AI and the War Against Plastic WasteAI and the War Against Plastic Waste
Plastic waste is one of today’s most complex environmental challenges, and people are putting AI to work to understand it and solve it.
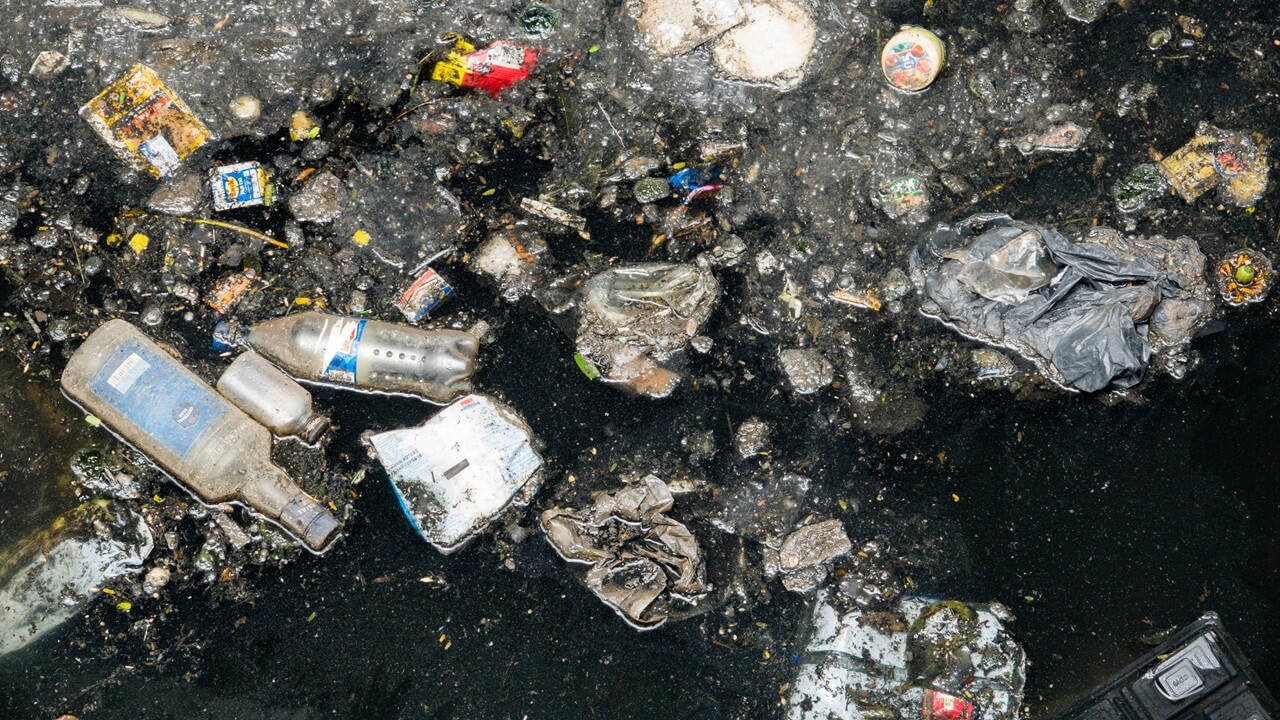
Plastic pollution is easy to visualize given that many rivers are choked with such waste and the oceans are littered with it. The Great Pacific Garbage Patch, a massive collection of plastic and other debris, is an infamous result of plastics proliferation. Even if you don’t live near a body of water to see the problem firsthand, you’re unlikely to walk far without seeing some piece of plastic crushed underfoot. But untangling this problem is anything but easy.
Enter artificial intelligence, which is being applied to many complex problems that include plastics pollution. information spoke to research scientists and startup founders about why plastics waste is such a complicated challenge and how they use AI in their work.
The Plastics Problem
Plastic is ubiquitous today as food packaging, clothing, medical devices, cars, and so much more rely on this material. “Since 1950, nearly 10 billion metric tons of plastic has been produced, and over half of that was just in the last 20 years. So, it's been this extremely prolific growth in production and use. It's partially due to just the absolute versatility of plastic,” Chase Brewster, project scientist at Benioff Ocean Science Laboratory, a center for marine conservation at the University of California, Santa Barbara, says.
Plastic isn’t biodegradable and recycling is imperfect. As more plastic is produced and more of it is wasted, much of that waste ends up back in the environment, polluting land and water as it breaks down into microplastics and nanoplastics.
Even when plastic products end up at waste management facilities, processing them is not simple. “A lot of people think of plastic as just plastic,” Bradley Sutliff, a former National Institute of Standards and Technology (NIST) researcher, says. In reality, there are many different complex polymers that fall under the plastics umbrella. Recycle and reuse isn’t just a matter of sorting; it’s a chemistry problem, too. Not every type of plastic can be mixed and processed into a recycled material.
Plastic is undeniably convenient as a low-cost material used almost everywhere. It takes major shifts in behavior to reduce its consumption, a change that is not always feasible.
Virgin plastic is cheaper than recycled plastic, which means companies are more likely to use the former. In turn, consumers are faced with the same economic choice, if they even have one.
There is no one single answer to solving this environmental crisis. “Plastic pollution is an economic, technical, educational, and behavioral problem,” Joel Tasche, co-CEO and cofounder of CleanHub, a company focused on collecting plastic waste, says in an email interview.
So, how can AI arm organizations, policymakers, and people with the information and solutions to combat plastic pollution?
AI and Quantifying Plastic Waste
The problem of plastic waste is not new, but the sheer volume makes it difficult to gather the granular data necessary to truly understand the challenge and develop actionable solutions.
“If you look at the … body of research on plastic pollution, especially in the marine environment, there is a large gap in terms of actually in situ collected data,” says Brewster.
The Benioff Ocean Science Laboratory is working to change that through the Clean Currents Coalition, which focuses on removing plastic waste from rivers before it has the chance to enter the ocean. The Coalition is partnered with local organizations in nine different countries, representing a diverse group of river systems, to remove and analyze plastic pollution.
“We started looking into what artificial intelligence can do to help us to collect that more fine data that can … help drive our upstream action to reduce plastic production and plastic leaking into the environment in the first place,” says Brewster.
The project is developing a machine learning model with hardware and software components. A web cam is positioned above the conveyor belts of large trash wheels used to collect plastic waste in rivers. Those cameras count and categorize trash as it is pulled from the river.
This system “… automatically [sends] that to the cloud, to a data set, visualizing that on a dashboard that can actively tell us what types of trash are coming out of the river and at what rate,” Brewster explains. “We have this huge data set from all over the world, collected synchronously over three years during the same time period, very diverse cultures, communities, river sizes, river geomorphologies.”
That data can be leveraged to gain more insight into what kinds of plastic end up in rivers, which flow to our oceans, and to inform targeted strategies for prevention and cleanup.
AI and Waste Management
Very little plastic is actually recycled; just 5% with some being combusted and the majority ends up in landfills. Waste management plants face the challenge of sorting through a massive influx of material, some recyclable and some not. And, of course, plastic is not one uniform group that can easily be processed into reusable material.
AI and imaging equipment are being put to work in waste management facilities to tackle the complex job of sorting much more efficiently.
During Sutliff’s time with NIST, a US government agency focused on industrial competitiveness, he worked with a team to explore how AI could make recycling less expensive.
Waste management facilities can use near-visible infrared light (NIR) to visualize and sort plastics. Sutliff and his team looked to improve this approach with machine learning.
“Our thought was that the computer might be a lot better at distinguishing which plastic is which if you … teach it,” he says. “You can get a pretty good prediction of things like density and crystallinity by using near infrared light if you train your models correctly.”
The results of that work show promise, and Sutliff released the code to NIST’s GitHub page. More accurate sorting can help waste management facilities monetize more recyclable materials, rather than incinerate them, send them to landfills, or potentially leak them back into the environment.
“Recyclers are based off of sorting plastics and then selling them to companies that will use them. And obviously, the company buying them wants to know exactly what they're getting. So, the better the recyclers can sort it, the more profitable it is,” Sutliff says.
There are other organizations working with waste collectors to improve sorting and identification. CleanHub, for example, developed a track-and-trace process. Waste collectors take photos and upload them to its AI-powered app.
The app creates an audit trail, and machine learning predicts the composition and weight of the collected bags of trash. “We focus on collecting both recyclable and non-recyclable plastics, directing recyclables back into the economy and converting non-recyclables into alternative fuels through co-processing, which minimizes environmental impact compared to traditional incineration,” explains Tasche.
Greyparrot is an AI waste analytics company that started out by partnering with about a dozen recycling plants around the world, gathering a global data set to power its platform. Today, that platform provides facilities with insights into more than 89 different waste categories. Greyparrot’s analyzers sit above the conveyor belts in waste management facilities, capturing images and sharing AI-powered insights. The latest generation of these analyzers is made of recyclable materials.
“If a given plant processes 10 tons or 15 tons of waste per day … that accumulates to around like 20 million objects. We actually are looking at individually all those 20 million objects moving at two to three to four meters a second, very high-speed in real time,” says Ambarish Mitra, co-founder of Greyparrot. “We are not only doing classification of the objects, which goes through a waste flow, we are [also] doing financial value extraction.”
The more capable waste management facilities are of sorting and monetizing the plastic that flows into their operations, the more competitive the market for recycled materials can become.
“The entire waste and recycling industry is in constant competition with the virgin material market. Everything that either lowers cost or increases the quality of the output product is a step towards a circular economy,” says Tasche.
AI and a Policy Approach
Plastic waste is a problem with global stakes, and policymakers are paying attention. In 2022, the United Nations announced plans to create an international legally binding agreement to end plastic pollution. The treaty is currently going through negotiations, with another session slated to begin in November.
Scientists at the Benioff Ocean Science Laboratory and Eric and Wendy Schmidt Center for Data Science & Environment at UC Berkeley developed the Global Plastics AI Policy Tool with the intention of understanding how different high-level policies could reduce plastic waste.
“This is a real opportunity to actually quantify or estimate what the impact of some of the highest priority policies that are on the table for the treaty [is] going to be,” says Neil Nathan, a project scientist at the Benioff Ocean Science Laboratory.
Of the 175 nations that agreed to create the global treaty to end plastic pollution, 60 have agreed to reach that goal by 2040.
“Ending plastic pollution by 2040 … seems like an incredibly ambitious goal. Is that even possible?” asks Nathan. “One of the biggest findings for us is that … it actually is close to possible.”
The AI tool leverages historic plastic consumption data, global trade data, and population data. Machine learning algorithms, such as Random Forest, uncover historical patterns in plastic consumption and waste and project how those patterns could change in the future.
The team behind the tool has been tracking the policies up for discussion throughout the treaty negotiation process to evaluate which could have the biggest impact on outcomes like mismanaged waste, incinerated waste, and landfill waste.
Nathan offers the example of a minimum recycled content mandate. “This is essentially requiring that new products are made with a certain percentage, in this case 40%, of post-consumer recycled content. This alone actually will reduce plastic mismanaged waste leaking into [the] environment by over 50%,” he says.
“It’s been a really wonderful experience engaging with the plastic treaty, going into the United Nations meetings, working with delegates, putting this in their hands and … seeing … them being able to visualize the data and actually understanding the impact … of these policies,” Nathan adds.
AI and Product Development
How could AI impact plastic waste further upstream? Data collected and analyzed by AI systems could change how CPG companies produce plastic goods before they ever end up in the hands of consumers, waste facilities, and the environment.
For example, data gathered at waste management facilities can give product manufacturers insight into how their goods are actually being recycled, or not. “No two waste plants are identical,” Mitra points out. “If your product gets recycled in plant A, doesn't mean you'll get recycled in plant B.”
That insight could show companies where changes need to be made in order to make their products more recyclable.
Companies could increasingly be driven to make those kinds of changes by government policy, like the European Union’s Extended Producer Responsibility (EPR) policies, as well as their own ESG goals.
“Millions of dollars [go] into packaging design. So, whatever will come out in ‘25 or ‘26, it's already designed, and whatever is being thought [of] for ‘26 and ’27, it's in R&D today,” says Mitra. “[Companies] definitely have a large appetite to learn from this and improve their packaging design to make it more recyclable rather than just experimenting with material without knowing how will they actually go through these mechanical sorting environments.”
In addition to optimizing the production of plastic products and packaging for recyclability, AI can hunt for viable alternatives; novel materials discovery is a promising AI application. As it sifts through vast repositories of data, AI might bring to light a material that has economic viability and less environmental impact than plastic.
Plastic has a long lifecycle, persisting for decades or even longer after it is produced. AI is being applied to every point of that lifecycle: from creation, to consumer use, to garbage and recycling cans, to waste management facilities, and to its environmental pollution. As more data is gathered, AI will be a useful tool for making strides toward achieving a circular economy and reducing plastic waste.
About the Author
You May Also Like