Assess and Realize Value from Generative AI Enterprise InvestmentsAssess and Realize Value from Generative AI Enterprise Investments
IT leaders must identify and prioritize high-value generative AI use cases to ensure positive return on investments.
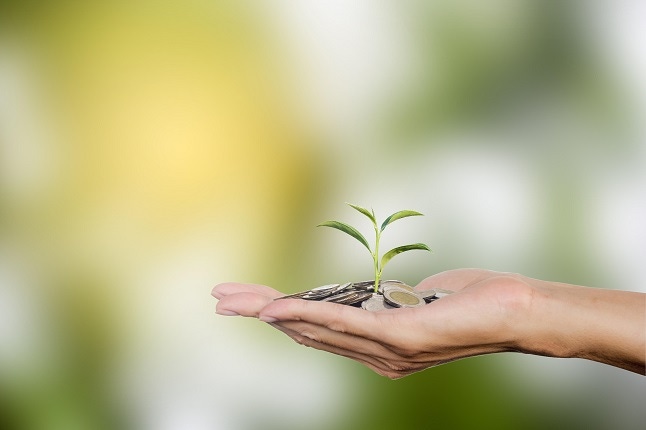
Business and technology leaders are in a race to get started with generative AI in the hopes that they will benefit from potentially unprecedented productivity improvements and business transformation opportunities.
Early adopters of generative AI chatbots, coding assistants, and similar tools are reporting significant business impact across a variety of processes. Most early studies of generative AI value realization are reporting on easily quantifiable benefits with clear cost and revenue alignment, such as improved task automation and work quality. However, generative AI value can extend to improvements in user experience, service levels, employee engagement, customer engagement, and employee training or upskilling. Generative AI also has the potential to completely transform industry dynamics, create new market winners and losers and even create new industries.
However, there are high structural costs to training and running large language models (LLMs). Even when LLMs with billions of parameters are trained, they continue to require massive computing power to run. Current vendor pricing models that pass on the high cost of innovation and developing, training, and running LLMs may result in a negative ROI for many seemingly high value use cases when deployed at scale. This can be true even when pricing is subsidized by vendors, due to the additional necessary investments in cloud and AI infrastructure, data management, new talent and skills, new systems to support changes in work and processes, and risk management.
Gartner predicts that by 2025, growth in 90% of enterprise deployments of generative AI will slow as costs exceed value, resulting in accelerated adoption of smaller custom models. By 2028, more than 50% of enterprises that have built their own large models from scratch will abandon their efforts due to costs, complexity, and technical debt in their deployments.
IT leaders tasked with assessing the value of generative AI investments should follow a framework to identify and prioritize high-value use cases by evaluating competitive impact, business value, urgency, cost, and risk. Use cases can be divided into three key categories, each of which requires a different measurement and implementation approach.
Leverage Quick Wins to Learn, Assess and Prove Value
Quick wins from generative AI productivity assistants have low barriers to adoption but may deliver diminishing competitive differentiation over time. Quick wins are easy to get started, easy to pilot and easy to buy, but are usually task specific. For example, generative AI coding assistants can support productivity gains for developers, while chatbots can help content marketers create copy, product descriptions, press releases and videos.
Benefits for quick win applications should be measured on time savings spent for those specific tasks and across aggregate tasks related to specific processes. For example, if an average software developer spends 50% of their time writing code, and can be 33% to 55% more productive, they can save 1.32 to 2.2 hours per day. Assuming a loaded cost of $120,000 per year per coding resource, that would translate into $79.20 to $132 of value per day or $1,584 to $2,640 per month in savings, for a license cost per user per month of $20 to $55. This value could be used to reduce coding staff or gain higher productivity from existing staff. This could mean that, with existing staff, more resources would be available to work on new projects and innovation versus maintenance or to reduce the backlog and time to deliver projects. Both outcomes from new-found productivity gains would result in realizing higher business value from existing coding resources.
Given their low barriers to adoption and broad democratization, gains from quick wins can rapidly become table stakes. However, how these capabilities are integrated with other business processes can still provide a competitive moat for enterprises. Moreover, there may be incremental costs for data management, risk management and security, training and change management, and content and benefit auditing.
Leverage low-cost pilots to learn, assess and prove value. Determine which roles are realizing productivity gains and measure the associated financial benefit and additional costs required when deploying at scale. Such costs might include investments in new types of data repositories, updating the knowledge architecture or and/or data labeling. Also identify outcomes that cannot easily be quantified in financial terms. Simulate upper and lower bounds for benefit and cost to account for uncertainty.
Invest in Differentiating Use Cases to Improve Specific Processes
Investments in differentiating use cases that leverage generative AI embedded in enterprise, domain and industry applications or custom applications have the potential to improve specific business processes. They can leverage enterprise data in unique ways for differentiation and more defensible competitive advantage than quick wins, but they come with higher and more unpredictable costs and risk at scale.
Differentiating generative AI use cases will require higher investment than quick wins, but the costs can generally be offset by financial benefit resulting from productivity gains and the potential for revenue generation -- assuming effective underlying process redesign, upskilling and risk management. For example, consider use cases such as generative AI assisted customer support, or hyper-personalized sales content creation. The license cost of the requisite applications will typically measure well below the ROI of the resulting productivity gains.
Conduct scenario analysis and planning as part of the proof of concept and pilot for these use cases to assess total costs at scale. Assess potential incremental costs for implementation, data management, running and maintaining the models, risk, security, systems to support new processes, training, and change management. Identify metrics that capture both financial benefits and strategic outcomes, such as better user experience, broader access to capabilities previously requiring higher skills, and employee and customer satisfaction -- and assess their impact to calculate ROI.
Explore Transformative Use Cases for Disruptive Innovation
Unique competitive advantage and industry disruption from new generative AI products, services and business models will require investments in transformative use cases with higher cost, complexity, and risk. For example, an insurance company could fine-tune a foundation model with its own policy documents to incorporate this knowledge into the model and transform its underwriting, risk management and opportunity identification performance. A financial services organization might create a foundation model trained with financial data, which could then be used for products that assist with investment identification, tax, risk, and compliance assessments, among others.
Executives and boards will need to accept higher risk tolerance for industry-transforming and disruptive innovation from new generative AI products and business models. Investment decisions should be based on strategic, competitive, and market-level impact considerations. Prioritize strategic benefits that may be difficult to quantify in financial terms over immediately identifiable task- or process-specific financial benefits.
Create a tiger team, including executive leadership, corporate strategy, and business and technology leaders to identify and assess potentially disruptive generative AI-based products, services, and delivery and business models. Socialize the need for a higher risk tolerance and new investment criteria that prioritize strategic value over task- or process-specific productivity benefits for transformational GenAI opportunities with the board and executive leadership.
By building a portfolio of quick wins, differentiating and transformative use cases, data and analytics leaders can determine the best opportunities for realizing value from generative AI investment. Use an options-based approach that combines initiatives with hard ROI with loss leaders and those delivering transformational benefits and competitive advantages that are difficult to initially quantify directly in financial terms. Monitor and reassess deployment approaches as new market options and processing innovations lower compute costs and as vendor pricing models evolve to drive demand.
Rita Sallam is a Distinguished VP Analyst and Fellow at Gartner, Inc. researching best practices for realizing business value from data, analytics, and AI investments. Rita and other Gartner analysts will provide additional analysis on generative AI and business transformation at Gartner IT Symposium/Xpo, taking place October 16-19, in Orlando, FL.
About the Author
You May Also Like