Avoid Failure in Self-Service AnalyticsAvoid Failure in Self-Service Analytics
Self-service analytics is becoming a popular option, but there are some steps professionals should use to make sure that they are interpreting results correctly, working well with others, and avoiding mishaps in reporting.
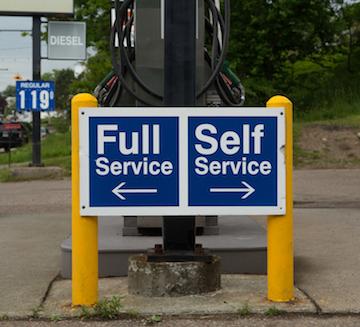
You would think that a CIO or executive responsible for analytics and business intelligence within an enterprise would see self-service tech as a boon to an organization's initiatives. After all, the market is flush with solutions that are providing a variety of analysis and visualization options for the onslaught of data. Instead, CIOs are likely finding their hands full with organizing their teams to better utilize that self-service stack.
Analytics services have been around for a while, but usage has spiked sharply because of the rising demand for data mining, various visualizations, and increased applications of machine learning techniques. Companies are learning that self-service analytics platforms hold potential for empowering employees to transform stored data into meaningful insights.
Self-service analytics include open source platforms such as Neo4j, a graph database, and SAS Virtual Analytics, a data visualization tool. For machine learning algorithms, cloud platforms exist for managing techniques such as decision trees, ensemble machine learning, and other applications. Microsoft, Google, and Amazon are among the large providers, while other players such as H20 and Apache bring unique features.
Despite a variety of tools, initiatives to use self-service analytics can suffer due to an organization's ability to manage skills and governance within teams that have access to the data. Managers within organizations can quickly lose sight of how practitioners view data on a daily basis, even when the data access is centralized. A meaningful vision of implementing self-service analytics involves more than data preparation with new platforms. It involves aligning people and processes to mitigate risks in leveraging skills and in managing data governance.
Understanding employee viewpoints of data is also critical, as machine learning becomes an essential process in organizations. Data hygiene related to machine learning requires more exacting demands for a model than reporting dashboards, because the model learning accuracy is based on the quality of collected data. Thus teams involved with an analysis need to agree with the initial assumptions for addressing empty fields, data outliers, and other assumptions that impact the model.
We can imagine professionals having a relationship with data, models, and metrics in the following list:
Executives -- only care about KPIs -- the bottom-line metrics that reflect business performance
Analysts -- usually analyze metrics related to KPIs
Data scientists -- have assumptions on data and models
External users -- have access to end reports or metrics alongside the analyst.
Each user is a subject matter expert who may view data in different contexts and with different needs. Each is in different departments and possesses different skills, all leading to views of data for different purposes. Thus the insights each expert gains may be useful to that particular expert's needs but they may not link into an overview that is best for the entire enterprise. That perspective handcuffs a team to limited solutions instead of looking at other solutions that can better advance an organization to its objectives.
Establishing coherent professional skills and communication across teams can help an organization avoid this false scope.
The key is to realize where to ask more probing questions. It is possible to scope a project, but is the result of the analysis the right kind of assessment with the data? Will it solve the problems the organization truly cares about?
Expanding the viewpoint beyond that of a subject matter expert will counter narrow perspectives on how data can be modeled. It means being proactive through collaborative tools and through occasional check-up discussions where teams voice their observations and identify potential governance concerns.
One more aspect that teams must recognize is how fragile the process of spotting potential skill and knowledge gaps can be. Depending on model purpose and how a department is aligned, identification of where to improve skills and communication can take some time to wind through the processes in creating a model. Self-service data preparation, for example, can take as long as two months. The tasks usually include gathering requirements, modeling data, creating a report, and arranging it for distribution of reports. So some needed tasks or even implemented improvement may be apparent immediately.
Those tasks are not going away anytime soon, for businesses of any size or scale. Small Business Trends highlighted a Gartner report that forecasted increases in business spend on analytic solutions, which includes self-service analytics. Look around your workplaces or consider your interaction with remote colleagues, and you can certainly see signs that the business community is indeed changing into a self-service world.
In response, executives should view a self-service data environment with an emphasis on managing team needs relative to data preparation and data integration. Doing so allows analytics professionals to remain alert on interpreting results, working with other professionals, and avoiding reporting mishaps. It will also allow CIOs to have their hands free for the next business challenge.
About the Author
You May Also Like