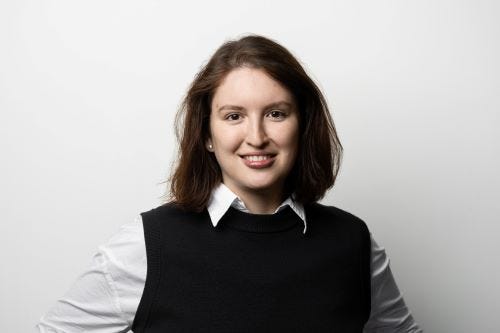
Valeria Kogan, Fermata
“We tend to have better mapping for things that are aerially extensive. Things that are smaller are poorly covered,” Flaxman adds.
Thus, the technology may not even yet be available for some plants. “That’ll be the next major push in agriculture. It will basically help this technology get special crop specifics, which is what it needs to be. Nobody grows general crops, right?” Flaxman says. “That’s an area where the mapping is going to get better. If you grow strawberries, and you’re mapping your strawberries, you’re going to do a better job at that than a government agency has ever done just because it’s in your interest.”
While IoT and AI technologies have shown great promise in assisting farmers with their operations, they are not yet available to independent farmers on a wide scale. Though some projects attempt to deploy them in developing countries and some small-scale farmers have managed to utilize them in targeted applications, they are largely the province of Big Ag at this point. Integrating the various technologies and data sets into actionable information -- and then actually acting on it -- is a challenge that requires substantial investment before it can pay off.
Even one application -- say, management of irrigation -- requires massive amounts of data and programs that can manage it. And each piece of technology deployed is only capable of harvesting particular types of data in the first place.
“The biggest barrier to entry is spending the money to buy that infrastructure,” Avvocato says. “It can add up quite quickly.”
FMISs have shown promise in putting more generalized insights from publicly available data into practice but flying drones over smaller operations to spot localized instances of disease and installing sensors to monitor soil moisture remain out of reach to many. Further, gathering data at a single point in time has limited utility. Granular, localized data -- as opposed to broader trends gathered from historical observations -- becomes more useful as it accrues over longer periods.
Flaxman notes that services are available that can conduct aerial surveys for farmers who cannot afford the equipment on their own. There are even cooperative programs in which neighboring farmers can collaborate on surveys and share the resulting data amongst themselves. He suggests combining this data with other, freely available satellite data and analyzing it using subscription web services and open-source tools as a start.
Training programs may be useful in equipping growers -- especially in food-stressed regions in the developing world-- to use IoT and AI to improve their operations as the technology accelerates and more comprehensive platforms become available.
“There's a lot more food instability there than in the US,” Avvocato says. “Being able to increase crop production 10, 20 or 30% can have a dramatic impact.”
In the meantime, the flights of drones and armies of sensors scanning the fields of larger operations will continue to fuel the data-hungry AI programs that are refining the art and science of feeding our species.
Read more about:
Internet of Things (IoT)About the Author
You May Also Like