Augmented Analytics Evolves to Make AI, BI Easier in 2021Augmented Analytics Evolves to Make AI, BI Easier in 2021
Augmented analytics has helped enterprises accelerate BI, analytics, and AI programs. Here's how it will advance for 2021.
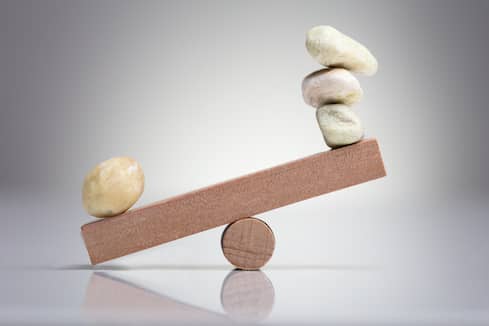
Augmented analytics is entering the mainstream in 2021, which means more enterprise organizations will be able to take advantage of its benefits to accelerate business intelligence, machine learning, and other forms of artificial intelligence in their organizations, whether that means more production projects or faster insights for decision makers.
But just what is augmented analytics? It's not a particular technology or product. But it is the idea of leveraging technologies such as machine learning and analytics to help automate the entire data management pipeline from data preparation to generating insights to assisting with building models and operationalizing them.
That's crucial because data science and machine learning are complex and difficult. That's why just a few years ago so many organizations were struggling to hire "unicorn" data scientists who were experienced in three different areas: statistics, coding, and a specific business domain. It's also why those organizations also struggled to get their initial machine learning pilots in place, and one of the reasons why they have struggled to get those pilots into production.
Read more on augmented analytics, AI, and BI here:
10 Trends Accelerating Edge Computing
Augmented Analytics Drives Next Wave of AI, Machine Learning, BI
How to Create a Successful AI Program
How Analytics Helped Accenture's Pandemic Plans
Augmented analytics or augmented intelligence use machine learning and automation to assist with the hard parts of analytics, machine learning, and other AI.
"Augmented intelligence has emerged as a practice that involves people, process and platform that aims to utilize mostly machine learning mechanisms to do a couple things," said Bradley Shimmin, Omdia chief analyst for AI platforms, analytics, and data management.
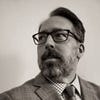
Bradley Shimmin
"One is to augment human decision making and the other is to automate processes that may be too difficult for people to make on their own if they didn't understand the context or the history, or if it's just too complex for any human to deal with."
Augmented analytics really got its start a few years ago when organizations began to use machine learning tools to assist with data prep. But the idea of augmented analytics has expanded since then to incorporate many other parts of the data and analytics pipeline, from preparation to insights.
That's meant that data scientists, data analysts, data engineers, and other data professionals aren't spending so much time on repetitive manual processes.
Shimmin said that the impact of this is not to replace data engineers. Rather it's to democratize data across the enterprise.
"Now I might have a dashboard that is always up to date, and always running," he said. "I can pull it up on my phone on the way to work and it will show me if there are any outliers and even tell me what action I might have to take...What you may have been paying your Cognos developer to do in three weeks, you can now create via drag and drop in a few minutes."
Augmented analytics has advanced this far in part because software development companies have started to include the functionality in their platforms. Some have made acquisitions to add automation and data prep to their platforms, like Data Robot acquiring Paxata and Tableau acquiring Empirical Systems. Microsoft's Power BI, Qlik, and other vendors have also added augmented analytics features to their platforms, too. These additions have opened up the practice to the mainstream enterprises in 2020. That will accelerate enterprise data initiatives in 2021 from business intelligence to AI.
One example of where this is going in business intelligence and data visualization comes from Tableau, which is baking Salesforce's Einstein Discovery into the Tableau platform. Shimmin said when you are in Tableau you can click on an outlier and be able to see all the models. Salesforce, Oracle, and SAP are already building this technology into their platforms, too.
"Having actionable intelligence at the moment of decision making is the goal," Shimmin said.
Another goal is to make consumption of the insights less overwhelming and more tailored to the person who needs it. Gartner VP analyst Rita Sallam said that augmented analytics will help move organizations beyond the dashboard paradigm to a new way of consuming insights -- a data story. These will help users understand just the insight and context that they need at the right moment to make the decision.
"By 2025, data stories will be the most widespread way of consuming analytics," Sallam said. "Seventy-five percent of those stories will be automatically generated using augmented analytics techniques."
{Image 3}
Just as machine learning helped with data preparation, these technologies will move down the line to help users consume the insights, she said.
"AI and machine learning techniques are making their way into analytics and BI platforms and to data science and machine learning platforms to automate insight generation, to automate model identification, feature engineering, and model management," Sallam said.
The idea is to reduce the amount of time that users will need to spend in predefined dashboards with predefined KPIs where they are required to do a lot of the manual analysis and filtering, sorting, and pivoting, according to Sallam. Because augmented analytics will perform these tasks, these systems will become easier to consume for more users across the enterprise.
Another development coming to augmented analytics that will also democratize its use throughout the enterprise will be the addition of natural language. This will allow users to submit queries using natural language voice or text queries to request the information that they need. Software providers are adding these functions into their tools now.
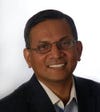
Rao Anand
Anand Rao, artificial intelligence lead at PwC, said that augmented intelligence has really come to the fore over the last 5 years, but we are still in the early days of what it has the potential to do. About 20% of companies have been leading the charge in this area, and they are moving into the realm of MLOps to help manage and automate the model development and operation process, according to Rao.
Augmented analytics is also likely to eventually help organizations get a handle on explainable AI. When artificial intelligence offers an insight or a result, it's important that organizations be able to dive into that and understand why, and also to be able to explain the result for governance or compliance purposes.
About the Author
You May Also Like