Avoid These Pitfalls to Get Maximum Value from Data ScienceAvoid These Pitfalls to Get Maximum Value from Data Science
Data science techniques are getting better, cheaper, and easier to use. Even small and medium sized organizations can now tap these technologies. But, if you fail to properly introduce, support, and integrate data science capabilities, a lot of money can be wasted.
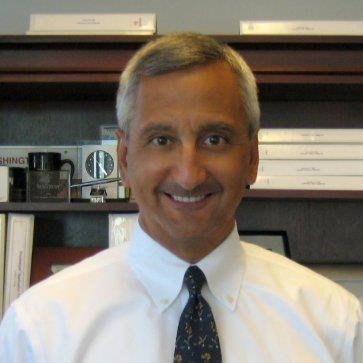
Despite enormous enthusiasm for data science, especially machine learning, many organizations struggle to realize the business value they had hoped for. At the same time, many data scientists feel bogged down with low value work that keeps them from focusing on where they can contribute the most to the business.
How might we increase data scientist productivity, boost data scientist job satisfaction (and retention), and get more out of our investments in data and analytics? I propose that there are four fundamental challenges which must be addressed.
First, many data scientists find themselves encumbered by all the legacy data challenges of the organization. Data may be difficult to extract and hard to integrate, semantics may be inconsistent and data quality questionable, and gaps in the data (historic or otherwise) may be significant. As a result, many data science leaders lament that 80% or more of their capacity is spent finding, assembling, cleansing, and preparing the data for use.
Second, once a valuable insight into the data has been found, or a valuable use of data has been developed and delivered, data science organizations are often burdened with making these solutions production ready, bringing them to speed and to scale, and conforming them to organizational standards. In some cases, it ends up being the data scientists themselves who are responsible for ongoing operation, maintenance, changes and enhancements, and production support.
Third, as data assets are created, expertise in data domains grows, and capabilities are developed, it is often the data science community that becomes the “go to” group for all ad-hoc information requests. These requests may be more akin to traditional business intelligence (i.e. reporting) than data science. You might be surprised how often regulatory requests for information end up distracting data science teams from their modeling and development efforts. Often, these then add to the maintenance and ongoing operational burden.
Fourth, in the early stages of analysis and development of new data science capabilities, it may be unclear how the solution ultimately will be deployed through the organization and integrated into the business process. Poorly thought through deployments of even the most impressive insights will hinder adoption, acceptance, and value realization.
It’s no wonder that organizational leaders are often unsatisfied with the bang for their data science buck. It's also no surprise when data scientists themselves are unsatisfied with the proportion of their time spent doing true data science.
The answer lies, as is often the case, in transforming the organization itself. Instead of data science being an interesting addition to the mix, it must be given a prominent and central position among business strategy, IT, line management, and operations. The data science organization, if properly supported, can be untethered from the more mundane aspects of their work, and the rest of the organization can be better positioned to take full advantage of the promise of data science achievements and capabilities.
If these challenges sound familiar, surround your data scientists with the right kinds of support, and formalize roles all along the data science value chain, from ideation to discovery and development to prototyping and testing, and finally implementation and on-going support. In particular, the relationship between data science and traditional IT in all but the most progressive organizations needs to be properly thought through and formalized. Often there is mutual mistrust between data science and IT. The data scientists may think IT doesn’t understand them, their needs, or the importance of what they do. IT may view the data people as mad scientists, who have no appreciation for IT discipline, engineering, and architecture.
If this sounds familiar, I recommend the following actions:
Establish a strong data organization separate from the data scientists themselves, but with the clear mission of supporting the data science community. The data organization, staffed with data domain experts, data engineers, data architects, and data governance types, should be tasked with sourcing the required data for your data science community. They should operate at two speeds, fast prototyping, and slower (more disciplined) production engineering. So-called data wrangling tools can be used to help rapidly source, analyze, assemble, and prepare data for solution prototyping. For those ideas that the business chooses to fully develop and deploy, the same group can leverage the knowledge gained from prototyping to engineer a most robust solution with the scale, speed, and reliability appropriate for the solution.
The IT organization must be engaged to determine how best to integrate the final solution into the production environment. Upstream and downstream feeds need to be built using whatever corporate standards apply, appropriately tested, and then released into production and maintained and supported by IT with appropriate change management protocols. The data scientist community must be made to understand that this is not optional. In my experience, poorly engineered and supported solutions that are rushed into operational use end up costing the organization far more in the end than the price of doing it right.
When ad-hoc or special information requests come along, get the right people involved up front to triage the request and decide the most appropriate sourcing, and fulfillment strategy. It may not be the people who can do it the fastest and the cheapest that are the best positioned to handle the request. I’ve seen too many requests get fulfilled quickly at low cost that end up causing massive confusion because the resulting data is poorly understood, inconsistent, or worse, falls apart under close scrutiny. The best process is to create a working group of IT, data management, data science, and business domain experts to review each request and agree on the best approach, relative priority, and acceptable level of risk (i.e. 80% accurate OK, or must be 99.999%) appropriate for each case.
During testing of new data science capabilities, business leadership must be engaged and change management, business process integration, training and communication challenges, as well as realization metrics, must be discussed. Accountability for the value realized at the end of the day rests with line leaders who own their P&L. If they are not ready to be held accountable for results and to take the lead on the organizational change necessary to integrate these new capabilities into the fabric of the organization, then other deployments should be given higher priority.
Data science techniques are getting better, cheaper, and easier to use. Recommender systems, neural networks, decision trees, prediction models, are now accessible to practically anyone with some technical expertise, access to the data, and the right business case. Even small and medium sized organizations can now tap these technologies. But, if you fail to properly introduce, support, and integrate data science capabilities, a lot of money can be wasted as well.
H.P. Bunaes was most recently chief data officer for the Consumer Bank at SunTrust, the 9th largest bank in the US, headquartered in Atlanta. He was responsible for all aspects of IT investment, data management, and business intelligence for Consumer Banking, National Consumer Lending, and Private Wealth Management. Formerly, Mr. Bunaes was chief data officer for SunTrust Corporate Functions, responsible for IT investment, data management, and reporting for Corporate Risk, Finance, HR and Marketing. Prior to moving to SunTrust, Mr. Bunaes was with FleetBoston Financial for 17 years, where he ultimately led the Risk Management Information and Technology function corporate wide for both Fleet Bank (US) and BankBoston franchises in 32 countries. In addition to an advanced degree from MIT, Mr. Bunaes is a graduate of Emory University Goizueta Business School’s Advanced Leadership Program, and holds degrees in computer science and mechanical engineering from Trinity College.
About the Author
You May Also Like