AI, Machine Learning Rising In The EnterpriseAI, Machine Learning Rising In The Enterprise
AI and machine learning are graduating from science fiction to reality. It's estimated that about half of large enterprises are currently experimenting with AI projects. Several vendors, including Facebook, Google, IBM, and Microsoft, have donated machine learning development projects to open source.
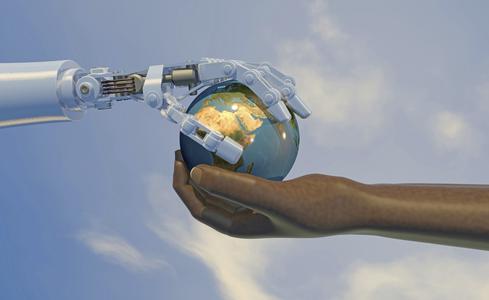
Apple, Microsoft, IBM: 7 Big Analytics Buys You Need to Know
Apple, Microsoft, IBM: 7 Big Analytics Buys You Need to Know (Click image for larger view and slideshow.)
Elon Musk invested millions in an effort to make sure that artificial intelligence is used for good instead of evil, but for much of the general public AI still seems like science fiction -- something far out in the distant future. However, if you talk to people who work closely with this kind of technology, which has been called deep neural networks, deep learning, smart machines, or machine intelligence, you'll find out that it has advanced significantly in the past few years, and even bigger progress is coming very soon.
There are several signposts that indicate this progress, including big enterprises running their own experiments with AI systems, as well as a sudden wave of tech giants taking certain technologies open source.
"The vast preponderance [of projects in enterprises] is still experimentation," Gartner Fellow and vice president Tom Austin told information in an interview. He estimates that about half of large enterprises are experimenting with "smart computing" projects. Organizations are examining problems and deciding whether AI can be applied to the solutions. These efforts are still in the early stages.
Figure 1:
(Image: pixone/iStockphoto)
For instance, maybe an organization would transcribe call center agents' interactions with customers and then compare those transcripts to the agent-generated reports of those interactions to identify differences.
Austin didn't discuss how organizations would use that kind of information, but it's not difficult to see how it could be applied to rate the performance of call center agents and enhance training programs for them, a process that would subsequently improve the call center experience for customers.
"There are many large organizations who are actively looking at that kind of opportunity," Austin said. Lacking the in-house skills, he said, these organizations are going outside their own firms and turning to expertise in academia at AI and machine learning startups and at organizations such as Kaggle. "Enterprises are seeing [this access to talent] as a supply chain problem."
[Intel has assembled an open source big data platform for analytics. Read Intel's TAP Big Data Platform Gains Healthcare, Cloud Partners.]
The arrival of smart machines or AI in the enterprise is not the only signpost showing the progress of these efforts. Another big indication is that several tech industry giants are contributing their machine learning development efforts to open source projects.
Tech Companies Donate Machine Learning to Open Source
Last fall, IBM announced that its machine learning engine SystemML for Apache Spark won acceptance into the Apache Incubator program.
Google released its TensorFlow machine learning system to open source, offering it as a standalone library and associated tools, tutorials, and examples under the Apache 2.0 license.
Microsoft announced the release of its Distributed Machine Learning Toolkit to the developer community, available on GitHub.
Intel made its open source-built Trusted Analytics Platform, more commonly known as TAP, available last summer, and showcased users of the system at the Strata + Hadoop event in New York last fall.
Facebook AI Research (FAIR), which had already released to open source its deep-learning modules for the open source development environment Torch in Jan. 2015, last month announced another move. This time Facebook said it would release its server hardware design that's been optimized for machine learning to open source. Facebook has submitted the GPU-based system design materials to the Open Compute Project.
The company said that the system is designed for greater energy and heat efficiency, as well as ease of maintenance. Digital tech giants such as Facebook, Google, and Amazon that have large data center operations have long designed their own hardware rather than use the designs from others, such as HP Enterprise and Dell.
So, why the big wave of open source releases for machine learning-related development by these big companies?
Technology vendors are looking to spread their developments to a wider audience through these releases. That's according to Gartner Research Director Alexander Linden, who wrote about these developments in a blog post recently.
Gartner's Austin also pointed out that these companies are not giving away the most recent versions of their software. But even if they are releasing the older versions of it, they are spreading the influence and creating momentum. Consider Elon Musk's release all of Tesla's patents to all automakers in an effort to drive the advancement of electric vehicle technology, Austin said.
These releases to open source have been centered on machine learning. Machine learning and AI are two different things.
Machine Learning Does Not Equal AI
Experts say that the definition of AI has evolved over the years since it was introduced. It used to refer to efforts to make machines emulate the way the human brain works, and that is still a field of research today. Yet even today we are not even close to emulating the brains of less sophisticated organisms.
So, today AI means something different.
Next Page: Evolving AI and Machine Learning
What Is AI?
"The definition of AI is very broad, and what goes into AI varies a lot," Ram Sriharsha, senior architect and machine learning expert at big data Hadoop distribution company Hortonworks, told information in an interview. "Machine learning is a subset of AI. AI is being able to communicate, being able to plan and reason and take actions. It's also being able to learn, and that's where machine learning comes in."
AI has more broadly come to refer to computer systems that implement and encompass multiple building blocks depending upon the goal of the system. Those building blocks can be made up of machine learning projects, natural language processing, search, and other technologies.
Michael Schmidt, CEO at Nutonian, a company that bills itself as "the Robotic Data Scientist," concurs with those definitions.
"Machine learning is a small part of AI," he said. "We actually call it machine intelligence. We don't call it AI because that is such a broad term."
Nutonian applies this machine intelligence to its consulting clients' problems, often with material science. For instance, an aluminum mining and production company needed to understand the factors causing grain-sized material produced in mass quantities to lose physical strength.
The material, when used in auto or aircraft manufacturing, would break down in a year. Forensically determining the cause of the failure and how to fix it was a task that humans could perform. But it would take them a long time.
"Not one single person understands the whole process on their own," Schmidt said. "But they do a great job of collecting the data." Yet the problem was too big for humans to solve in a time-effective manner.
Big tech vendors' moves to place some of this machine learning development into open source will spur development even more and will accelerate adoption.
Open source machine learning startup H2O.ai's CEO and cofounder SriSatish Ambati told information in an interview that the goal of open source in this instance is to get machine learning tools to a wider audience.
"We want them to feel that they can take advantage of the data they are capturing and not miss a beat," he said. "The ultimate vision is for prediction to become as powerful as search. Companies will no longer just search for what has happened, but will turn to predicting what's going to happen."
Open source also enables companies to leverage the power of the community.
"What open source is doing for them is simply to recruit the same kind of talent that a software company is able to recruit," he said. That's the pivot that will change all businesses, including the ones that are H2O.ai's clients, such as Capital One and Progressive Insurance. These companies leverage machine learning for the applications and services they offer to their customers.
"How do I build a software maker culture within a bigger business?" Ambati said. Trailblazing organizations across non-technology vertical industries are turning to apps to deliver their services and information -- information built with AI -- to customers. To do that effectively they must nurture a development culture and development talent within the organization. "That's the bigger transformation we are seeing."
About the Author
You May Also Like