AI's Hand in Developing Promising New DrugsAI's Hand in Developing Promising New Drugs
Artificial intelligence is being used to identify drug targets and design new, more effective drugs. Can AI revolutionize how we treat disease?
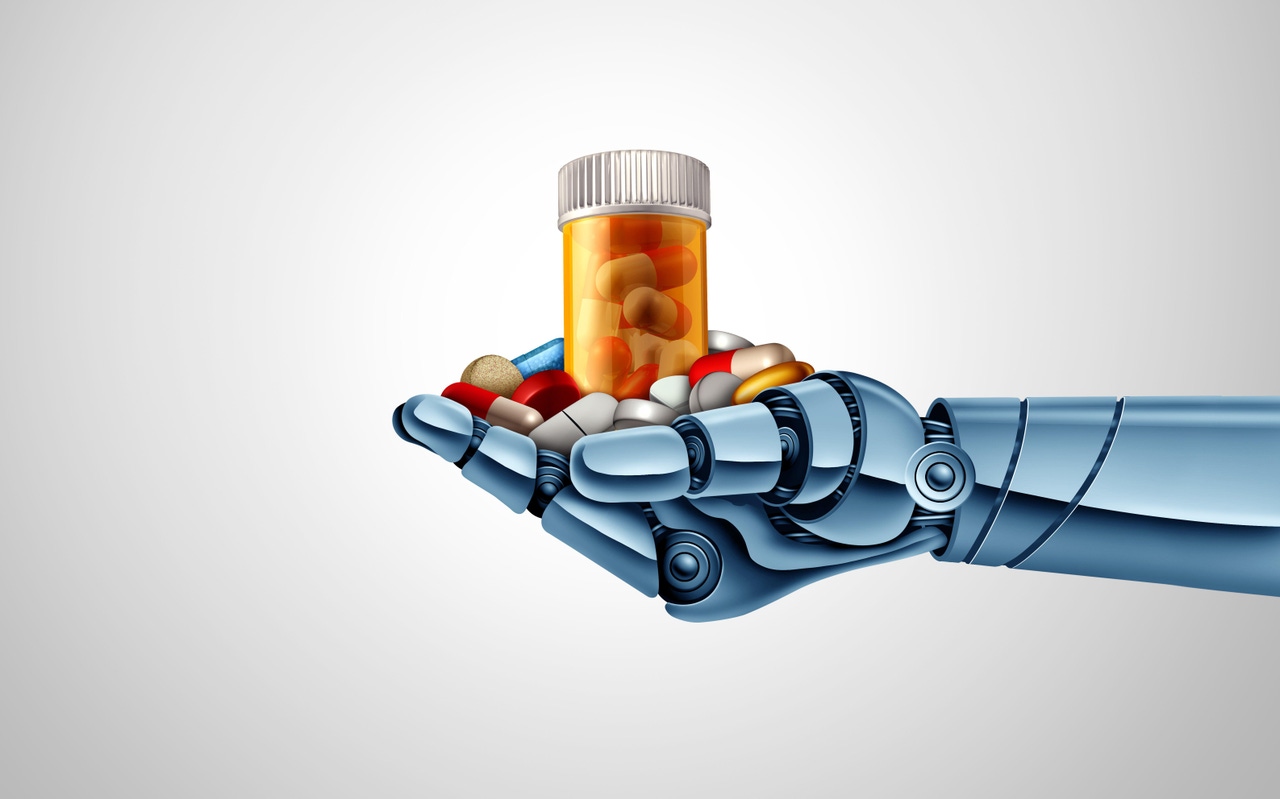
Creating new drugs to treat the panoply of diseases afflicting humanity is an expensive, lengthy and complex process. Fortunes are spent each year attempting to treat our most pernicious and intractable afflictions, from cancer to infections to genetic disorders. There are more than 25,000 diseases affecting humans alone.
Just landing on a promising compound takes years of research and testing it takes years more. It may be decades before a promising treatment reaches patients. A survey of some 21,143 compounds tested found that only around 14% were eventually approved for use between 2000–15.
During the discovery stage, a target for the drug must first be identified -- a DNA or RNA molecule, a protein receptor, or an enzyme. Potential compounds that might attach to it and positively affect the course of disease must be narrowed down, balancing efficacy and potential side effects.
Then, the compound must enter the preclinical stage, in which it is tested on animals to see how it affects the disease in that species and is metabolized by the body. These two stages alone take around six years. The clinical trial stage may take an additional decade or more, depending on the technology used. A huge proportion of the costs are incurred during clinical trials.
It costs an estimated $2.5 billion to develop a drug that can actually be used to treat patients.
Computer-aided drug design (CADD) has been around since the 1990s, but the use of AI has only accelerated in the past decade or so. Now, AI programs are leveraging reams of existing research to identify new targets and compounds that may attach to them.
At lightning speed, these programs are homing in on new avenues for treatment, sometimes using compounds that have never before been deployed. And they are simultaneously identifying potential side effects and suggesting refinements of the manufacturing and regulatory processes. As encouraging as the early results are, these drugs must make their way through clinical trials and obtain regulatory approval.
Here, information investigates the dynamic AI drug development landscape, with insights from Richard Bonneau, vice president of machine learning at biotechnology company Genentech, and Alex Zhavoronkov, founder and CEO of AI drug discovery company Insilico Medicine.
Improving Drug Development Using AI
One study found that as of this year, the top 20 pharmaceutical companies are now using AI to develop new drugs, often in collaboration with dedicated biotechnology partners. Dozens have already advanced to clinical trials. According to a 2021 analysis, 158 candidates were in the discovery phase, suggesting many more could enter the trial phase shortly.
Early estimates suggest that cost savings of nearly 50% may be possible using these technologies. AI generated drugs may represent a $50 billion market in the coming decade according to Morgan Stanley. It might eventually result in annual revenues of up to $110 billion ,according to McKinsey.
Small molecules -- so-named because of their low molecular weight -- make up a large fraction of the drugs currently being tested. These drugs make up most of the current pharmaceutical treatments due to their ease of absorption. They are commonly formulated for oral consumption and are easily absorbed through cell membranes.
Roughly 50% of current trials are focused on cancer treatments. Smaller numbers of vaccines and antibodies are also being developed.
Google’s Deepmind project announced in 2020 that it had been able to predict the formation of proteins using its AlphaFold AI model -- a crucial development given the integral role that proteins play in the body. The program built on early successes in beating the game Go.
While previously the determination of the sequences leading to the folding of proteins into different shapes had been costly and time-consuming, the program accurately calculated how proteins would form and bind to other molecules, opening the door to the development of a wide variety of new treatments.
Prior to the development of this technology, which other companies have employed as well, the number of protein structures that could be accurately predicted numbered in the hundreds of thousands. Now, these programs have done the same for hundreds of millions of proteins.
Promise has been shown in individualized medicine -- analysis of ‘omics,’ such as genomics, proteomics and transcriptomics to allow more finely targeted drugs. In one application, Genentech is testing cancer vaccines tailored to individual patients -- using antigens that help the body recognize and destroy particular types of cancer cells.
These programs utilize a range of techniques. The creation of knowledge graphs drawn from the existing literature can identify patterns in previous findings. Analysis of clinical data, including images and tissue samples, can elucidate the progression of various pathologies and how treatments affect them over time.
Zhavoronkov explains that Insilico’s P3GPT, a large language model (LLM) engine, can identify promising avenues of research.
“Although P3GPT is not conversational, you can communicate your research question to it with a structured prompt,” he says. “You can ask it: What genes are overexpressed in human aging? And then, follow up with another question: What small molecules can reverse this expression signature? P3GPT is flexible enough to support this workflow in different species and tissues. In a similar fashion you could get compounds targeting a particular disease or pool together its responses for prompts featuring different tissues to get a compound with a systemic effect.”
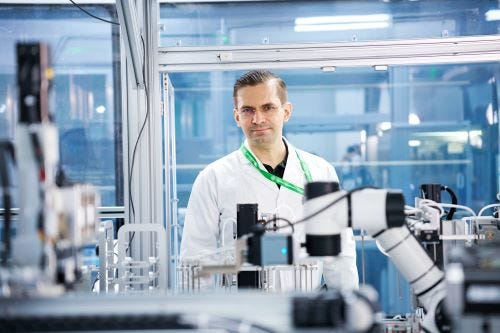
Alex Zhavoronkov, Insilico
As the predictions created by AI and machine learning programs are tested in the real world, the results of these experiments are then fed back into the models to improve future performance. Genentech refers to this as a ‘lab in a loop.’ AI programs can filter out less promising drug candidates and refine the most promising ones before they are compounded and tested.
The experiments are conducted in silico -- in a virtual environment. The term builds upon those used for traditional forms of experimentation. In vitro experiments are conducted outside of the body, in petri dishes and other set ups and in vivo experiments are conducted on living subjects.
Even in traditional drug development programs, AI may be useful in managing the project, synthesizing internal data, and applying it to the experiments. In many cases, these applications do not directly affect the development of the drug itself but may apply to the creation of software utilized in the process or the extraction of knowledge from previous projects.
Looking at Disease Using AI
In designing new drugs, one of the most useful places to start is their targets -- the areas of cells that they will affect, thus stopping pathogens, limiting the spread of cancer, or reducing pain. By analyzing these target areas, it then becomes easier to locate or design molecules that will attach there. Traditional drug design has been a process of trying to jam roughly compatible puzzle pieces together and seeing what clicks. By more clearly identifying the first puzzle piece -- the target; the second, the drug -- can then be more efficiently refined or designed.
Analysis of target sites from millions of papers and reams of clinical data can reduce the time it takes to zero in on compounds that will affect their function. Sometimes, these targets are entirely new. Poring over countless microscopic images and laboratory findings may help to identify trends in the expression of proteins that manifest in diseases that have otherwise been missed.
“A lot of it is just organizing the information -- making sure you can access the library of everything that came before,” Bonneau says. “You can then take it a step further and try to infer what the pathways are and incorporate genomic information. At Genentech, we are doing quite a bit to integrate the sum total of knowledge encapsulated in the literature.”
In some cases, existing drugs have been matched to new or existing targets, creating further efficiencies. If the drug is already in use, it can skip phase I trials and move directly to phase II. Matching existing drugs to these targets can save tens of millions of dollars in development costs. Further, analysis of interaction with undesirable targets may allow for existing drugs to be modified, reducing their side effects. So-called ‘me too’ drugs are essentially altered forms of existing drugs, in which minor portions of the molecules are tweaked.
“Once you have targets, you have to very quickly come up with tool molecules to verify that your hypothesis is sound,” Bonneau says. “You need an early version of the drug fast. And that's all about molecular design.”
Refinement and Identification of New Compounds
Programs such as AlphaFold and its competitors have made great strides in developing new proteins by predicting their structure using their amino acid sequences. These sequences determine the protein’s eventual final shape -- an organic form of origami. These complex shapes determine how the protein will interact with other molecules and how it will function in the treatment of disease.
By reverse engineering proteins and determining how similar shapes aligned with similar arrays of amino acids -- based on decades of examination of these structures in the lab -- these programs have been able to discern patterns in protein formation. This predictive ability now allows scientists to alter existing proteins and create new ones -- antibodies for example -- to treat disease.
These same principles can be applied to other compounds, such as small molecules. Discovery of small molecules was estimated to increase 40% each year in 2022.
AI can also assess how the body metabolizes drugs. Some drugs, for example, are highly effective but are quickly destroyed by other processes such as digestion. Adjusting their structure can mean they survive longer and have a greater impact on targeted diseases. AI programs can also be used to predict how toxic a particular treatment may be and thus lead to adjustments that reduce undesirable side effects.
“As you move that molecule from tool compound to drug to medicine, then you have to start layering in safety clearance and the pharmacokinetics and pharmacodynamics. Where does the drug go? How long does it stay?” Bonneau notes.
More radical and even novel structures can be designed as well. There are some 1060 chemicals, only a small fraction of which have been deployed in drug development. Mining the vast library of known chemicals may help to identify treatments unlikely to have been imagined by human researchers.
“There are cases where we're deriving and optimizing something. And there are other cases where we're fishing for a wholly new thing. When you're fishing, you almost always get new fish. When you're developing things, you might make a very small change. It could be mind-blowingly useful,” Bonneau says.
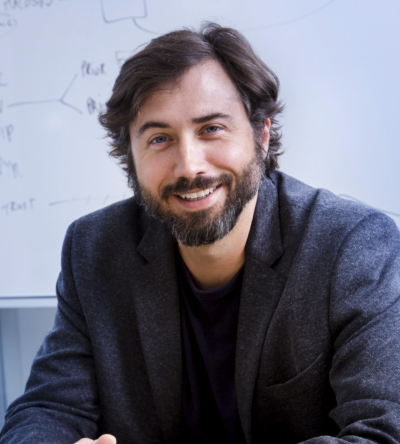
Richard Bonneau, Genentech
Depending on the parameters defined for the drug, AI programs can then rank them according to their properties, quickly organizing the positives and negatives of each so that the most useful -- and ultimately marketable -- candidates can be tested in the lab first.
AI programs can also be used to adjust other aspects of a compound -- such as its solubility or stability, leading to better absorption by the body and longer shelf life. The manufacturing process can be tested and refined as well. Alternate means of synthesizing these complicated compounds may be suggested, ultimately leading to reduced costs. And physical processes such as the speed of the blades used to blend powdered drugs and the means of coating them for consumption can be optimized.
“Our target discovery philosophy is to discover if there is an optimal balance between commercial tractability, novelty and confidence. Then we identify the best possible molecule out of generative AI that will likely make a great drug -- something that satisfies all the rules of classical medicinal chemistry but at the same time exceeds human intelligence and basic computational tools,” Zhavoronkov claims.
“At the very beginning, when we are deciding which target molecule to go after, we make a plan and a forecast of the probability of Phase II to Phase III transition. Our goal is to ensure that we pass efficacy testing in Phase II human clinical trials. At the very early discovery stage we already have incorporated that information into the decision-making process,” he adds.
First AI Drugs Moving to Trials
As the drugs themselves progress toward and through the trial stage, the information gathered along the way can be organized by AI as well, identifying patterns that humans might not notice and potentially reducing redundancies and procedures that may lead to dead ends. This frees researchers from laborious analysis and gives them time to engage in real-world lab work that can then itself be fed back into the models.
“If we can build bigger ensembles of molecules then we can be harder on the things that are in that ensemble, and select and then ultimately optimize even better molecules,” Bonneau says of this feedback process.
AI may also lead to the selection of the most appropriate trial subjects, so patients are not subjected to useless treatments and the drugs themselves can be tested on those they may actually help. More narrowly selected groups of subjects can help to address needs that would be overlooked in broader, more general trials.
Early trials are already progressing. The first AI-generated drug, a cancer treatment designed by Exscientia, entered trials in 2020. While the company discontinued them in 2023, citing ‘strategic’ reasons, a suite of other AI-generated treatments are currently being tested.
Insilico began trials of a drug used to treat idiopathic pulmonary fibrosis, a lung disease, that was based on an AI-identified target in 2022 -- only two and a half years after it was identified. The compound received orphan drug designation, which provides additional incentives for the development of drugs that treat rare diseases, in 2023 and entered phase II trials that same year. Phase IIa trials were initiated in June 2024.
Nearly 70 molecules identified by AI programs are now in clinical trials, with 21 having advanced to phase II trials at the end of 2023 according to one analysis. That indicates a success rate of some 90%, an improvement over the 40% success rate for standard trials. Insilico was the first to bring an AI generated drug to phase II in July 2023.
However, these impressive gains leveled off in phase II trials, with only four out of ten being successful. The study notes, however, that some of the trials were simply discontinued due to shifting business priorities.
New drugs are subjected to rigorous and complex regulatory processes. AI programs can help identify potential sticking points before the onslaught of Health Authority Queries (HAQs) that interrogate the safety and usefulness of the drugs, so that answers can be prepared beforehand.
Hurdles to Implementation of AI drugs
While AI drug development has shown remarkable promise, substantial obstacles remain in place before it becomes a truly viable means of creating new and useful compounds. Potential drugs still need to clear trials and pass regulatory muster. The Food and Drug Administration (FDA) claims that it is looking at developing a more “agile regulatory ecosystem that can facilitate innovation while safeguarding public health.”
Still, a number of additional issues remain. AI programs may “hallucinate,” coming to conclusions that are not accurate or are actually dangerous. In the realm of drug development, this may result in the suggestion of drug compounds that are not actually feasible or that may result in unpredictable negative effects. And some AI programs may not yet be sophisticated enough to manage the volumes of data needed to suggest compounds that will actually be useful.
Conversely, in some situations, there may not be enough existing data to usefully train the models. If models are developed using inadequate data, they are likely to return inadequate results. Synthetic data can make up some of the shortfall, but it may have limited utility in developing drugs that can actually be implemented.
While a range of purpose-driven AI drug companies have emerged, allowing for the development of targeted protocols, AI is also being implemented by existing drug companies. Striking a balance between long-standing pathways and novel, AI-driven ones can create significant disruption. Rolling out AI implementation in a constrained manner can allow companies to test and balance its benefits and potentially chaotic impacts.
Some of these issues will ultimately be addressed as the molecules are produced in the real world and tested. The results of these tests can then be included in the AI models that produced them in the first place. When errors are identified, they can be factored into future models, which will -- at least hypothetically -- then avoid the same mistakes.
Concerns have also been raised that -- like other AI innovation -- these programs may replace human workers. Advocates counter that there is still a substantial role to be played by human researchers in guiding them and in implementing their results in the lab. Indeed, it seems human researchers will remain essential in testing AI compounds and feeding their results back into the machine intelligence from which they originated -- and teaching it to suggest even more useful ones in the future.
About the Author
You May Also Like