Amazon's HR Gaffe Demonstrates Important PointsAmazon's HR Gaffe Demonstrates Important Points
Amazon recently proved it isn't infallible when it shut down a human resources system that was systematically biased against women. However, there's more to the story that today's enterprise leaders should know.
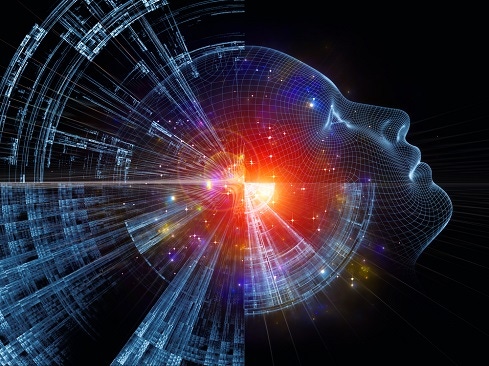
When people talk about machine learning masters, Amazon is always top-of-mind. For more than two decades, the company's recommendation capabilities have been coveted by others hoping to imitate it. However, even Amazon hasn't mastered machine learning completely, as evidenced by a biased HR system it shut down. What may be surprising to some is the reality of the underlying situation, which is that biased data isn't just a technical problem, it's a business problem.
Specifically, Reuters and others recently reported that since 2014 Amazon had been using a recruiting engine that was systematically biased against women seeking technical positions. It doesn't necessarily follow that Amazon is biased against tech-savvy women, but the situation does seem to indicate that the historical data used to train the system included more males than females.
Historically, more men have held technical positions than women, generally speaking, not just at Amazon. At the present time, the world is comprised of about half men and half women, with one sex more predominant in some cultures than others. However, women hold 26% of "professional computing occupations". If the dataset represents that three out of four workers in a technical position are men, then it follows an AI trained on the data would reflect the underlying data.
Amazon is now faced with a public relations fiasco even though it abandoned the system. According to a spokesperson, it "was never used by Amazon recruiters to evaluate candidates." It was used in a trial phase, never independently and never rolled out to a larger group. The project was abandoned a couple years ago for many reasons, including that it never returned strong candidates for a role. Interestingly, the company claims that bias wasn't the issue.
If bias isn't the issue, then what is?
There's no doubt that the outcome of Amazon's HR system was biased. Biased data produces biased outcomes. However, there is another important issue not identified by Amazon or other some media, which is data quality.
For years, organizations have been hearing about the need for good-quality data. For one thing, good-quality data is more reliable than bad-quality data. Just about every business wants to use analytics to make better business decisions, but not everyone is thinking about the quality of the data that is being relied upon to make such decisions. Data is also used to train AI systems, so the quality of that data should be top-of-mind. Sadly, in an HR context, bad data is the norm.
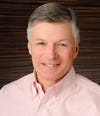
Kevin Parker
"If they'd asked us, I would have said starting with resumes is a bad idea," said Kevin Parker, CEO of hiring intelligence company HireVue. "It will never work, particularly when you're looking at resumes for training data. "
As if the poor quality of resume data wasn't enough to derail Amazon's project, add job descriptions. Job descriptions are often poorly written, so the likely result is a system that attempts to match attributes from one pool of poor quality data with another pool of poor-quality data.
Bias is a huge issue, regardless
Humans tend to be naturally biased creatures. Since humans have created and are still behind the creation of data, it only stands to reason that their biases will be reflected in the data. While there are ways of correcting for bias, it isn't as simple as pressing a button. One must be able to identify the bias in the first place and should also understand the context of that bias.
"We think of resumes as a representation of the person, but let's go to the person and get to the root of what we're trying to do, and try to figure out if the person is a great match for this particular job. Are they empathetic? Are they great problem solvers? Are they great analytical thinkers? All of the things that define success in a job or role," said HireVue's Parker.
HireVue is building its own AI models that are correlated to performance in customer organizations.
"[The models are] validated. We do a lot of work to eliminate bias in the training data and we can prove it arithmetically," said Parker. "The underlying flaw is don't start with resumes because it won't end well."
HireVue looks at the data collected during the course of a 20 to 30-minute video interview. During that time, it's able to collect tens of thousands of data points. Its system is purportedly capable of showing an arithmetic before and after, so if all successful people in a particular role are middle-aged white men but the same level of success is desired from a more diverse workforce, then what are the underlying competencies and work-related skills is the company seeking?
"By understanding the attributes of the best, middle and poor performers in an organization, an AI model can be built [that looks] for those attributes in a video interview so you can know almost in real-time if a candidate is a good candidate or not and respond to each in a different way," said Parker.
Recruitment software and marketplace ScoutExchange analyzes the track record of individual recruiters to identify the types of biases they've exhibited over time, such as whether they hired more men than women or whether they tend to prefer candidates from certain colleges or universities over others.
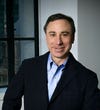
Ken Lazarus
"There's bias in all data and you need a strategy to deal with it or you're going to end up results you don't like and you won't use [the system]," said Ken Lazarus, CEO of ScoutExchange. "The people at Amazon are pretty smart and pretty good at machine learning and recommendations, but it points out the real difficulty of trying to match humans without any track record. We look at a recruiter's track record so we can remove bias. Everyone needs a strategy to do that or you're not going to get anywhere. "
The three things to take away from Amazon's situation are these:
Despite all the hype about machine learning, it isn't perfect. Even Amazon doesn't get everything right all the time. No organization or individual does.
Bias isn't the sole domain of statisticians and data scientists. Business and IT leaders need to be concerned about it because bias can have very real business impacts as Amazon's gaffe demonstrates.
Data quality matters. Data quality is not considered as hot a topic as AI, but the two go hand-in-hand. Data is AI brain food.
[For more about data bias in AI, check out these articles.]
10 Ways AI Will Alter the Future of Work
Six Steps for Businesses to Earn AI Trust
AI Is a Powerful Ally in Public Safety - Responsible Use Is Paramount The Coming Wave of Regulation over Facial Recognition
About the Author
You May Also Like