AI-Driven Quality Assurance: Why Everyone Gets It WrongAI-Driven Quality Assurance: Why Everyone Gets It Wrong
AI in quality assurance isn’t a magic solution, but we’ve learned how to leverage generative AI to enhance productivity and improve efficiency, despite some challenges.
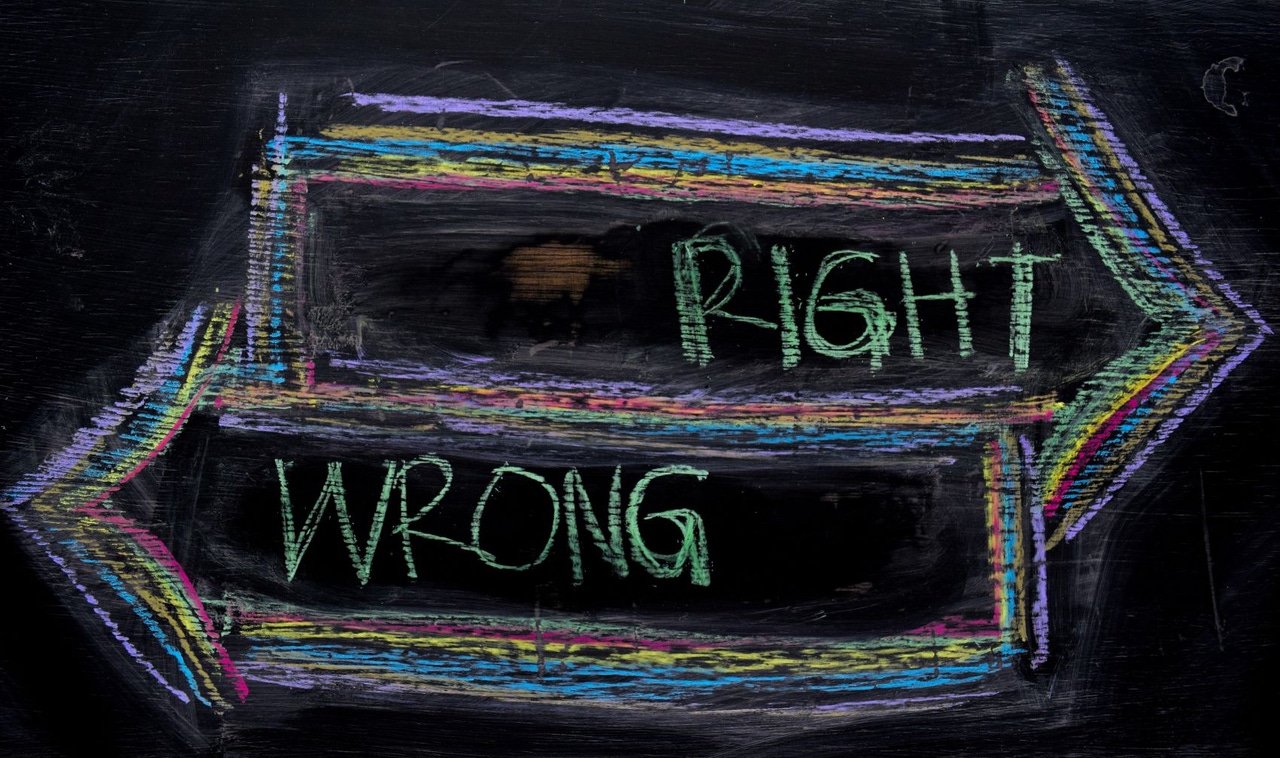
Artificial intelligence is already a big deal, but not everyone is using it effectively. Many clients ask us how we’ve integrated AI into our QA process, but creating a real, usable approach wasn’t as easy as it seemed. Today, I want to share how we approached AI in quality assurance and the lessons we learned along the way.
The AI Hype and Reality
Two years ago, ChatGPT exploded onto the scene. People rushed to learn about generative AI, large language models and machine learning. Initially, the focus was on AI replacing jobs, but over time, these discussions faded, leaving behind a flood of AI-powered products claiming breakthroughs across every industry.
For software development, the main questions were:
How can AI benefit our daily processes?
Will AI replace QA engineers?
What new opportunities can AI bring?
Starting the AI Investigation
At our company, we received an inquiry from sales asking about AI tools we were using. Our response? Well, we were using ChatGPT and GitHub Copilot in some cases, but nothing specifically for QA. So, we set out to explore how AI could genuinely enhance our QA practices.
What we found was that AI could increase productivity, save time, and provide additional quality gates, if implemented correctly. We were eager to explore these benefits.
Categorizing the AI Tools
Over the next few months, we analyzed numerous AI tools, categorizing them into three main groups:
Existing tools with AI features: Many products had added AI features just to ride the hype wave. While some were good, the AI was often just a marketing gimmick, providing basic functionality like test data generation or spell-checking.
AI-based products from scratch: These products aimed to be more intelligent but were often rough around the edges. Their user interfaces were lacking, and many ideas didn't work as expected. However, we saw potential for the future.
False advertising: These were products promising flawless bug-free applications, usually requiring credit card information upfront. We quickly ignored these as obvious scams.
What We Learned
Despite our thorough search, we didn’t find any AI tools ready for large-scale commercial use in QA. Some tools had promising features, like auto-generating tests or recommending test plans, but they were either incomplete or posed security risks by requiring excessive access to source code.
Yet, we identified realistic uses of AI. By focusing on general-use AI models like ChatGPT and GitHub Copilot, we realized that while QA-specific tools weren’t quite there yet, we could still leverage AI in our process. To make the most of it, we surveyed our 400 QA engineers about their use of AI in their daily work.
About half were already using AI, primarily for:
Assisting with test automation
Generating test data
Proofreading documents
Automating routine tasks
Developing a New Approach
We then created an in-house course on generative AI tailored for QA engineers. This empowered them to use AI for tasks like test case generation, documentation, and automating repetitive tasks. As engineers learned, they discovered even more ways to optimize workflows with AI.
How profitable is it? Our measurements showed that AI reduced the time spent on test case generation and documentation by 20%. For coding engineers, AI-enabled them to generate multiple test frameworks in a fraction of the time it would’ve taken manually, speeding up the process. Tasks that used to take weeks could now be done in a day.
The Downsides
Despite its benefits, AI isn’t perfect. It isn’t smart enough to replace jobs, especially for junior engineers. AI may generate test cases, but it often overlooks important checks, or it suggests irrelevant ones. It requires constant oversight and fact-checking.
Why Many Companies Get It Wrong
The biggest mistake companies make is jumping into AI without understanding its limitations. Many fall for the hype and end up using AI tools that don’t work well, only to face frustration. The truth is that AI is a valuable assistive tool, but it needs to be used thoughtfully and alongside human oversight.
Key takeaways from our journey with AI in QA:
AI is not a magic bullet. It provides incremental improvements but won’t radically transform your processes overnight.
Implementing AI takes effort. It needs to be tailored to your needs, and blindly following trends won’t get you far.
AI can assist, but it can’t replace human oversight. It’s ineffective for junior engineers who may not be able to discern when AI is wrong.
Dedicated AI testing tools still need improvement. The market isn’t yet ready for specialized AI tools in QA that offer real value.
AI is exciting and transforming many industries, but in QA, it remains an assistive tool rather than a game-changer. We at NIX are embracing it, but we're not throwing out the rulebook just yet.
About the Author
You May Also Like