AI, Machine Learning Power TransformationAI, Machine Learning Power Transformation
AI and machine learning are transforming how companies work with big data. Here's how one organization is implementing the technology.
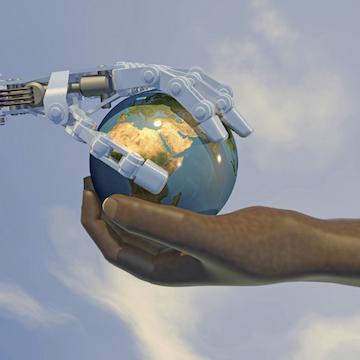
As big data continues to grow, extracting value from it calls for new tools. Increasingly, businesses that rely on data to drive decisions are applying AI to surface actionable insight quickly and accurately.
Finding innovative solutions to the problems raised in data analytics, particularly with respect to adapting machine learning to credit scores, is what they've been working on for the past six years at Experian's DataLabs. The EVP and Global Head of the labs, Eric Haller, spoke to All Analytic about the new direction for predictive modeling.
There's a difference between how modeling was done in the past and the possibilities of current approaches. In the past, Haller says, "it was dominated by logistic regression scoring models." This starts by taking in "a lot of data" that is then distilled "down to characteristics or attributes" that form the basis for the models built.
In contrast, the present approach adapts machine learning, which opens up the possibility of different techniques. Machine learning falls into two types: supervised and unsupervised. While the former is somewhat closer to the older models in that you know the outcomes, the latter entails now knowing outcomes to model against.
For unsupervised machine learning, you "just have a lot of data." You don't go in with the topic defined but go about discovering it through the data and come up with clusters. Now "you see better results, better performance with approaches that are "free of those linear constraints" of the past.
Haller explains that machine learning can help a bank analyze credit card data "to see how its tens of millions of customers are using the card" to direct crossmarketing strategies. For example, you can pull out data clusters that identify which "people may be responsive to Amazon reward cards because that how they behave."
The data can draw on the card usage and find "which merchants or retails locations you'd be most interested in going to." Then the when the card is scan, it can prompt an offer based on the user's patterns. Because of the ability for AI "to fine-tune interests" on this level, Haller believes it works to the advantage of both the customers and the businesses that serve them.
AI's ability to dig through data in this way makes it possible to formulate "much more personal" offers for customers. As a result, marketing is much more specific to the individual than it used to be.
Marketing based on data was usually limited to "where you live." Now that information is "combined with other demographic factors" to arrive at "geo-demographic scores" that can identify differences among neighbors, "and marketing changed because of that," Haller says.
AI's ability to zero in on individual patterns of behavior can also be helpful in flagging fraud. It can be applied to "detecting subtle anomalies that may signal identify fraud" or attempts to compromise an account.
These alerts are more discerning than the type of fraud detection most credit card companies have used. For example, those, would likely freeze a card if it is used abroad. However, thanks to deep learning, that wouldn't happen to a New Yorker on a trip to London.
That's because based on "AI leveraged with deep learning networks that we've established," Haller says. "We know it's very normal for someone from Manhattan to take a trip to London," even if that's not the case for someone "from Brooklyn."
That's the advantage of "deeper, more precise" analytics that "gives us more insight into your true behavior." It's like the difference between using a "surgically precise tool" and the "hammer and nail" of 40 years ago, observes Haller.
The work of the Experian DataLab is to make those tools even more precise, Haller says. We're "continually looking for how to help consumers and small business in coming up with novel ways of leveraging AI and new data sets emerging in the market."
About the Author
You May Also Like